Knowledge Institute Podcasts
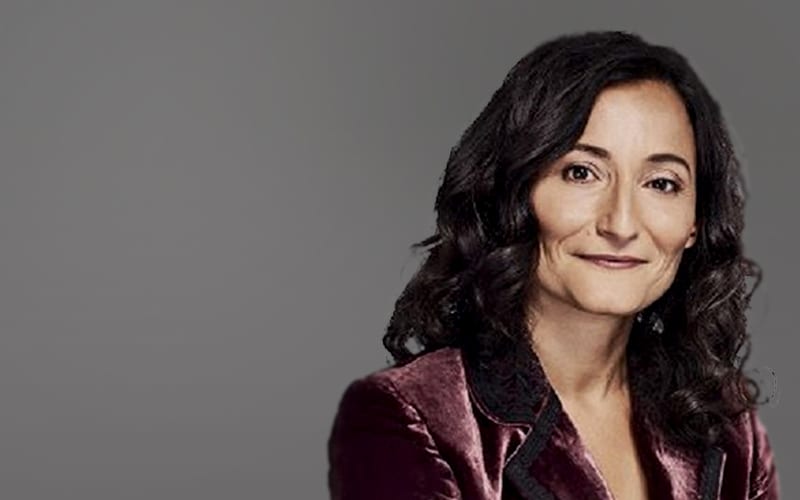
-
AI Interrogator: Trustworthy AI and Ethics with IBM Consulting's Phaedra Boinodiris
April 8, 2024
Insights
- There is a need for a comprehensive approach to AI development, recognizing it as a sociotechnical challenge that requires collaboration across diverse disciplines. By incorporating perspectives from anthropology, sociology, psychology, law, and other fields, AI projects can better address ethical considerations, societal implications, and biases throughout the AI lifecycle.
- Engaging young people in discussions about AI ethics and governance is crucial. By integrating AI literacy into education curricula, particularly in civics classes, students can develop critical thinking skills and awareness of algorithmic bias from an early age. Empowering youth to ask tough questions about AI and understand its societal impacts is crucial for shaping a responsible AI future.

Kate Bevan: Hello, and welcome to Infosys's being AI- first podcast, the AI Interrogator. I'm Kate Bevan of the Infosys Knowledge Institute, and my guest today is Phaedra Boinodiris. Phaedra is hugely distinguished. Her day job is as IBM's Consult-ing's global lead for Trustworthy AI. And on top of that, she's the author of the book, "AI for the Rest of Us," and she's also the co-founder of Future World Alliance, which is a nonprofit that works with young people, and we'll come back to that. Also, you are working towards a PhD in AI and ethics, is that right?

Phaedra Boinodiris: Correct. Correct. Yes.

Kate Bevan: And also, you've won United Nations Women of Influence in STEM and inclusivity award, so I'm absolutely delighted to have you here.

Phaedra Boinodiris: The pleasure is absolutely mine.

Kate Bevan: Let's start off by asking you what brought you to AI, and how did you come into doing it as both your day job and in the wider part of your life?

Phaedra Boinodiris: I'd been fascinated by artificial intelligence early on in my career, since the get-go, just because it's such an incredibly useful and powerful tool that can truly be used in all kinds of ways, whether it's to solve complex problems, or to really just bring delight. So, I noticed several years ago that there were examples in the news of organizations that truly had the best of intentions with respect how to use artificial intelligence. But then, because of one reason or another, they ended up causing unintended either individual or societal harm. So, I wanted to learn as much as I possibly could about the space of AI ethics and AI governance. And the more that I learned about it, the more I rec-ognized that it wasn't... Earning trust in something like artificial intelligence, it wasn't strictly a technical problem at all, but something that was truly sociotechnical. With this revelation, and thinking through, "What does it actually take for an organization to earn people's trust?" I worked with leadership at IBM in order to be able to establish a set of services through IBM Consulting to help our clients holistically with this space.

Kate Bevan: Okay. So, what do we need to do to get the outcomes we want from AI?

Phaedra Boinodiris: Since we know it's a sociotechnical challenge, what you need to do anytime you hear the word sociotechnical means you have to approach it holistically. Diving into making sure that indeed you're approaching the space with an open growth mindset, that you approach it with humility, that you really consider the teams of who is developing AI to-day, and recognize that there is this teeny, tiny, homogeneous group of people determining what data sets to use to train our models. So having those teams truly be diverse and inclusive, not just in terms of gender, race, ethnicity, neurodiversity, et cetera, et cetera, but also in truth, different lived world experiences. And then, making sure that those teams are multidisciplinary in nature. And this is on top of making sure that you're considering things like, "What is the relationship that your organization wants to have with artificial intelligence, right?

Kate Bevan: What do you mean by, "Relationship with AI?"

Phaedra Boinodiris: As an example, when we asked ourselves this question, we at IBM, the three guiding principles of our relationship started off with the purpose. What is the purpose? What's the purpose of AI? The purpose of AI is not meant to dis-place human beings. It is not meant to have control over human beings. It is meant to augment human intelligence. But what that means in practice, is you actually have to have people trained to be thinking about who is being given power with AI. Are they empowered? What is the experience like of a person if they've been augmented by AI, right?

Kate Bevan: There's a difference, isn't there? Between being empowered, and being given power over AI?

Phaedra Boinodiris: Being given power with AI... How are you being given power with AI? And then, what is that experience like? It means that we actually had to staff up, or stand up, an entire AI design guild thinking through that human-centric experience. But then, thinking about also the data and insights associated with those data belong to their creator. That's a statement about consent, that all AI systems should be transparent, then explainable. And then, that's how we characterized our three principles around the relationship we want to have with AI. But then, there's these hu-man centric questions that need to be answered.
The big challenge of why this is a sociotechnical challenge, is because what we're trying to ultimately do is have our very human-centric values be reflected in our technologies. We expect AI to not lie to us, to not discriminate, to be safe for us and for our children to use, which means we've got these human-centric questions to ask. "Is it fair?" We can all agree. Of course, we expect AI models to be fair, but what I think is fair might not be what you think is fair. So, thinking about, "What are all the different worldviews on the subject of fairness, and how do we expect that worldview to show up in our models?" Because, for example, there's equality, there's equity, there's meritocracy, there's all these different world views. How do we expect to measure that in an AI model?

Kate Bevan: Do you think we're succeeding in holding it to account at the moment? I mean, I'm looking at some of the things that are coming out of Google's new model, which has been producing... I don't know if you saw this over social media over the last week, but Google's model. There was a backlash because somebody asked it to produce images of a pope, and it produced a black pope. Now, some of us might think, "That's fantastic. That's progress." But others were going, "This is terrible. All popes have been white." So, are we succeeding in holding it for account and getting it to behave in ways that we want it to behave?

Phaedra Boinodiris: That is an interesting example. So, one of the questions that I regularly ask organizations who are developing AI and putting it onto the world is, "Who in your organization, which business persona, is accountable for equitable out-comes from AI?" And when I ask this question, I typically get three answers. There are three dominant answers, and each of these three are actually concerning in their own right. The first answer is, "We don't use AI," which, baloney. Absolutely there are people in your organization using AI in some way, shape, or form. The second answer is, "No one," which, of course, is obviously concerning for its own reason. And then the third one, which is also concerning, is, "Everyone." And the reason why this is concerning, is because you actually need to have groups of individuals who have enough power and a funded mandate to do the work of AI governance, because they have to have enough power to do things like shut down projects, or to audit AI models. Not everybody has that power. Who in your organization has that mindset, and has enough power with funding to go and do that kind of work?

Kate Bevan: So where do you think ownership and governance of AI best sits? Because at Infosys, at the IKI, we've done quite a lot of research into how businesses are rolling out generative AI. We've looked at it across North America, across Europe, and across APAC, and we've seen a very wide range of who has ownership, who has governance of these. Sometimes it's the CIO, sometimes it's the CTO, sometimes it's the IT teams, sometimes it's not even the C-suite. Have we got a handle on that; do you think?

Phaedra Boinodiris: Well, let me rephrase. There are best practices that have been described through a number of different reports. There are some concerns that have been relayed about having someone like a CTO also be responsible for AI gov-ernance. And the phrase is, "The fox watching the henhouse," meaning, are they actually incentivized to do the work of AI governance? Then I think it's important to recognize that the work of AI governance has to be actually multi-stakeholder and multidisciplinary. Which means you're going to need people who not only understand AI models and the operationalization of what happens the AI life cycle, but also people with backgrounds in law, peo-ple with backgrounds in comms. I mean, because, again, you've got to have all these different kinds of people work-ing together.
What I'm finding interesting is that often, we're seeing more and more, not just chief compliance officers, chief pri-vacy officers, but also CISOs being told that they're now accountable. And what's interesting about seeing CISOs with this role, is one could opine that they do have the right mindset, because they've already been doing the work around data privacy to make sure they're compliant with regulations like GDPR and cybersecurity. That they may have the right mindset to ensure that models are behaving in the way that we ultimately want them to behave. Now, it is a stretch, because now they're having to go into things like making sure those models aren't discriminatory or biased, which had not historically been in their purview. So, we'll see. We'll see.

Kate Bevan: Yeah. I mean, it's interesting that you make that comparison, because it's often occurred to me that a lot of the thinking we do around cybersecurity, secure by design, treating each individual as an endpoint, very much applies to how we work with AI governance, ethics around that. At this point, I want to ask you about your work with young people, because young people have got to be involved as stakeholders, right? How are you getting young people involved?

Phaedra Boinodiris: Oh, goodness. As I've been doing this work, it is becoming more and more apparent to me that the way we've been teaching artificial intelligence in formal learning settings has really been hurtful in some ways. And it is because the way that we have communicated what's in these classes, and where we have put these classes, for example, in schools of engineering, we've been messaging to the world that these classes are for those who self-categorize in a certain way. They self-categorize as future computer scientists, future machine learning scientists, future data scien-tists, but literally not everybody else who actually needs to have a seat at the table when it comes to responsibly curating AI.
I love the definition of the word "data," that data is an artifact of the human experience. We humans, we make the data, the machines that make the data, but we have over 188 biases and counting. So, we need people trained to be thinking about things like, "Is the data that we're choosing to train these models, is it representative of all these di-verse communities that we need to serve? Is it gathered with consent? Is it even the right data to be using according to a domain expert to solve the problem?"
And so often, when thinking about things like, "Is it empowering a human being?" and "Is it representative?" I mean, so many of these things stretch well beyond the tech. We desperately need to have people who have back-grounds in anthropology, sociology, psychology, behavioral design, legal backgrounds, et cetera, working together in order to be able to curate AI in a responsible fashion. So, I've been really advocating that instead of just having artifi-cial intelligence being taught in computer science classes, that it's actually taught in civics classes. So, when I engage with our youth, what we do is we use games and play to introduce the subjects of things like algorithmic bias. And it's been wonderful. It's been so enlightening to see the reaction from children.

Kate Bevan: Just remind me how old the kids are that you work with.

Phaedra Boinodiris: Oh, I've worked with mainly middle school and high school kids. Yeah. And for example, last spring, we had a big summit at the Duke University with 400 Girl Scout troops.

Kate Bevan: Oh, wow.

Phaedra Boinodiris: And a dear friend of mine from Microsoft, she, and I, we gave a talk. And not just about social media, but also on the subject of algorithmic bias. And we asked these girls. We said, "Well, give us examples of where AI has absolutely delighted you. Give us examples where you were playing around with an AI, but you really didn't like the output. You didn't think it was fair. Or give us examples of where this has happened to you." And Kate, just listening to these girls, I mean, was wonderful, because you could see they really got it.
As an example, one girl was saying how she was playing around with an image generator where you upload your photo and it will redraw you in different ways, right? And she was saying, "When my brothers or my dad upload their photos, it redraws them as cowboys, and presidents, and astronauts. But when me, or my sisters, or my mother up-load our photos, it redraws us in various states of undress."

Kate Bevan: Oh, wow.

Phaedra Boinodiris: Yes. And it approached the conversation about training data. Right? And then another girl, she was from Nepal, and she was there with her family. And she was using an image generator asking it to generate a painting of two children in her mountain village of Nepal. And she said, "Look what it drew. Look what it drew." It got the mountains beauti-fully, and then drew two blonde-haired blue-eyed children.

Kate Bevan: Oh, no.

Phaedra Boinodiris: And she said, "This doesn't look like my people." And again, I broach the conversation about training data. Since then, there's just been wonderful, wonderful examples where generative AI has really been able to, I think because it's so easily accessible, open people's eyes about things like training data and the algorithms that are behind these. Like the London Interdisciplinary School came out with this wonderful video, showing where they had a number of statisticians asking one of these image generators, "Show me a photo of a typical doctor. Show me a photo of a typi-cal CEO. Show me a picture of a typical prisoner, caregiver, terrorist." And these statisticians, what they were doing was they were measuring which gender, which race, what ethnicity, what skin color is being represented in these images. And mind you, there's no test-retest reliability with generative AI. So, you can ask it the same prompt a thousand times to get a thousand different photos, right? So, what they were able to demonstrate were examples of representational harm, as I'm sure you can imagine. "Show me a picture of a typical terrorist." Guess what image is being shown again and again?

Kate Bevan: You can just imagine, can't you? What it has shown?

Phaedra Boinodiris: Yeah. Yeah. They were able to demonstrate just the level of representational harms. And they went further, which I thought was delightful. I'm really a big fan of what these guys have done. They asked this image generator, "Show me an image of a Barbie doll that represents each country around the world." And it showed... All the South Ameri-can Barbies had fair skin and blonde hair.

Kate Bevan: Ugh.

Phaedra Boinodiris: The one from Germany was wearing an outfit reminiscent of an SS Nazi uniform.

Kate Bevan: Oh my God.

Phaedra Boinodiris: Several of the Barbies from African countries are literally holding machine guns. And again, it goes back to who is de-ciding what training data to use behind these models. And that is ultimately why we need far more diversity and in-clusivity than we have today.

Kate Bevan: Are you seeing a different way of thinking from the young people, that can feed into how we treat this in the fu-ture?

Phaedra Boinodiris: Well, I think what we need to do is make room at the table in the conversation with AI. We need to be really explicit about who belongs in this conversation. Which means we need to be very careful about how we communicate on the subject of artificial intelligence. We need to do this yesterday. And what we need to do is get people into the habit of asking tough questions about AI, like, "Who's accountable for this model?" Right? Or "Where can I learn more about what training data was used in order to come up with this output? Where did this output come from? How much better does this AI model perform compared to a human being? When was this audited? How often is it audited? What was it audited for? Let me look at this. Is this interpretable? What does this mean in terms of a gen-der bias audit or age bias audit?" We have to build up our muscle memory, not just for us, but for any consumers of artificial intelligence to get used to asking these kinds of questions.

Kate Bevan: So I'm going to finish up, because this brings me to my finishing question that I ask everybody. And it's about your optimism, but it's also about fear. Do you think the AI is going to kill us all?

Phaedra Boinodiris: Oh, I think, earnestly, that is a red herring. Earnestly, I do. And I think it's much more obvious what the risks are, much, much more obvious. Earnestly, it's not going to be a Skynet or a Terminator. It is going to be simply our own fallibility, as we just described. As we just described, which is why it's so important to impress upon people the im-portance of AI literacy in a holistic fashion, so that people understand, because they don't. People do not under-stand how the sausage is made. Black boxes aren't enough. That is not okay. We should be able to demand more from those who are generating these models.

Kate Bevan: Phaedra, thank you so much. That's a great place to leave it. Thank you so much for joining us.

Phaedra Boinodiris: Oh, the pleasure is mine. Thank you for hosting me.

Kate Bevan: The AI Interrogator is an Infosys Knowledge Institute production in collaboration with Infosys Topaz. Be sure to fol-low us wherever you get your podcasts and visit us on infosys.com/iki. The podcast was produced by Yulia De Bari and Christine Calhoun. Dode Bigley is our audio engineer. I'm Kate Bevel of the Infosys Knowledge Institute. Keep learning. Keep sharing.
About Phaedra Boinodiris
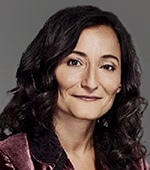
A fellow with the London-based Royal Society of Arts, Boinodiris has focused on inclusion in technology since 1999. She currently leads IBM Consulting’s Trustworthy AI Practice; she is the AI ethics board focal for all of consulting and leads IBM’s Trustworthy AI Center of Excellence. She is the co-author of the book ‘AI for the Rest of Us’. Boinodiris is a co-founder of the Future World Alliance, a 501c3 dedicated to curating K-12 edu-cation in AI ethics. In 2019, she won the United Nations Woman of Influence in STEM and Inclusivity Award and was recognized by Women in Games International as one of the Top 100 Women in the Games Industry as she began one of the first scholarship programs in the United States for women to pursue degrees in game design and development.
- On LinkedIn
Connect with Phaedra Boinodiris
- "About the Infosys Knowledge Institute" Infosys Knowledge Institute
- Trustworthy AI
- London Interdisciplinary School
- “Generative AI Radar” Infosys Knowledge Institute
Mentioned in the podcast