Insights
- Energy transition is a top priority for CEOs in 2024 and is expected to remain a key focus.
- The energy industry faces a dual challenge to meet growing global demand while accelerating energy transition towards net-zero emissions.
- Generative AI has emerged as a transformational technology, helping companies gain a competitive advantage and to address their challenges.
- Generative AI spending across the value chain in energy, mining, and utilities would grow from $571 million in 2023 to more than $1 billion in 2024.
- Generative AI should be used to enhance core business operations to benefit the bottom line, while new revenue streams and business models should be explored.
Energy transition is a top priority for CEOs and is expected to remain a key focus of the C-suite for years. At the same time, generative AI has emerged as a transformational technology, helping companies gain a competitive advantage. Trained on past data, generative AI produces new content in text, simulations, data, computer codes, audio, and video.
The power and utilities sector is expected to lead IT spending growth, with a five-year compound annual growth rate of 11%, reaching $360.5 billion by 2028. The top potential benefits include operational efficiency, streamlined product development, and improved reliability — areas where generative AI could drive success.
Generative AI surged into prominence in 2023 with the launch of ChatGPT, prompting companies across sectors to experiment and construct exciting use cases, such as AI tools to analyze energy consumption, optimize grid load, and predict outages. These solutions offer transformative opportunities for energy, mining, and utilities companies. Generative AI is expected to advance innovations across the entire energy value chain, from oil and gas exploration to power plants, transmission, and last-mile utilities.
Generative AI is expected to advance innovations across the entire energy value chain
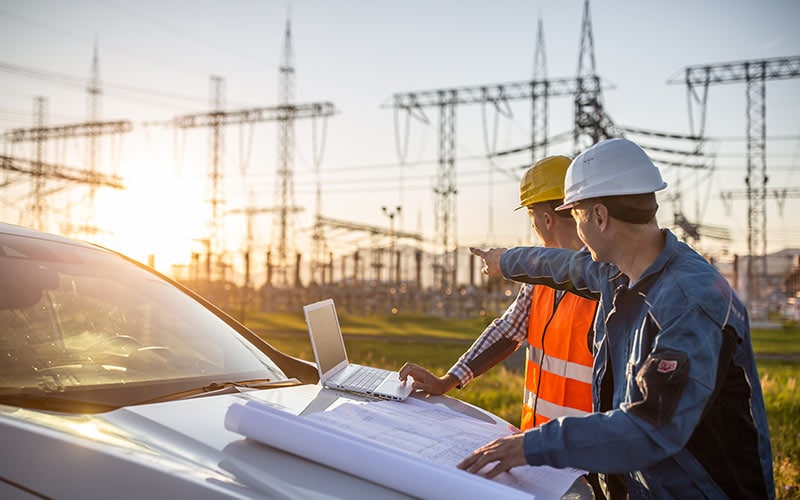
However, the rise of generative AI and associated technologies carries new risks and magnifies concerns about responsible AI, specifically data usability, privacy, and security. The International Energy Agency (IEA) reported a rapid increase in cyberattacks on electricity systems since 2018, reaching alarmingly high levels in 2022. Recent cyberattacks have disrupted energy meters due to IT system unavailability, disabled remote controls for wind turbines, and leaked critical customer information through data breaches. Globally, the average cost of a data breach in the energy sector hit a record $4.72 million in 2022.
Beyond malicious threats, AI inaccuracies during early development stages can threaten critical infrastructure operations. The US government recently set up the AI Safety Institute Consortium (AISIC) with more than 200 member organizations. AISIC’s objective is to unite AI creators and users, academics, government and industry researchers, and civil society organizations to support the development and deployment of safe and trustworthy AI.
Other risks include potential intellectual property infringement and liability from misuse. Ongoing litigations worldwide are examining whether training generative AI models on IP-protected content and the models’ outputs constitute IP infringement. In addition, the industry’s slow technology adoption could place it at a disadvantage when pursuing generative AI initiatives. While private models can mitigate many of these risks, they limit the benefits of knowledge sharing and external insights.
The value chain perspective
Companies have launched generative AI pilot programs at various levels of maturity and scale across the energy value chain (Figure 1):
- Upstream oil and gas discovery
- Midstream transportation
- Downstream refineries
- Power plant operations
- Transmission
- Utilities last mile delivery to homes, offices, and factories
For example, Shell plans to use generative AI to analyze large amounts of seismic data in its search for new oil reservoirs. GE Vernova created AI software to collect and understand operational and sustainability data, leading to more efficient and effective use of power plant resources. Exelon, the largest utility company in the US, generates synthetic data to create thousands of labeled images of various grid defects. These images are then used to train drones for inspections, improving grid maintenance, reliability, and resilience.
Schneider Electric has pointed to many generative AI use cases across the value chain, such as exploration, reservoir modeling and simulation, environmental monitoring, scenario planning and decision support, predictive maintenance, data analysis, and programmable logic controller code generation. One study reported four functions among 16 that contribute 75% of the value from generative AI. These four are customer operations, marketing and sales, software engineering, and research and development. The value from each function varies from industry to industry, based on objectives, challenges, client requirements, regulations, and technology maturity.
Figure 1. Generative AI use cases in the energy value chain
Source: Infosys Knowledge Institute
Seismic optimism
Generative AI investment in energy, mining, and utilities boomed in 2023, much like it did in other sectors. Infosys forecasted that generative AI spending in these industries would grow from $571 million in 2023 to more than $1 billion in 2024. While companies continue to test and develop use cases, evidence is building that businesses will finally get a handle on generative AI in 2024.
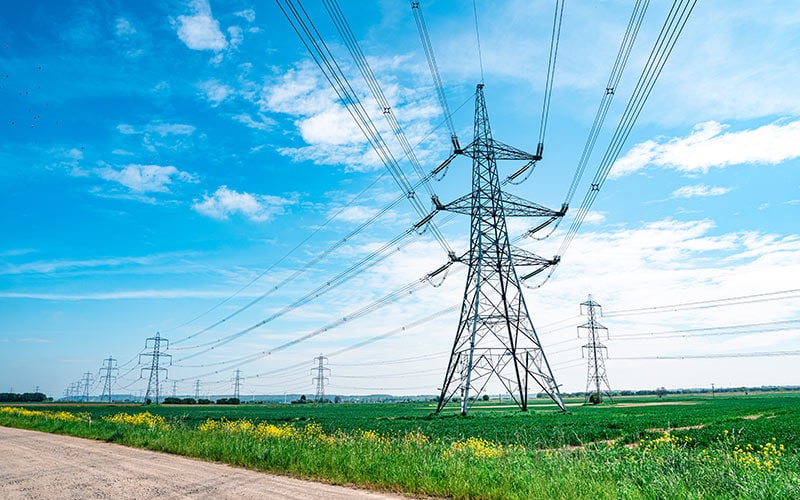
In March, Brazilian energy giant Petrobras saved $120 million in just three weeks by improving the accuracy of its tax filings with AI. Partnering with US software company Automation Anywhere, Petrobras used the Automation Copilot AI to reduce tax inaccuracies and better comply with regulations.
However, using AI in sensitive areas like tax filings requires caution. AI can misinterpret tax laws, especially when parsing highly technical and precise language from different countries. AI is also prone to hallucinations, meaning the mistakes go beyond just missing nuance and making up outright falsehoods. Companies need strong governance and risk management practices, such as human-in-the-loop systems, to responsibly use AI.
The Petrobras example illustrates the transformative potential that generative AI offers, often at mind-boggling speed. Energy, mining, and utilities have opportunities to innovate every aspect of their value chain, from exploration to extraction to transmission. And this has already started. Nearly half of energy, mining, and utilities executives say they have already implemented or are currently implementing generative AI solutions — only 17% yet to start (Figure 2).
Figure 2. Generative AI adoption in energy, mining, and utilities firms outpaces overall trend
Source: Infosys Knowledge Institute (N=259)
These real-world examples show that generative AI is more than a passing trend. It presents the opportunity to drive innovation, reduce costs, and uncover new opportunities across the entire energy value chain. However, energy, mining, and utilities must overcome significant obstacles to fully realize this potential.
Obstacles to generative AI adoption
Availability of clean, complete data in a usable state without noise is a major obstacle for AI adoption in the energy sector (Figure 3). Concerns about ethics, bias, fairness, and safety in the energy industry are less than half that of the overall sample.
Successful deployment of emerging trends needs top-down support, starting from the boardroom and CXOs, trickling down to middle management, and finally to the rest of the organization. Generative AI is no exception, and the oil and gas industry is at this stage. The lack of C-suite buy-in and investment are obstacles for more than twice as many study participants in the energy industry, compared to the overall study results.
Figure 3. C-suite buy-in and lack of investment are bigger obstacles to AI adoption
Source: Infosys Knowledge Institute
Workforce generative AI readiness
A recent study of the oil and gas industry underscores the importance of human resources initiatives such as reskilling, cultivating a culture of continuous learning, aligning learning initiatives with business objectives, and leveraging learning platforms to prepare the workforce for digital transformation. Among the technologies, big data and analytics are expected to experience the highest adoption rates, followed by the internet of things, cloud computing, and wearable electronics. AI and generative AI are poised to extend the capabilities of big data and analytics.
Our study shows a slightly more positive mindset about the adoption of generative AI (Figure 4) in the energy sector. One reason is generative AI’s potential to enhance safety in high-risk environments such as oil rigs, refineries, and power plants.
Figure 4. Executives are optimistic about workforce readiness to adopt generative AI
Source: Infosys Knowledge Institute
Research shows that 34% of the oil and gas workforce requires reskilling to fill emerging AI-driven roles, a challenge given the competition for tech talent across industries. A pre-pandemic study found five broad competencies required for AI readiness: leadership, foundational or basics, behavior, knowledge, and technical. Among these, technical competencies have the most potential for automation, which has led companies to focus their reskilling efforts in this area due to its predictability and feasibility.
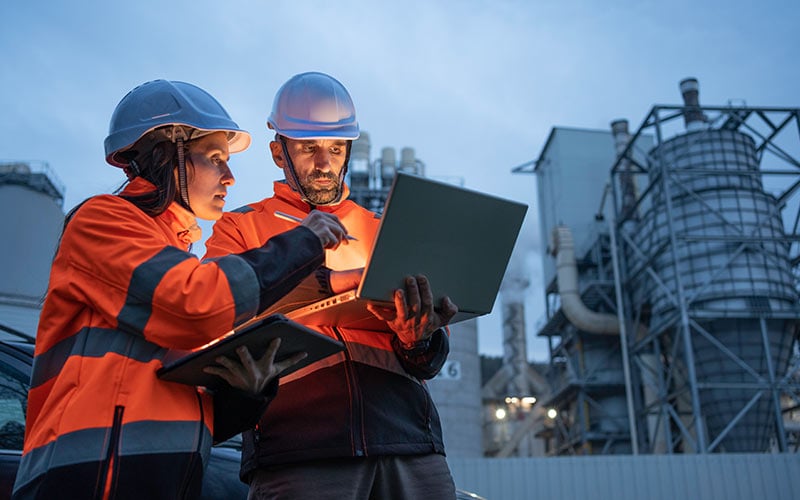
Generative AI policymakers
The number of CIOs acting as decision makers suggests that there is still a siloed approach to generative AI adoption (Figure 5). The most successful companies have adopted a cross-functional approach, cutting across the enterprise.
AI’s biggest impact is felt when it is developed collaboratively by cross-functional teams with diverse skills and perspectives. When business stakeholders work with IT teams, the result is not just a technical solution but a resolution for a business challenge or opportunity. Shell considers technology the least important element for digital transformation. Success is driven 60% by data, 30% by people, and 10% by technology.
Figure 5. Nearly half of energy companies say CIOs are the primary policymakers, almost double the overall trend
Source: Infosys Knowledge Institute
Case for generative AI in energy, mining, and utilities
EPRI has identified safety and reliability as two unique factors driving generative AI adoption in the energy industry, beyond the usual focus on revenue and profit. There can be no compromise on these factors when adopting any emerging technology. While these are the reasons for the delay in adopting AI when compared to other industries, they are the same reasons for impactful applications of generative AI today. The technology is mature enough to be implemented for critical applications.
At the same time, generative AI should be used in responsible ways. Data centers that host generative AI are already major consumers of electricity and sources of carbon emissions. Energy usage for running data centers is set to double, from 4% of electricity production in the US today to more than 9% by 2030. Energy firms should decide on meaningful use cases for generative AI and be efficient in implementing and using them.
Practices to manage the generative AI duality
Energy, mining, and utilities firms must balance the needs of experimentation and risk mitigation to extract the most value possible from generative AI.
The energy sector must balance the needs of innovation and risk mitigation to extract most value from generative AI.
They must understand the potential impacts on operations and establish comprehensive data and AI governance. An AI-first approach can streamline approvals for key decisions, future investments, and new initiatives.
Here are key practices to manage the duality of balancing innovation and risk mitigation:
- Maximize core and emerging value. Use generative AI to enhance core business operations —improving efficiency, yield, safety, and compliance — all benefits to the bottom line. Explore new revenue streams and business models, such as subscription-based products and services, faster time-to-market for new offerings, and development of new markets.
- Integrate AI across IT investments. Incorporate generative AI into IT investments to automate tasks and optimize decision-making. This will widen the reach of AI across the organization and amplify its impact throughout all disciplines in IT.
- Enhance skill development. Ensure investments in skill development translate into practical value. Create incentives for employees to actively use these learning initiatives and ensure these initiatives are linked to business goals.
- Progress beyond obvious. Move from generic use cases (query resolution with large language models and chatbots for example) to more impactful AI applications that drive revenue, improve profit margins, and enhance safety and reliability. Identify and implement industry-specific use cases that address business and client needs, challenges, local regulations, and maturity of technologies.
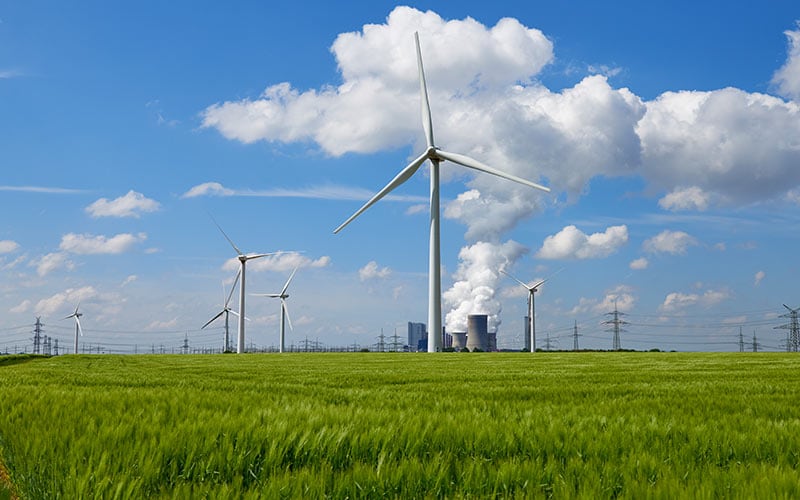