Knowledge Institute Podcasts
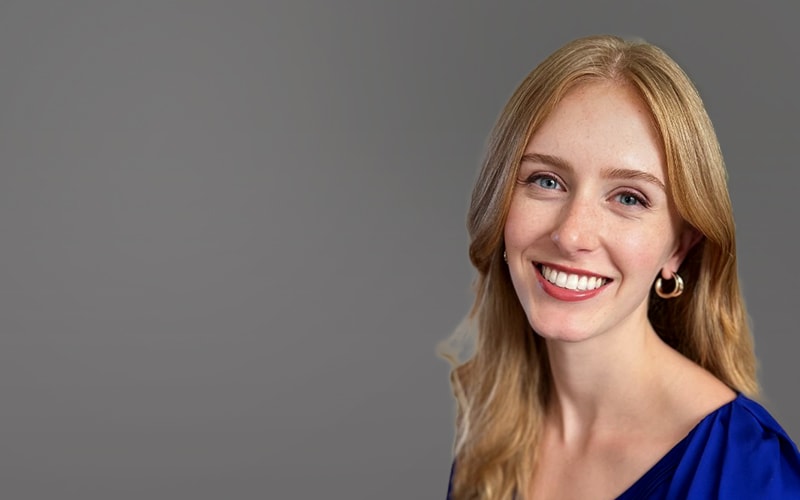
-
AI Interrogator: How AI Transforms Automation and Enterprise Workflows with Appian's Gabby Rothschild
May 30, 2024
Insights
- AI's integration into automation is transforming enterprise workflows, reducing manual labor, and increasing efficiency across various industries by handling complex and repetitive tasks.
- The future of AI in traditionally slow-to-adopt sectors like healthcare and government holds significant promise, potentially revolutionizing processes and solving critical issues with advanced automation and data management capabilities.

Kate Bevan: Welcome to this episode of the Infosys Knowledge Institute's podcast on all things being AI first, The AI Interrogator. I'm Kate Bevan of the Infosys Knowledge Institute, and my guest today is Gabby Rothschild, who works at Appian, which is a cloud computing platform. Gabby, thank you so much for joining us. It's lovely to have you.

Gabby Rothschild: Thanks, Kate. Lovely to be here as well.

Kate Bevan: Tell me a little bit about what you're doing at Appian. I know Appian works a lot with automation, and one of the things we've been looking at is automation and AI. What do you think the impact AI is going to have on automation on jobs?

Gabby Rothschild: Yeah, so I'm currently a product manager at Appian, and Appian is a low-code software company. So, we primarily work with very complex enterprises who have quite a lot of manual work and difficult workflows to automate. And so, since the beginning of Appian or its genesis, we've always aimed to automate various processes through new invention and through low code as well. So, we introduced something called the Data Fabric to help companies manage and connect their data across workflows and processes. And Appian has always aimed to reduce the amount of overhead and lift it takes to automate enterprise processes, especially ones that are highly complex and require a lot of rules and people involved in them. So, to answer your question, what do I think the impact of AI will be on jobs related to automation in those manual processes? I think that AI will first come for tasks that are highly repetitive and being able to quickly complete those tasks.

Kate Bevan: What sort of tasks are we talking about there?

Gabby Rothschild: Yeah, I would say things like extracting the same amount of information out of emails, documents, or workflows over and over again. For many years, Appian and many other companies have tried to automate doing that with machine learning and reached a certain amount of automation, but it's still a highly manual and difficult process because documents and communication between humans is very complicated to understand. So being able to leverage something like a large language model to really understand what is being said in a piece of communication and almost function as a human to do that very repetitive task, I think will be a huge boon to those industries that require that. And so, I think highly repetitive tasks, especially around extracting information or generating similar pieces of content will be the first place where AI will have an impact on enterprise.

Kate Bevan: You talked about trying to use machine learning through that and how AI is doing it better. What's the difference between the two there?

Gabby Rothschild: So, machine learning is kind of a subset of AI.

Kate Bevan: People use the two terms almost interchangeably, and there is a difference, and I wanted to get into that.

Gabby Rothschild: And so, when I say machine learning, I mean more kind of classical machine learning approaches where you're actually training a model based on your data in order to automate a task. So, we have that capability at Appian, for example, the ability to train a model to extract information out of documents by uploading documents and annotating where that information should be extracted from. And then using various algorithms and feature extraction methods in order to create a model fully based on that data. And so now when I'm referring to AI, I am more referring to, and I should be more specific, generative AI and the ability to leverage a pre-trained model on a huge amount of data from across the internet and across various sources. So, it really understands human language and semantic positioning of words rather than that specific subset.
So, the pro is that it can target a lot more problems by leveraging this model that doesn't require any training. And the con is that if you need something highly specific for a very targeted task that is specialized, it might not be as good at that because you aren't training the model. So that's why we see in the medical field, for instance, the need for specialized LLMs that are trained on medical data for specific tasks because the accuracy with which you need to complete that task is high. We need to be accurate if we're making medical decisions, that sort of thing.

Kate Bevan: So presumably that also means you can buy an LLM product pretty much off the shelf and not have to do all the data preparation yourself?

Gabby Rothschild: Correct. And that's the approach that we're taking at Appian, plus the ability to instruct the model and provide examples of your specific scenario or problem in order to have the model behave a little bit better. So that is kind of that field of prompt engineering that we hear a lot about the ability to instruct the model to behave in a certain way. It doesn't always listen, but providing specific instructions or examples of how to complete the task accurately has been found to drastically increase the accuracy of completing that task. So that's kind of where we're focused at Appian to both reduce cost for enterprises, but also increase the efficiency in which the machine learning or the LLM model is operating.

Kate Bevan: I think one of the things I'm getting a sense of as we get further into the generative AI age is a sense that the big public LLMs are going to be increasingly less useful, and the focus is going to be more on training, having a version of it for your own documents. Am I right in thinking that?

Gabby Rothschild: I think what will happen first is kind of the advancement of those larger LLMs that are built for general use. So, I'll be very interested to see what happens with the next release of GPT, for instance. So going to GPT-5 and the delta between the current version of 4 and seeing how much better we can be at tasks that struggles with. Because the delta between 3.5 and 4 was so great that I wouldn't be surprised if the next version of these large language models, which are so rapidly progressing, are so much better at those specialized tasks. And it kind of negates the need for training specialized machine learning models, which is really costly for enterprises to do.
And also, just very difficult because you need so much data when gathering and cleaning and processing that data is something that we've seen people have a really big challenge with.

Kate Bevan: So, when we talked about it before, we realized that we are at both at very different ends of our careers. You are right at the start of your career, and that's really exciting because you've got a huge, long horizon of where AI might go and the impact on jobs. And I'm at the end of my career, I mean, I'm old enough to remember when hot metal was being phased out in journalism when we moved to computerized type setting. So, there's a big gap there. With the focus you have particularly on automation, what do you think the impact of automation and AI-driven automation is going to be, not just on jobs, but on the way, we work, going right into over the next 30, 40 years of your career? Where do you see that going?

Gabby Rothschild: Yeah, I think we'll see AI become very mainstream in a variety of industries. I would say that right now it is very hot within enterprise and within various consumer technologies that we're already used to seeing a lot of personalization with AI, so Google Ads, Netflix, Spotify, those sorts of companies. But I think we'll start to see a big penetration within industries that have been traditionally slow to adopt new technology. So, the areas where I'm the most excited to see AI make a big impact are going to be within government and healthcare because we've seen that they're still very reliant on fax machines. So-

Kate Bevan: Fax machines were hot when I was starting out in my career.

Gabby Rothschild: It's because those institutions are so complex. It is very hard to initiate change because you have to initiate it so broadly and overhaul very deeply entrenched processes across thousands of people. And you have to ask yourself, is it worth it? Is it worth the effort of going through that change based on the value that I'll get back? And I think for a lot of previous technologies, the answer has, but no, it's not worth it. Even if it does provide value and it does provide more automation or an easier task or making something faster, the process by which you have to go and make that change is just too onerous. So, I think that the difference with AI here is that the value add is so high that it will be worth it for those institutions to make those changes.
So, for example, within healthcare, we see so many enterprises struggling with just the sheer amount of information they have to process and understand because obviously there are a lot of great clinical uses for AI and being able to detect disease and that sort of thing. And I really hope AI is going to make a big impact in that area. But even starting with non-clinical use cases and being able to look at a patient record and help doctors and practitioners find the information they're looking for quickly can reduce so much overhead and make an impact on the lives of patients and practitioners so tangibly. That I'm very excited to see what is going to happen with innovation in those industries that have taken a long time to progress.

Kate Bevan: One of the things I think we're seeing in AI development, particularly in automation and augmenting human work, is it frees up high status, high knowledge people who do more of that. What do you think the impact will be on people who do the less high knowledge work? Are you worried about that? Are you excited about other opportunities?

Gabby Rothschild: I tend to lean more optimistic, so I'm excited about opportunities, but I also do recognize that there could be a huge disruption as there always is when new technology comes out and tends to first affect people who, like you said, are in those types of roles. I would say that it can be transformed into an opportunity to maybe up-skill those people in those roles that might get affected by AI to be operating in those more high skill, high knowledge roles as well. And so, I think companies need to be mindful of that and invest in their workforce. Education needs to be mindful of that and preparing the workforce for the types of jobs where they can be contributing to the future direction of AI and working alongside it as well. I still think it's incredibly important to have humans working alongside AI and being able to do their job faster or more effectively and just ramp up the pace of change overall. And so, I think being mindful of that in education and the workforce is going to be extremely important going forward.

Kate Bevan: I think that's really interesting as well, because one of the things we've been seeing in our research on AI, both in generative AI radars and some research we've got coming out about banking tech is it's really hard to get the right talent for AI. And so, there's an opportunity there for companies to take some of their workforce and as you say, re-skill them. Are you seeing that happening already?

Gabby Rothschild: I would say directly within enterprises that I'm working with at Appian, I'm not seeing it happen too much already. I think there is still a great need for humans to be involved in these processes that are still manual because at the end of the day, there are going to be pieces of it for humans that need to review or step in. We work a lot with case management use cases, and so there is a need for always a human to kind of be involved to handle more complex cases that have been long-running or require empathy or require creativity or a new problem. That is the key thing with I think AI and automation is AI is not too great yet at encountering kind of new problems and extrapolating patterns. It's getting much, much better, especially with large language models that jump between that, and kind of traditional machine learning approaches was a really great... But I think that being able to always involve that human alongside the AI in order to being able to solve a new problem or do something more creative or provide that empathetic ear will be important as well.

Kate Bevan: You said you don't have any children yet, and maybe you'll never have children, but thinking about your potential future children or the future children of your friends and your family, are you excited about how AI might change their working life?

Gabby Rothschild: I am excited. I think the thing that I'm most excited to see progress from with AI and large language models specifically is for really difficult problems. So being able to cure diseases, being able to make large advancements in areas of climate change. And so, I am very excited and optimistic for them that they will hopefully be able to benefit from some of those discoveries and also potentially even be the people helping lead the AI to make those discoveries. And so, I think that the next generation will be in a strong position to lead change with AI and be better educated than someone like me who went through school without having to understand how AI and language models work.
They'll just be more natural at using that technology and having it integrated into their life, and therefore also more accepting of being able to use it. So, I know that there's still a lot of pushbacks about... We don't want AI to drive our cars. We don't want AI to drive our planes. We're very used to having humans able to do that even though most accidents and errors are caused by humans, and automation would be much stronger at some of those tasks. And so, I do think we'll see a shift in the acceptance of those technologies to drive previously humans under decisions or processes.

Kate Bevan: Because the kids being born now will be the ones going into the 22nd century, which is quite mind-blowing when you think about it. Think about what AI might be like in the 32nd century, which actually brings me onto my final question. It's a question I ask everybody, which is, do you think AI is going to kill us?

Gabby Rothschild: And I've heard this question before, obviously there are a lot of takes on it. My take is that I just don't know yet. I think that there is a famous quote from Carl Sagan, "Extraordinary claims require extraordinary evidence or extraordinary proof." And I don't think that we have that proof yet. We're still seeing language models struggle at basic tasks or generating information correctly. And so, I still think that there is a long way to go, and there are many barriers to hitting AGI or hitting a place where we have the infrastructure, the knowledge, and the data that is required to train models that are that powerful and present in society as well. And so, I think that right now, I don't think so, but AI changes so much every day that my answer could be different tomorrow, but I believe it has a long way to go.

Kate Bevan: Maybe I'll come back to you next year. Gabby Rothschild, thank you very much for your time. It's really brilliant talking to you.

Gabby Rothschild: Thank you all for having me. It was great to talk with you Kate.

Kate Bevan: The AI Interrogator is an Infosys Knowledge Institute production in collaboration with Infosys Topaz. Be sure to follow us wherever you get your podcasts. And visit us on infosys.com/iki. The podcast was produced by Yulia De Bari and Christine Calhoun. Dode Bigley is our audio engineer. I'm Kate Bevan of the Infosys Knowledge Institute. Keep learning, keep sharing.
About Gabby Rothschild
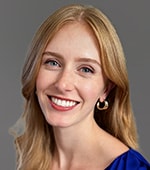
Gabby Rothschild is a Senior Product Manager at Appian, where she develops AI and machine learning products. Prior to Appian, Gabby worked as a consultant at Yext with enterprise clients. Gabby earned a B.A from the University of Pennsylvania, and currently resides in Washington, DC.
- On LinkedIn
Connect with Gabby Rothschild
- “About the Infosys Knowledge Institute”
- “Generative AI Radar” Infosys Knowledge Institute
- Appian
- Infosys Topaz
Mentioned in the podcast