Knowledge Institute Podcasts
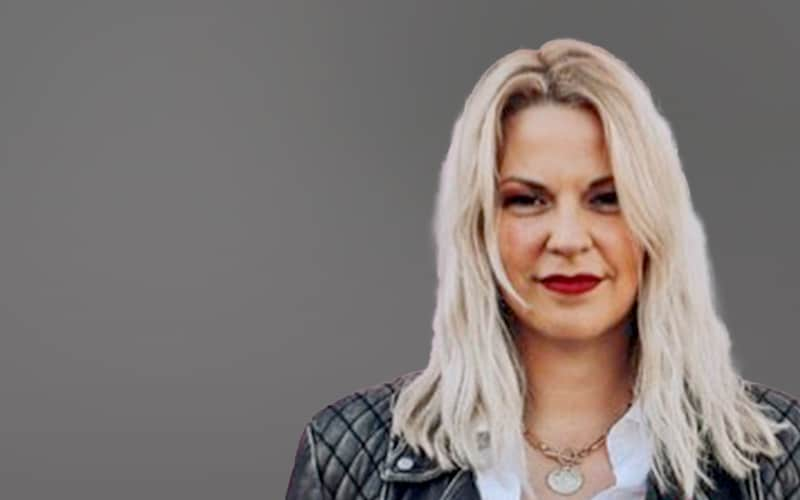
-
AI Interrogator: AI, Data, and the Future of Healthcare with Lauren Bevan
February 21, 2024
Insights
- Proper data labelling in healthcare for AI applications is critical. There is a need for a more comprehensive approach to capturing rich and nuanced information within medical records to enhance the effectiveness of AI algorithms.
- Startups in the healthcare AI sector face challenges. Governments and organizations need to create an environment that encourages innovation while streamlining regulatory processes, ensuring that promising solutions are not hindered by excessive barriers to entry.

Kate Bevan: Welcome to this episode of the Infosys Knowledge Institute's podcast on all things AI, the AI interrogator. I'm Kate Bevan of the Knowledge Institute and my guest today is Lauren Bevan. No relation as far as we're aware, but I think it's fair to describe Lauren as an accidental data scientist. Lauren initially trained as a physiotherapist and worked in major trauma but ended up in data science after shifting into accountancy, so that's quite a journey. Lauren, however, is now the director of consulting at Ethical Healthcare Consulting here in the UK. So has lots of interesting things to say about healthcare and AI. And let's actually start with a fairly broad question. What can AI bring to healthcare Lauren?

Lauren Bevan: So, I think healthcare would benefit from the benefits in a number of different ways. There are global work-force shortages, which AI can help with in order to help augment some of that human decision-making. But likewise, it can standardize practice as well. So, what we know is healthcare outcomes are affected by indi-vidual's clinical practice and their knowledge, which is largely gained through experience once you're out of the training sausage machine and outside of formal education. So that really does vary about the patients that your clinician has seen before you to be able to help them diagnose you properly and be able to give you effective treatment. So that knowledge gained is quite difficult for practicing clinicians to find time to do, especially when paired with a global pandemic and a workforce shortage. It's hard to find the time to read up on everything in the Lancet every week and make sure you stay on top of everything even if you are in a super specialist area. So that can certainly be a really practical application of AI.

Kate Bevan: And how does that work if you do that then? Is it like functioning as a chatbot, going through maybe the Lancet and summarizing stuff for you?

Lauren Bevan: So, part of it is in clinical decision-making tools. So, there are tools which exist, which scoop up all of that data. They make sense of it, and they help people understand actually what the treatment options are. And there are other things, not really like the chatbot side of things because that's quite fallible, but at least giv-ing people a reading list to say, "Everybody else is reading this and you really should have a look at it too. It's got quite a lot of callbacks. Lots of people are referencing it in their papers as well. It's got a quite high Q index. So potentially this is something you should be checking out of journal club."

Kate Bevan: And also, I suppose diagnostics. How are we using AI and diagnostics?

Lauren Bevan: So, there's a really good use case for AI and diagnostics for two reasons. One is the workforce shortage that I've mentioned earlier. It takes a good eight or nine years to train a radiologist. And at the moment we are, I think in the UK I think about 40% sure or something absolutely staggering in terms of radiologist work-force. Given the amount of time it takes to do a medical degree and then to do your postgraduate qualifica-tions and then be a radiologist consultant and therefore operating independently, that's a really long lead time to get people in situ and therefore the gulf is going to grow. So, there's a couple of really interesting use cases in diagnostics.
One is because it is image-led, machines can see things which the human eye can't. For example, if you're a woman of a certain age, you will be invited for a mammogram. And the way that that gets triaged and looked at is that two radiologists will have a look at that image, and they will see whether or not it's normal or whether or not there's anything for concern. And they will then flag what they think it is. At the moment there are quite a lot of algorithms which are training to do that second reviewer. So, you still have a human involved in the process, but you have a machine doing that second review.
So that has two benefits. One is that it performs a training loop. So, if the computer and the human disa-gree, they can then arbitrate between themselves to see who's winning. But then it also just means that that's 50% of the time that's needed to get a clinical opinion on that is taken out so you can get more people through, they can get their results quicker. And it also means that we're quite sensitive about ma-chines taking our jobs. Nobody's losing their job on that because you've still got a human involved in the process and there is always a backlog around people needing mammograms. So again, it just means people get seen quicker and they can have a little bit more faith in the result that comes through that process.
And the other thing, it's interesting, Moorfields have been doing a huge amount of work. So then Moorfields is an eye hospital, the leading eye imaging center. They've done quite a lot of work around feeding quite a lot of these high-grade images into a number of different algorithms. What they've realized is that machines can see things that humans can't. So, there is, for reasons we don't understand, you can tell the gender of a person based on their eye scan, humans cannot do this. And we're unclear at the moment around what pat-terns they are seeing, which they're then interpreting and then able to segment in terms of male and fe-male. And they think it's something to do with the vasculature at the back of the eye, but that is, at the moment, a hypothesis. There isn't in any medical literature a known difference between the genders around how that happens, but the machines can see it. So, we are finding use cases we didn't know we had.

Kate Bevan: That means you could work out further down the track from that data whether one sex or the other is more likely to get an eye condition?

Lauren Bevan: Yeah, so they're using it in macular degeneration at the moment. So, it does throw up the interesting ques-tion of are we asking them the right questions? Are we asking them human questions rather than machine questions?

Kate Bevan: Do we know yet whether machines are better than humans at using images for diagnostics? What do you think on that?

Lauren Bevan: I think the jury's split. I think there are certain things where you're relying on very precise changes and if you've got time series analysis, so say if someone's got a progressive disease, they are quite good at pick-ing up subtle changes and changes that when you're going from one reviewer to the next, because it won't be the same person reviewing if you have a scan every two years for a certain condition, they are con-sistent. So, they have that benefit over a time series. What we also realize is that they're very fallible be-cause they are picking up things and using those as diagnostic tools, which humans wouldn't.
So, a good example is if you're looking for a collapsed lung, you're usually diagnosed with an X-ray to be able to see the amount of basically blackness or whiteness that exists in the lung, they had an algorithm which was put out for proprietary sale. The training data they'd used had a lung drain in it and that was the feature it was using to make the diagnosis. But you only have a lung drain in if you have a collapsed lung because what's used to re-inflate it. So, there was a slight boo-boo in terms of understanding. You have to understand the feature that the machine is using to be able to diagnose. And in that case, this is a post hoc analysis of you don't stick a lung drain in if you don't have a collapsed lung. It's a treatment rather than the diagnostic marker.

Kate Bevan: Well, that actually brings me on to my next question really, which is about the data we use. Good AI, good outcomes rely on good data. Do we have good data? Are we ready for AI and healthcare from a data point of view?

Lauren Bevan: So, if I'm thinking about say UK and Western world generally, in some cases yes, and in some cases no. So, the nuance is that in England in the NHS we have full coverage over everybody. Everybody that lives in the UK has access to the same services, whereas in other places where it's an insurance backed model, you have differentials in terms of what care people can access. They're overly represented in the data in terms of certain things. If you can't afford to get a scan to find out that you've got COPD, you might be living with that condition and you only get diagnosed very late, in which case you are missing all of those early stage disease markers that might help to predict that and help you to get a better outcome. But in the UK, we have that data, we have it over a longitudinal basis, which is great. We have it stored. A lot of it is still in paper. A lot of it is still in dusty warehouses, so it's not digitized. And lastly, it's about data quality, it's about the consistency.
The NHS in the UK exists as lots of different sub-business units. So, it still has the same banner. You still have the same lozenge with its own Pantone color, which we all recognize, but they're separate fiefdoms. They use different systems, proprietary technologies, and they have different file formats, and they also classify things slightly differently. So that variation in practice then feeds through into variation in data. So, when we're looking at national data sets, it's quite hard to get that specificity. And also, there is an element of, again, that rich-poor divide. If you are a university teaching hospital, you typically have much better sys-tems. You are doing research, you are doing clinical trials, all of that stuff, which means your data's really good. You are also often in large urban centers. So, if you're a small district general hospital in a slightly more rural, slightly more deprived area of Great Britain, what that also means is you probably are more like-ly to have paper-based records, which means that your data then is less likely to get used as part of deci-sion-making. It's not in the pot for people to use.

Kate Bevan: How do we compare in the UK to other countries? Do you think the UK is reasonably okay about data? Are other countries more advanced at this?

Lauren Bevan: So, the Scandinavian countries I think are really good. They look at data in a more holistic way. So, what I mean by that is in most Scandinavian countries they'll have a citizen ID, which is then used for your healthcare data, for your national insurance data. So, your taxation for your education, all of those things tie back to you as a citizen. So, we can see, using Scandinavian data what impacts good housing and good edu-cation have on health outcomes in a way that we can't in the UK because we have an NHS number, and we have a national insurance number, and we have those other disparate things that makes it quite hard to get a citizen view of those things to then inform policy. Scandinavia had that in the nineties, so I think it was '96, '97. So, we've got a good couple of years. Good couple of decades of that to be able to see if you pull this policy lever over here, what does this do to the health and wellbeing of a population?

Kate Bevan: And coming back to AI, of course that means when you've got the good data, you can pull the insights and then you can come up with actions from that. If you could wave one magic wand, what would you do to im-prove data for AI healthcare?

Lauren Bevan: So, I would say it's about data labeling. So, one thing that we don't do very well is label our data and have it in correct storage areas. So, if you fall over and end up in A&E, the doctor in A&E they will write a little note and say query fracture of left tibia, and that's what goes to the radiologist. They then report back and say yes or no. Any other interesting clinical features on their x-ray are disregarded because they're answer-ing the exam question that's put in front of them.
What other countries and other economies are doing better in order to prepare for this multifaceted learn-ing approach is that they are labeling everything that's on there. So, say if you have an old fracture that's healed, that will be labeled. If you have a bony spur that will be labeled, if it looks like you've got early signs of osteoporosis or other micro-fractures that will be labeled. We don't do that, which means that we can't get the richness of the data. But that again comes back to workforce and time pressures that if you're asked yes, no question, you don't go into streams about how interesting this thing is, but that's what other countries are paying to do.

Kate Bevan: That sounds like a barrier to innovation the way we are labeling data properly in the UK. What are the barri-ers to innovation in healthcare when it comes to AI?

Lauren Bevan: So, I think there is public understanding about what that means. So how can you give your data up in a safe and secure way for it to be used for the powers of good and not for the powers of evil? And how do you as a citizen have a say about what gets used and when? So, there is this concept around secondary uses of data. What that means in reality is anything that is not used to clinical care, so non-bedside use, but that is really broad. It can still be used by healthcare planners to think where do we need to put new facilities? Where do we need to maybe set up a new clinic over here? Because we're looking at activity trends and understanding, well actually this seems to be really prevalent over here, but there's no service need that is classed as a secondary use.
Likewise, a secondary use is being used by academic institutions to come up with things, drug discovery, all sorts of things. So I think this binary, primary and secondary is not particularly useful way of classifying the uses and it doesn't give for nuance of people who are happy to say, "Well, I'm happy for the NHS or health boards to use it to plan where to put hospitals and clinics, but are not happy that a third party insti-tution who is a full profit-making thing uses my data to make money off the back of my information." At the moment, the GDPR and the UK data sharing legislation and other things don't make the distinction beyond those two categories, which makes it very hard for people to have anything. If they have any objection to anything in that secondary data pile the reflex is to say no to all of it.

Kate Bevan: Yes, I remember when we were talking about the COVID app a couple of years ago. During the start of the pandemic, I was very much team, actually we should have a more open COVID app that gives planners in-sights into the pandemic, where the hotspots are, where the provision is. And the app we had didn't allow for that, but it was very private. And I remember that being a tremendous tension at the time. Is that pre-sumably still very much a tension?

Lauren Bevan: It's a tension in every data use case you can imagine. It's just that's something that brought it to the fore, and I think the use of the DNA data around what was happening with the disease was happily given up to big pharmaceutical companies who could use it to do vaccine development. So, I think people saw that as a beneficial use case. But I think that there isn't enough public dialogue about what those things mean. And I think going back to my earlier point around the NHS being all of these different fiefdoms, there is a misun-derstanding that an ambulance service does not have access to your data. If you have said that your GP can't give it to anywhere else, what that means is it stays in that GP practice, or it stays in that hospital or it stays literally in that ambulance. By not sharing for those secondary uses, they don't know who your next of kin is. They don't know what your drug allergies are. Those things aren't shared.

Kate Bevan: There's a lot of interesting startups in this space. It's also been a couple of quite spectacular failures. I'm thinking of Babylon. How do you see the role of the private sector, particularly in the AI in healthcare?

Lauren Bevan: I think that the bombastic individuals, and I am thinking about Babylon in this, who over-promised and under deliver, undermine the other people that are doing the slow and steady. I think particularly those academic spinouts who have got a quite good grounding in how to do this thing and how to bring it to market and how to make it work well, I think are, I guess the people to look at really because they've got the good foundations in terms of understanding how the data can be used, not overselling it.
I was talking to an SME on Monday and there were a lot of people that were saying that some of those smaller startups are starting to go out of the UK and they're starting to go to other areas because they're finding it too hard to do business because of the requirements, not just of all the regulators, which I think most people would argue is totally fine, so NICE, MHRA and all those people that make sure that things are safe and secure. But the challenge around actually how to sell the benefits and the use cases into different areas and understand who the buyers are, it can be a really hard market for people to break into. Even if they've got a really good product, even if it's sound, even if it's had all the rubber stamps, it's incredibly wearing on having the door shut in your face, not understanding how to access procurement routes. And generally, people end up worrying that they're going to run out of cash, and they exit to go into different global markets.

Kate Bevan: How do we build trust in companies that are private sector where they are doing something incredibly use-ful, have something to bring to the table?

Lauren Bevan: I think it's about how governments and organizations can safely welcome innovation, what barriers that they need to put in necessarily to make sure that that only the good organizations get through. But likewise, making sure that a pilot in an analogous health institution should be enough to get people through. If you've proven it once and it's safe and secure and people like it and people have benefited from it, if you've got a broadly similar population, there's no reason that you need to do another six-month pilot before you sign up with a contract with that organization. So pilotitis is what I call it, but that's the kind of barrier that I think a lot of people face with innovation.

Kate Bevan: That brings me on, we were talking about trust to the question I ask everybody to finish off with is do you think the AI is going to kill us all? Particularly this is interesting in context.

Lauren Bevan: Yes, but accidentally. I don't think that's what it's going to set out to do. If we don't allow it to train on enough data, it's not going to be sufficiently well-versed in how to do its job. Just like anything, if it's only given apprentice work, it's going to be operating at apprentice level. But likewise, if there isn't sufficient views about how it can be used and starting to get it working at foundational level, or maybe operational stuff. So, scheduling all of those things that is done with pen and paper a lot of the time on the fourth-biggest global workforce, which is largely scheduled using pen and paper on how to do off duties, then those are the safe training areas in order to build the confidence that it can do something greater.
So, I think that there is a risk that we rush to put it at the top of the tree and say, "Tell me how long I'm going to live and tell me what I'm going to die from," and all of these things and diagnose me without get-ting it settled in making sure that there's enough people at nine AM on a Tuesday to run a ward. So, I think it will be in application and not design.

Kate Bevan: That's actually reassuring, I think. Lauren Bevan, thank you very much for joining us.

Lauren Bevan: Thank you.

Kate Bevan: The AI Interrogator is an Infosys Knowledge Institute production in collaboration with Infosys Topaz. Be sure to follow us wherever you get your podcasts and visit us on infosys.com/iki. The podcast was produced by Yulia De Bari, Catherine Burdette, and Christine Calhoun. Dode Bigley is our audio engineer. I'm Kate Bevan of the Infosys Knowledge Institute. Keep learning, keep sharing.
About Lauren Bevan
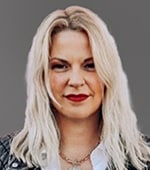
As director of consulting at Ethical Healthcare Consulting, Lauren leads the delivery teams on projects and is responsible for the external-facing brand and its propositions. With a clinical, financial and technology background, she has worked for almost 20 years on helping the health and social care system in the UK and overseas to be more responsive to patient needs and to reduce waste and frustrations of clinical and opera-tional staff of the sector – including senior roles at BJSS, EY, PwC and the NHS. She’s a firm proponent of boring basics and would like to see a day when the NHS has nothing keyed into an aged Excel spreadsheet.
- On LinkedIn
Connect with Lauren Bevan
- “About the Infosys Knowledge Institute” Infosys Knowledge Institute
- Do Comprehensive Deep Learning Algorithms Suffer from Hidden Stratification? A Retrospective Study on Pneumothorax Detection in Chest Radiography Seah J, Tang C, Buchlak QD, et al, BMJ
- The Fall of Babylon: Failed Telehealth Startup Once Valued at $2B Goes Bankrupt, Sold for Parts
- “Generative AI Radar” Infosys Knowledge Institute
Mentioned in the podcast