Insights
- By flipping the inputs and outputs of the previous generation of AI models, generative AI is creating vast opportunities. Simple inputs now can yield more complex outputs, often with breathtaking speed.
- The training of the largest of the large language models, which power generative AI, can emit dozens to hundreds of tons of carbon. At the same time, a generative AI enhanced search might consume 100 times more energy than a traditional search on Google, according to one estimate.
- The rush to adopt AI is clashing with another corporate priority: decarbonization. Virtually all large companies have made public commitments to significantly reduce, or even eliminate, their carbon footprint. Nearly half of companies on the Forbes 2000 list have set net-zero targets.
- The electricity sources and energy efficiency of data centers are critical to AI sustainability, in addition to information technology systems overall. Data centers and data transmission networks use about 2-3% of the world’s electricity.
- Hardware innovations are critical to AI sustainability but must also be paired with strategic decisions about AI models. Open models and the use of quantization, pruning, and knowledge distillation can reduce the size models and computing power or storage needed.
From the toys of the 1990s to the online recommendation engines of today, artificial intelligence (AI) has steadily migrated from the laboratory to the marketplace. IBM’s Global AI Adoption Index found that 42% of enterprise-scale businesses have already deployed AI, and another 40% are either exploring or experimenting with the technology.
This strong but steady adoption of AI was thrown into overdrive with the release of ChatGPT, which convinced many executives that AI is not just a promising solution but an urgent business necessity and potentially a transformative one. IBM’s research found that 59% of companies that haven’t deployed AI are now accelerating their investment or rollout.
However, this AI gold rush is clashing with another corporate priority: decarbonization. Virtually all large companies have made public commitments to significantly reduce, or even eliminate, their carbon footprint. Nearly half of companies on the Forbes 2000 list have set net-zero targets, according to Net Zero Tracker.
Business executives have known for a while that emerging technologies might present a challenge to their environmental goals. Now, the clash is happening faster and more significantly than most would have expected. The impact is felt in two areas:
- Training (usually one-time event).
- Inference or prediction (ongoing).
The training of the largest of the large language models (LLMs), which power generative AI, can emit hundreds of tons of carbon — although Google researchers say many of the eye-watering numbers are much too high. At the same time, a generative AI enhanced search might consume 100 times more energy than a traditional search on Google, according to one estimate.
While LLMs offer rapidly growing benefits, their transformative nature also makes them an environmental risk.
AI getting more powerful — and power hungry
The previous generation of AI models are proficient at identifying and classifying objects, both online and offline. The algorithms amazed users by identifying significant elements in an input — including words, sounds, or pictures — and then almost instantly turning them into labels that can then launch additional actions. For example, the app Vivino uses AI to identify a specific bottle of wine in a photo and then match it to crowd sourced ratings. And automated transcriptions, as software features or in standalone apps, are now common. This was enough to create an AI market worth more than $140 billion in 2022.
Generative AI arrived with an obvious and enticing appeal for the C-suite, who are already seeking ways to incorporate AI into their businesses. LLMs promise efficiency, innovation, and optimization — all while making these tools more user friendly. It was the public, rather than corporations, that demonstrated the first capabilities of ChatGPT and created much of the buzz. This ease of use accelerated the adoption of generative AI, even among risk-averse large companies.
By flipping the inputs and outputs of the previous generation of AI models, generative AI is creating vast opportunities. Simple inputs now can yield more complex outputs, often with breathtaking speed.
Figure 1. Generative AI has reversed the inputs and outputs in the models
Source: Infosys Knowledge Institute
Rather than identifying and classifying objects, foundation models can create something new, whether it’s video, 3D models, audio, or computer code. These models can transform a written prompt into a realistic simulation of photos or audio of a human-sounding voice, with specific accents and intonations. The newest wave of AI, powered by foundation models, is enabling new capabilities that were not easily possible previously.
Source: Infosys
The most significant difference between the past and present generations of AI is that they require different models. Previous versions were more compact since their jobs were narrowly focused on individual tasks, including translation, parsing, sentiment classification, and answering simple questions that fall within a predictable range. Today’s foundation models bring all those capabilities — and more — into a single model that is trained on large, unlabeled datasets. Multimodal AI also increases the computational demands by training with different types of datasets, including video, audio, and text.
As a result, the energy intensity is only accelerating as model inputs increase exponentially. The original ChatGPT was trained on 117 million parameters. By version three, the model had increased to 175 billion. And a new Chinese LLM, Wu Dao, relied on 1.75 trillion parameters.
The training of foundation models can take 100 days or more — sometimes using tens of thousandsof graphics processing units (GPUs). As a result, these models use a great deal of electricity when training and create tons of greenhouse gases, depending on how their data centers are powered.
How to make AI more sustainable
The first wave of generative AI fever has focused on finding as many use cases as possible, and then scaling them up as quickly as possible. Soon, the C-suite will expect its technology leaders to continue this accelerated pace of AI adoption, only now in a more sustainable manner. The feared business phrase might be on its way: Do more (AI) with less (emissions).
There is not a single or simple path forward. Businesses will need to align their sustainability goals with their technology capabilities. The challenges are varied depending on whether their companies are concerned only with scope 1 and 2 emissions, or also with the significantly more challenging scope 3 emissions (generated by a company’s value chain, including suppliers and customers).
Companies’ ability to lower their emissions will vary based on several factors, some of which they have more control over than others.
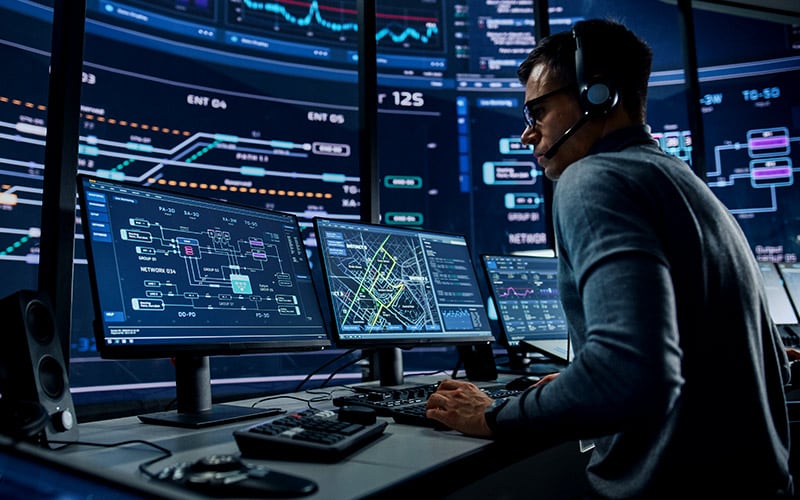
Data centers
The electricity sources and energy efficiency of data centers are critical to AI sustainability, in addition to information technology systems overall. Data centers and data transmission networks use about 2-3% of the world’s electricity.
The most obvious sustainability solution is to power data centers only with renewable energy — a growing priority for hyperscalers. Despite the interest, there are limitations in some regions based on the cost and availability of renewables. Renewable capacity is growing rapidly, but these sources still make up about one-third of electricity production worldwide.
No matter the electricity source, companies are closely scrutinizing their data center’s efficiency through metrics such as power usage effectiveness (PUE) and carbon usage effectiveness (CUE). Greater efficiency offers cost benefits, sustainability benefits, or both.
- PUE calculates the power used by the IT equipment compared to the total power transmitted to the data center. A number close to 1 is better, meaning less of the electricity is wasted. PUEs for the world’s largest data centers averaged 2.5 in 2007 but have dropped to slightly more than 1.5. That average has been relatively flat for the past several years, however, many companies are seeking greater efficiency. The Lefdal Mine Datacenter was constructed in a former mine to enhance cooling and in a section of Norway with 100% renewable energy. The data center’s PUE of 1.10 to 1.15 makes it particularly attractive to companies, such as Daimler, that want to reduce their carbon footprint.
- CUE drills down further, taking into account the energy sources used. This number calculates the carbon emissions created in proportion to the IT energy usage. A data center that uses only renewable energy would score a perfect zero.
These metrics have allowed data centers to quantify the progress they are making. Energy-efficient hardware and cooling systems, virtualization, and workload scheduling have ensured that the exponential growth of data and internet traffic did not lead to an exponential increase in electricity usage. The International Energy Agency (IEA) calculated that data center workloads increased by 340% between 2015 and 2022, while data center energy use increased from 20% to 70%.
Data centers might struggle in the near future to continue this trend. The IEA projects that data center electricity consumption could double by 2026, thanks in part to AI and cryptocurrency.
Google executives addressed some of these issues in the 2023 Hot Chips semiconductor industry conference in Silicon Valley. Amin Vahdat, vice president of engineering at Google, explained that the computing demand for dense parameter AI models has increased 10-fold annually in recent years. He laid out a sustainability path that requires increasingly sophisticated steps, including new AI-specific chips, specialized data representation, optimized power parameters for every job, and other data center advances. His colleague, Jeff Dean, chief scientist for Google DeepMind and Google Research, highlighted the possibility of AI’s use to design more efficient chips to run the next generation of AI models.
“The kind of computing infrastructure that we have to build to meet this challenge has to change,” Vahdat said at the conference.
Models
Hardware innovations are critical to AI sustainability but must also be paired with strategic decisions about what models to use and how to use them.
Open vs. closed models — Generally, businesses have adopted closed models, such as ChatGPT, since implementation is faster and initial costs are lower. However, open models offer specialized capabilities and reduce the amount of data required. This more compact training data creates outputs that can be tailored to a company or industry, and also reduces the processing and data storage needs — resulting in a smaller carbon footprint.
Students in the US and Abu Dhabi created the open-source Vicuna chatbot as a more affordable and more environmentally sustainable option than the best known LLMs. Vicuna cost $300 to train and produced a fraction of the carbon footprint of ChatGPT. At the same time, it has scored close to ChatGPT and Google’s Bard on subjective language assessments.
Model and algorithm efficiency — Engineers can reduce the complexity of AI in several ways but still get the complex outputs and accuracy needed. In some cases, companies can use “tiny AI” models that run on edge networks rather than cloud servers — reducing the electricity needed for computing and for data transmission.
- Quantization reduces the size of an AI model’s parameters so that it requires less computing power and minimizes data transfer. This can also help AI run on smaller, less powerful edge devices and make inference faster.
- Pruning includes a variety of techniques to reduce the number of parameters after a model has been trained. This doesn’t reduce the environmental impact of training but does lower the environmental impact of storage and use.
- Knowledge distillation takes the knowledge from a large model and “distills” it down to a small model that requires less energy for inference.
Pre-trained models — By using pre-trained models as a starting point, companies can build on that foundation, rather than starting from the ground up. Fewer computational resources are needed, which lessens the environmental impact. Also, this approach can accelerate training since the basics won’t need to be duplicated.
Driving AI’s future
Financially, there is no doubt that AI will fuel a technology spending boom for the foreseeable future. Goldman Sachs projects that AI investment will reach $200 billion globally by 2025 and continue upward rapidly. By 2032, the generative AI market could reach $1.3 trillion, according to a Bloomberg Intelligence report.
The business impact of AI is still up in the air for many, particularly for the newer and more hyped generative AI. In Infosys’s Generative AI Radar report, we found that most companies were at least experimenting with generative AI and the rest had plans for the technology (with few exceptions). But a relatively small percentage had actually created business value from generative AI: 16% in North America, 13% in the Asia-Pacific region, and 6% in Europe. Even with that slow start, executives were very optimistic about the business promise of generative AI.
The environmental impact of AI, however, has a higher degree of uncertainty. For business executives and data scientists, AI creates a complicated race running along parallel — but very different — tracks. The growth of AI in business, government, education, and among consumers is speeding along at an ever-accelerating pace. Innovations from computer scientists and engineers, and growing access to renewable electricity, have been able to slow AI’s impact on greenhouse gas emissions — for the moment.
Technology and business experts generally see AI’s future as a positive one, offering transformational opportunities that we can’t yet comprehend. While true, uncertainty lingers over many elements of AI, whether it’s bias or security risks, workplace displacement, or the technology’s contribution to climate change. AI’s future will continue to be bright only if organizations take a step back to clearly understand the advantages and disadvantages of AI and think strategically about how to maximize the former and minimize the latter.