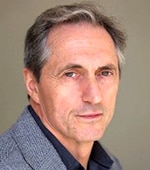
Prof. Valery Yakubovich, executive director at the Mack Institute for Innovation Management, The Wharton School, is focused on fostering synergy between research, teaching, and the practice of innovation management. He emphasizes dialogue between academia and corporations, while strengthening connections with regional and corporate partners' innovation ecosystems.
In a fascinating interaction, Prof. Valery Yakubovich shared his views on several aspects of generative AI.
The Mack Institute was setup as a research center for technology-driven innovation in 2000 with alumnus William Mack’s initial contribution which he turned into the institute’s endowment in 2014. The institute’s focus is on funding and supporting Wharton faculty’s research on innovation and entrepreneurship and translating its output into experiential learning and business practice. While large and medium-sized companies are the institute’s traditional constituency, the more recent faculty research prompted deeper engagements with startups and innovation ecosystems locally and globally. Commercialization of academic science, corporate venturing and interactions between established companies and startups are major areas of the institute’s current research and practice.
Generative AI: What and how?
The Mack Institute has always focused on technology-driven innovation. When ChatGPT burst into fame in November 2022, companies were worried: will jobs vanish, will we miss the bus, what should we do about it? In this regard, generative AI, like other disruptive technologies, is likely to follow a typical pattern of initial hype, followed by a 'bubble and burst' period before its true potential emerges. What sets it apart is its rapid adoption, driven by its accessibility for users across various industries.
Unfortunately, there has been a knee-jerk reaction from corporates who rush to capture value before it is created. Corporates should try out generative AI, explore, experiment with it, and not rush the adoption journey. The focus should be on improving productivity, not cost reduction. The investment in this technology is staggering. But there are not many proven, successful business models yet for returns from generative AI. It is hard to account for all cost elements that go into adopting it – infrastructure, data preparation, model customization, validation, deployment, and skill development. It is important to define the economic value from AI investments, how to unlock it first and capture second.
“Corporates should try out generative AI, explore, experiment with it, and not rush the adoption journey.“
Machine learning (ML) has existed for over a decade, yet its value remains elusive in many fields. For example, when an ML-based x-ray diagnosis contradicts a doctor's assessment, the inability to explain the algorithm's decision due to the 'black box' nature of many models presents a significant challenge. However, as large language models (LLMs) improve their reasoning, new solutions for addressing explainability will emerge. Yet, as the introduction of the OpenAI o1 model shows, vendors are likely to hide their models’ reasoning chains to protect their competitive advantage. The business community should hold vendors accountable for such behavior.
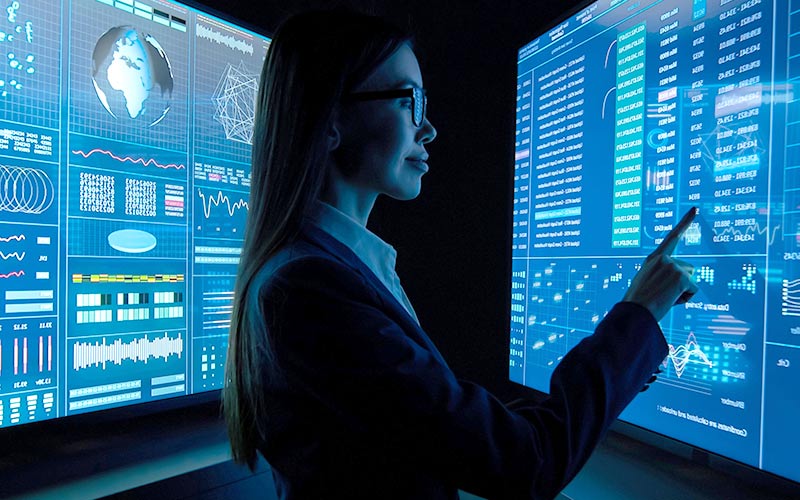
Automation vs. generative AI
The ongoing debate between automation and augmentation in the context of generative AI overlooks a critical distinction in this technology. Automation relies on well-defined inputs, processes, and outputs that can be consistently controlled, allowing users to trust the system to deliver reliable results. Generative AI operates differently: inputs are highly variable, outputs are unpredictable—even with the same input—and the entire process remains a 'black box.' This unpredictability raises concerns about the potential loss of human control, as evidenced by numerous examples already. This is why Prof. Valery Yakubovich and his colleagues emphasized in a recent WSJ article that , regardless of the role AI assumes, the need for human oversight and thorough vetting will always remain essential.
As the amount of data used and output produced grow exponentially, the number of humans in the loop should also grow, albeit at a much lower rate which will ensure productivity gains. Humans will not only deal with hallucinations, non-standard situations, and conflicts, but will also bring in tacit knowledge. That knowledge is either not encoded at all or is encoded in unstandardized records, such as emails. So far, organizations have been very hesitant to entrust such records to generative AI for the reasons of quality, privacy, and confidentiality.
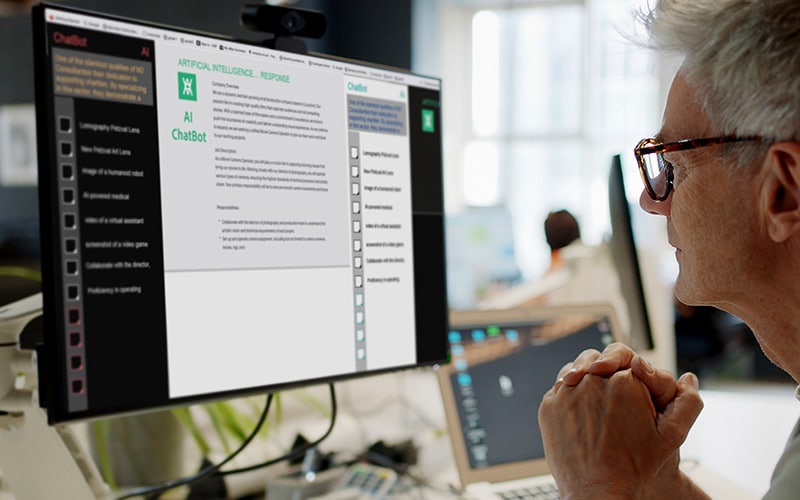
Use cases and the future of work
There are two broad use cases where generative AI can deliver value: customer relationships and knowledge management. However, realizing AI’s potential requires a lot of trial and error. In one recent example, a company automated the manual task of data extraction from semi-structured documents. A customized LLM achieved a more than 90% accuracy but turned out to be very expensive to use on tens of thousands of documents. As an alternative, the company implemented OCR (optical character recognition) first, flagging records where the input was incomplete or not clear. In this case, only 3% of the documents had to be sent to AI. As a result, the cost came down enough to achieve higher efficiency vis-à-vis the manual approach.
This example points to one path towards automation: an LLM is adapted for a specific task whose input and outputs are well-defined and can be controlled. In other words, generative AI, as a general-purpose technology, gets implemented in a specific productive tool. Software vendors are likely to design plenty of such tools and thus facilitate their adoption by businesses.
Alternatively, businesses can use an LLM to train a bot to control the input and output and engage humans only if something goes wrong. Such an implementation of LLM as an AI agent rather than a tool requires not only technological advances but major organizational and cultural shifts whose contours we are only beginning to comprehend.
Hallucination for innovation
Hallucination is a handicap for productivity but a benefit for innovation. When designing a new product for example, crazy ideas and thus large variance in the output of generative AI are welcome. Although it means that many exceptionally bad ideas are generated, we need just one exceptionally good idea to make the process worth the effort, of course, if the process can efficiently separate the wheat from the chaff. This is an active area of the Mack Institute’s research and an exciting opportunity for collaboration with Infosys.
“Hallucination is a handicap for productivity but a benefit for innovation.“