Knowledge Institute Podcasts
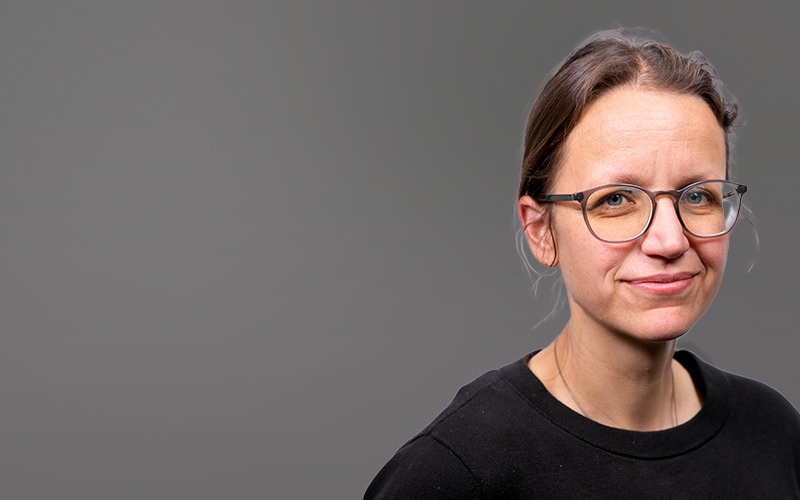
-
Balancing Innovation and Responsibility in AI with Francine Bennett
December 19, 2024
Insights
- The fast growth of AI technologies requires a stronger ethical framework. Transparent data usage and responsible innovation are key to minimizing risks and ensuring AI benefits society fairly.
- Embracing diversity in AI leadership drives innovation and creates technologies that meet a wider range of societal needs. It also helps reduce unchecked biases.

Kate Bevan: Hello and welcome to this episode of the AI Interrogator, Infosys' podcast on enterprise AI. I'm Kate Bevan of the Infosys Knowledge Institute and my guest today is Fran Bennett, who is a data and AI specialist and she's a board member at the Ada Lovelace Institute. Fran, thank you very much for joining us today.

Thanks for having me.

Kate Bevan: Can you just tell us me a little bit about what you mean by being a data AI specialist? What have you been doing around AI?

Francine Bennett: So my background's actually in mathematics, so a very, very long time ago now, I studied maths and philosophy just because I thought they were very interesting topics. The maths, obviously you're thinking very, very analytically, but then the philosophy gives you a chance to zoom out and say, well, it's not just about a correct answer or proving a theorem. It's like, why? What's it for? What does it mean to ask some maybe quite pretentious but important questions about all those things. And at the time I was just very interested in those, but then my career later led me as we all started to realize data was very important and then you could build things with data it turned out. And then later on we are really realized you can build quite socially impactful things with data. It is quite useful to be able to think in both of those framings, the technical and also that social and ethical aspects of it all.

Kate Bevan: Yeah, I suppose it kind of makes you almost a perfect person to be looking at AI and how we're using it now.

Francine Bennett: Oddly, yes, it's sort of come around that way through a very, very circuitous route. So I spent quite a lot of time building things but also trying to say, well, what should we build? How should we do it? What's the right way forward?

Kate Bevan: I mean, that's probably the next question, isn't it? What should we build? And also maybe who should be building it?

Francine Bennett: That's an interesting one right now, I think for a long time obviously there've been a lot of interesting things being built with data and with AI and in the last couple of years it's suddenly become a very, very hot area and there's suddenly been a real narrowing of the people influencing the conversation and doing the building.

Kate Bevan: Maybe one of the things we need to think about now - are women prominent in enough AI? Is it too narrow as a range of people who are working in this?

Francine Bennett: I think at the moment it really is too narrow, and I'm a little bit reluctant to say that because I don't want to say women should be there just because they're women. It feels very tokenistic and I don't want to be in rooms because of my gender. I want to be in rooms because I'm good at what I do. But at the same time, when you look at the groups of leaders being consulted and making the calls, it's very visible, particularly in terms of gender because it's so easy to see that it's a very narrow group of typically white male, Californian, 30-somethings, who have a very specific range of life experience and particularly when the technology is so fast moving I think they're making a lot of judgment calls from their life experience, which may not be the right ones for people with other experiences.

Kate Bevan: What kind of judgment calls do you think we should be making that reach outside that range of experience?

Francine Bennett: I mean, it's hard to pin down one thing, but as an example, if you look at the AI image generation tools at the moment, those generate images based on the training data that they have obviously, which is great from the internet. Choices are made about what training data to use. Choices are also made frankly about what kind of outputs are acceptable to have or to unacceptable. So know in text and image generation, certain constraints get put on those so that they don't just generate all sorts of things, which we find socially unacceptable.

Kate Bevan: What do women bring to AI that we're not seeing enough of?

Francine Bennett: It's both the things that are getting built right now, thinking about how to shape and shepherd those and how to roll them out. I think it's also just thinking about what problems do we want to address or what should we be building at all? There are all sorts of interesting turns of what's being worked on and loads and loads of focus, for example, on weaponry at the moment, which to massively stereotype, it might be a sort more male thing to naturally gravitate towards. I think if you had more mixed other people I think involved too, you would probably start to think about just different product sets you start to solve for different types of things.

Kate Bevan: How do we make data sets and companies creating and using those data sets more transparent?

Francine Bennett: I think part of it is government and policy. I think there's a really challenging situation right now where governments are in this position where they want to create good policy and regulation for AI to work on behalf of people and society because it's the role of government. But at the same time, there's an intense anxiousness about missing the boat on an industrial and economic thing that could be very important. And trying to strike that balance is incredibly hard, but I think governments could go a lot further in pushing for at least more transparency on what data and models are, what's going into them, how they're working, so that people have a chance to really inspect and understand what they're using.

Kate Bevan: Are we doing a good enough job on regulation at the moment? For me, it feels like we are making a big effort to be out in front of it in a way that we weren't with things like social media. Am I right in that feeling or...? I mean, you see it closer than I do.

Francine Bennett: I think you're right. I think there is a lot of effort and thought going into it. I think it is incredibly difficult at the moment, so I've got enormous amount of sympathy for the people trying to work out what good regulation might be here because it's not obvious. There are a lot of genuine unknowns, which cannot be known at the moment and government can't really move fast enough to be on top of everything. But I would love to see a little bit more proactive regulation, a little bit more thought about how can it be that government can move fast enough to respond, as you say, to not get into a social media situation where you wait for harms to arise and then you go, oh my goodness, now what?

Kate Bevan: What kind of leadership challenges do you think companies face when we're dealing with AI?

Francine Bennett: It is challenging both from the leadership of AI companies and also for leadership of other companies to work out how do I engage with this? Because I think if I were running a large corporate right now, I would be thinking about, oh, how does this technology help me? How does it give me a competitive edge? But also, again, there's a lot of genuine uncertainty right now about it. It's an opportunity for an edge, but it's by no means certain the pathways aren't well trodden. So you've got to sort of simultaneously be innovative and excited, but also just withhold judgment so that you're able to change course in a flexible way as you learn more.

Kate Bevan: But that feels like a real challenge when there's so much... There's just this torrent of information about AI is going to make the business more productive. It's going to do this for you, and if you can't work out where you need to be ready, then that means you're not going to get the best out of it.

Francine Bennett: Most companies would benefit, in my opinion, from having better data quality and thinking more coherently about their data. No matter what else they're doing that's going to be a useful resource for them. And generally it's not as good as it could be. So that will always see you right, dealing with your data quality. You can build fancy AI with it, or you can do much simpler things with it, but it's going to be a useful investment. So just sort of slowing down for a moment and thinking about those basics and how can you realize benefits from simpler technologies already is worthwhile I think.

Kate Bevan: That's a really good piece of advice, actually. Do you think we are at the top of the hype cycle on AI? Are we heading for some retrenchment and realism, or have we got more to go on the hype?

Francine Bennett: I think it's going to slow down a lot. I maybe made a fool of by this comment, but I think it's going to slow down a lot in the next year because everyone has got so much expectation built up, and I don't think we can realize the kind of things that we've been hoping for in a short timeframe. I think longer term it will, particularly generative AI, will probably prove to be quite significant. It's just going to take longer to play out, and I think it's not about keeping on top of the very latest release. It's about saying, well, even the things that we already have, how are they going to play out over a longer period?

Kate Bevan: If you could give one piece of advice to leaders about using AI in their businesses, what would that piece of advice be?

Francine Bennett: I would say just be very clear about your business strategy and get that very clear because then working out how AI helps you is much easier. So you say, what outcome am I actually seeking here? Do I have a problem with internal productivity? Okay, now you can see how AI links to that, or do I have something customer facing I want to think about? And then AI can link to that. I think where I see things going awry is where business leaders are saying AI, that's very exciting. Now where can I apply it without framing it in any current way. So if you know what your outcome is, then you are much more able to rethink and say, oh, well, maybe this could work, but it turns out our data quality is not there for example. Okay, let's fix that. Or it turns out the technology's not quite at the precision that we would need for the outcome we want. Okay. So we have to park that for another N months until the technology gets there. But just knowing what those outcomes are that you seek lets you be much more effective I think in your applications.

Kate Bevan: Where have you seen effective uses of AI? What is effective for businesses?

Francine Bennett: I mean, I've seen very effective uses in the sort of more traditional AI, sort of supervised predictive models.

Kate Bevan: So what do you mean by traditional AI?

Francine Bennett: So what I mean is machine learning based on data making predictions. So expert systems as the sort of previous wave. And then you've got the machine learning wave, and it can be very, very helpful, particularly in highly digital, highly online businesses where there is a lot of data. You can use that data and make predictions which just enable better allocation of resources, better decision making, better prioritization, and it works really well. And it's much, much simpler than generative AI. And we've been around it enough times now that we understand what the success modes and the failure modes of it look like. So it's quite well-worn, but then that makes it almost unexciting.

Kate Bevan: Yeah. I mean, are you seeing effective uses of generative AI?

Francine Bennett: I'm seeing some interesting experiments, and I think there are cases when it can be really helpful in sort of applications like customer service or summarization seem quite effective.

Kate Bevan: I'm going to come around to my final question, what I always ask everybody is do you think AI is going to kill us?

Francine Bennett: No.

Kate Bevan: That's a nice clear answer.

Francine Bennett: But I think there are lots of important things to think about with AI, which are actually real problems as well as opportunities. So there's the energy impact. There's the concentration of power, which I think is little discussed and is actually quite important. I think climate change is going to kill us frankly before AI, unfortunately.

Kate Bevan: Where do you think its promise lies?

Francine Bennett: I think it does have promise for all sorts of automation, interesting detection of patterns. I mean, there is a lot of drudge work that frankly AI can do in the way that computers and calculators are great to have taken out a lot of that drudge work and have changed jobs as a result. Bookkeepers don't write down columns of numbers anymore. That's not a thing. We don't have elevator operators anymore. Lifts just have buttons. Jobs do change over time as technology alters them, and that's a positive and interesting thing.

Kate Bevan: I think that's a great place to leave it. Fran Bennett, thank you very much.

Francine Bennett: Thank you very much.

Kate Bevan: The AI Interrogator is an Infosys Knowledge Institute production in collaboration with Infosys Topaz. Be sure to follow us wherever you get your podcasts and visit us on infosys.com/IKI. The podcast was produced by Yulia De Bari and Christine Calhoun. Dode Bigley is our audio engineer. I'm Kate Bevan of the Infosys Knowledge Institute. Keep learning, keep sharing.
About Francine Bennett
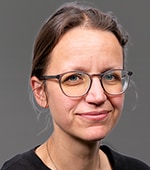
Francine Bennett is a data and AI expert, with a background in building new products and services with data and using AI/ML. Her experiences include founding and running a startup in the early days of ‘big data’, advising government entities on how to use data better, and running data and AI teams at a drug discovery scale-up.
Most recently, she was interim Director (and current Board member) of the Ada Lovelace Institute, a Nuffield Foundation funded organisation which seeks to make AI and data work for people and society. In that role, she has been a central part of the UK and international AI governance conversation, including joining the Bletchley Summit and appearing in media including R4 Today and BBC TV. She was also a founding trustee of DataKind UK, which provides pro bono data science support to UK charities, and a board member of Bethnal Green Ventures.
She has been an invited speaker and advisor on data and AI topics for organisations including the Royal Society, the Economist, and the UN.
- On LinkedIn
About Kate Bevan
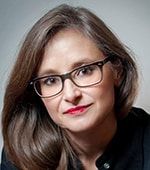
Kate is a senior editor with the Infosys Knowledge Institute and the host of the AI Interrogator podcast. This is a series of interviews with AI practitioners across industry, academia and journalism that seeks to have enlightening, engaging and provocative conversations about how we use AI in enterprise and across our lives. Kate also works with her IKI colleagues to elevate our storytelling and understand and communicate the big themes of business technology.
Kate is an experienced and respected senior technology journalist based in London. Over the course of her career, she has worked for leading UK publications including the Financial Times, the Guardian, the Daily Telegraph, and the Sunday Telegraph, among others. She is also a well-known commentator who appears regularly on UK and international radio and TV programmes to discuss and explain technology news and trends.
- On LinkedIn
- “About the Infosys Knowledge Institute”
- Infosys’s Enterprise AI Readiness Radar
- “Generative AI Radar” Infosys Knowledge Institute
- Ada Lovelace Institute
Mentioned in the podcast