Knowledge Institute Podcasts
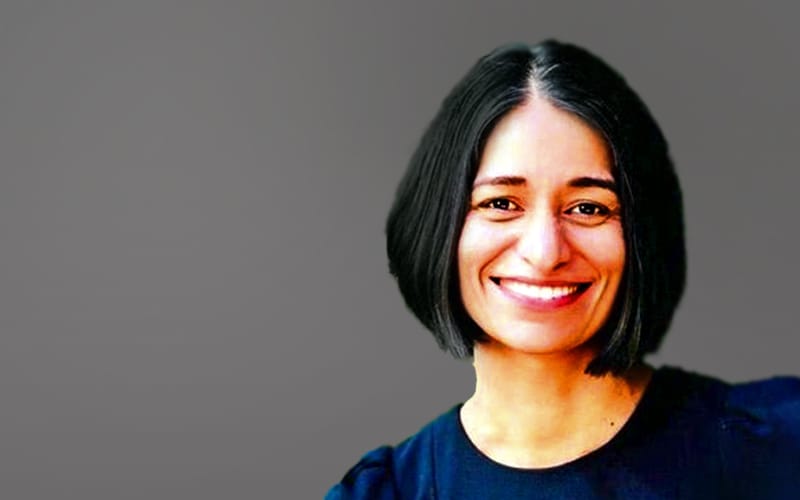
-
AI Interrogator: AI for Sustainability with Google’s Miku Jha
April 16, 2024
Insights
- Miku Jha's emphasis on starting with the problem rather than the technology itself highlights the importance of a problem-centric mindset in AI development. By identifying real-world challenges and considering how technology can be leveraged to solve them, organizations can ensure that their AI initiatives are purposeful and impactful.
- The discussion on the integration of sustainability and responsible AI practices underscores the growing importance of ethical considerations in AI development. By incorporating principles such as energy efficiency, environmental impact, and user safety into AI frameworks, organizations can ensure that their AI solutions not only deliver business value but also contribute positively to society and the environment.

Kate Bevan: Hello and welcome to Infosys's Being AI-first podcast, the AI Interrogator. I'm Kate Bevan of the Infosys Knowledge Institute, and my guest today is Miku Jha, who describes herself as a serial entrepreneur. She's now at Google Cloud overseeing their approach to using AI responsibly and sustainably. Miku, thank you very much for joining us today. It's great to have you.

Miku Jha: Thank you, Kate. Glad to be here with you.

Kate Bevan: Well, I think I'd like to start by joining the dots on your serial entrepreneurship and how you've ended up at Google, because certainly it seemed for you when we spoke about it initially that there's a really clear path between what you started out doing and what you're doing now.

Miku Jha: It has been pretty much like lot of decisions from the heart as opposed to from the head, but it has always been that how do you solve the problems, which can have a real impact? And technology just happened to be a way to ex-press that or a way to solve it as opposed to, "Okay, I have this. Let me go and see how I can apply it."
One good example of this, Kate, is when I did my last startup, which was an AI startup back in 2014. It just started from attending many, many different conferences and having this notion that we really have to think about provid-ing more food because of the growing population, and it seemed to be a major, major goal. But then if you boil it down very simply, you think of what if we wasted less and wasting less is something which we can tangibly do some-thing about, because you can't just grow more food. You are bounded by however much land you have.
So, it's like answering those fundamental questions, which helped me make every single decision. Not all of them were, in hindsight, right because hindsight is perfect, but at that point it did seem like using technology could help. Even if you solve a small percentage of a big problem, then you have solved something big, right? That's the philoso-phy with which I am where I am and continue to do so.

Kate Bevan: How are you using technology and AI techniques to solve food problems?

Miku Jha: So, we just started thinking where can you have a maximum impact in reducing food waste? And that ended up be-ing in the supply chain because that's where millions and millions of fresh produces goes through quality inspection and has pretty suboptimal manual processes. And then you go through quality assessment. 100 berries is a sample. If it gets rejected, you have literally wiped off one year worth of a grower's effort to grow that produce, and it has a monetary value. It could be 10 million worth of shipment.
So, we just thought, okay, what if there was a more rational, better, objective way of looking at the samples and making such an important decision of do you want to accept the shipment or not? So that's where it started, Kate. Again, it was just like, how do we make this better? How do we make this better for the business? How do we make this better for the grower? How do we make this better for the environment? When you bring all of that together, it was like, okay, we can make it more objective.
So very simply, rather than inspecting 100 berries, can I inspect a 1,000? And half of the time you have more data points, you're going to make better decision. It wasn't really anything more complex than that. And I think that's what helped us have those conversations with growers, with businesses.
AI ended up being a logical way as opposed to a forced way. So, it was like, okay, I need to look at images and a sys-tem can do a much better job of finding all the different visual patterns which goes into making those decisions. So that's what we did. We took the model; we trained them to look at patterns and built a full-fledged solution to help the entire supply chain just become better at assessing the quality.

Kate Bevan: That's a really interesting way of approaching quite a hard problem. How did that though lead you to Google and try-ing to make the cloud more sustainable?

Miku Jha: Yeah, if you think about it, aren't we in many ways at a similar point? When I did the startup, this was 2014. But to-day-

Kate Bevan: That's 10 years ago.

Miku Jha: Yeah, it feels like wow. But I think it's in the sense today where we are at, even being at Google, I cannot keep up the pace with the innovations even though I'm part of it. So, at this pace, and given where we are at with AI, a big aspect of if you fast-forward 10 years from today would be not that did these solutions work? Did they deliver busi-ness value? That's an important point, but I think me and you, when we had another podcast 10 years from today, we would say, "Were we responsible? Were we sustainable? Did we put the right guardrails to ensure the impact on the environment? Did we put the right guardrails to ensure that the users are safe? Did we put the right thought processes to ensure that the data is protected?"
So those are the questions which I think we want to answer, and that's where Google is a very logical choice given all the things that we are doing in these aspects, in these hard problems and hard questions that we have put together as part of our solutions and offerings.

Kate Bevan: Isn't there a tension between doing it sustainably and getting business value from it? How do you manage those tensions?

Miku Jha: I don't think there is. I think one leads to the other. What I mean is that first of all, it really starts from any organiza-tion., If I just take an enterprise-focused approach, that they should be starting with what are their AI principles? It's very important. It's very easy to take solutions and put something together but if you're thinking anything long-term, anything at scale, if you're thinking anything where it'll touch the lives of thousands of users, then you would start by saying what are your AI principles? What are the things that you want to put it to work for, and what are the things that you'll not do with AI?
Google was one of the first companies to do that, and it's not just something on the whiteboard. It serves as a guardrail for every single feature that comes out of our stack to our ecosystem. It's a pretty rigorous exercise, but we do it every single time; bringing in customers, partners, our entire ecosystem that is it serving to what we have stood up as our principles, as our framework of responsible AI?
So, I think you have to have that, and then it becomes easy. Then when you think of your business solutions and business outcomes, it dovetails into this framework. Are you being intentional about the environment? Are you be-ing intentional about resource consumptions? Are you being intentional about choosing a cloud provider who sub-scribes to the similar values? Is it energy efficient? What is their story about a green data center? What is their story about giving you AI chips that might be more sustainable for the environment? So, it'll evolve, and I think they com-plement provided you have that intentionality of having those principles and using that in your decision-making pro-cess day in and day out.

Kate Bevan: So, is it appropriate to use AI for all kinds of problems? I'm thinking what the right problems for it are to be solving. What are the things we shouldn't be doing with AI?

Miku Jha: It's a wonderful question, Kate. I would rather start by saying absolutely not every problem is an AI problem. Just not true. In fact, even if it's an AI problem, it may not be a generative AI problem. I think you always want to start with here is the problem and what's the best way to solve it? You have to have a problem centric mindset. And we are not there yet. It's a tool. At the end of the day, might feel magical, but it's still a tool and we are still so fascinated with it that we are trying to find problems to solve.

Kate Bevan: You see a lot of startups at the moment popping up going, "Oh, we can use AI to do this, and we can use AI to do that," and that's the wrong way round, isn't it? That's the tail wagging the dog.

Miku Jha: Yeah, it is the wrong way around, and I think now you can see the cracks when you are in the boardroom today. Now you hear the questions of, "This is cool. It's awesome. What is it solving?" So, I think start from the problem first. In fact, I would strongly suggest that if the problem can be solved using traditional, regular software develop-ment engineering practices, then go for it because this is not magic. It feels magical, but AI takes a lot of effort, en-ergy, resources to get to the point where it feels magical. So, if you can solve it without AI, solve it.
Second thing is that there are certain problems for which it is really good. So, if you look at any kind of a process, your workflow, and you can identify the steps in that where it's repetitive, where you're doing the same thing over and over again, that's a perfect low hanging fruit to offload it to an AI system.
Now, where we are with generative AI is that it's not just good at solving those, but it also has a very strong level of reasoning and intelligence built into it. So, any kind of interactions that you have where you have multi turn going back and forth, it's a great problem statement there. And at the end of the day, one important thing that we want to be thinking about is it's a very powerful assistant or augmentative tool for whoever. Anyone, it doesn't matter what's the profile. Whether you're a marketer, whether you're a sales agent, whether you are a CFO, everyone can benefit from it.
So, the idea is that you as a business want to utilize your best experts and best minds to solve 20% of those com-plexities, which cannot be codified. 80% of it, let the AI do it so that your best minds are utilized on solving the most complex problems, and that collaboration is the best use case for AI systems today.

Kate Bevan: I'm wondering if we are using it almost frivolously. I think I mentioned to you when we spoke before-

Miku Jha: Yeah.

Kate Bevan: ... I've tagged a lot of photos in my personal Google photos, and I also have a lot of old family photos in it, and sud-denly Google photos throw up a photograph of my dad 85 going, "Is this Simon Bevan?" I thought, "Gosh, that's amazing." It was a baby picture of my dad. But I thought, "Are we really devoting these enormous resources, burn-ing the planet, enormous data centers, massive compute, to identifying photos of my dad as a baby?" Are we asking too much of AI?

Miku Jha: But I also think we are asking too much of it because of what it can potentially do. Think about it, Kate. When was the last time that we could interact with one of the most complex systems using a natural language of our choice? And not just interact with it. Actually, makes sense of the output it gives us. You don't have to be a technologist. You don't need to know a programming language. You choose your language, and you make the system do the work for you.
So that level of democratization is at the core of why we are asking so much of AI. But to answer your question in terms of use cases which may be frivolous. I think we are coming around to that because at the end of the day, like I said, these systems are not magical by themselves. So now we are coming around to is there a business use case? Is it delivering value? Is it enriching the life of my customer or user in a meaningful way? We are coming around to it because now that initial euphoria of, "Look what it can do! It can differentiate a cat from a dog." We have gotten over it in some ways, and now we are going to put these systems of work and solutions to solve the right meaningful problems going forward. Just because there's no other way. There's no other way to sustain the effort and the work it takes to push this forward.

Kate Bevan: How do we bridge the gap between the talk and actually delivering on making AI sustainable? Because I really worry about that.

Miku Jha: I think it's the right thing. Every one of us should be worried about it. I also think it's great to talk about it because that's the first level which creates the very much needed awareness that it's not just free for all. When you are using these systems, there is an aspect of sustainability, of impact on the environment, impact on users' privacy and all of that. So, my first thing is we should talk a lot about it.
Second thing is that now how do you translate it into some actionable steps? Just like, Kate, when I did the startup, there was and continues to be so much talk about food waste, but I was like, "Okay, I can't solve the whole prob-lem."
But for me, the big deal was that I was able to show to the investors, to the food industry that this can be looked at differently. You can have a business outcome, but at the same time, you can reduce food waste.
If I go to a business in full transparency and say, "This is going to reduce food waste, but I don't really have any busi-ness metrics for you," it's a very hard conversation to have. But if I tell them that, "I'm going to give you 30% opera-tional efficiencies, increase your sampling size by 10X, reduce your labor cost by 30X. And by the way, we are reduc-ing food waste," that is the conversation which helps things move forward.
So similarly, I think when it comes to how do we think of sustainable AI, you can club it into three very important cru-cial things. One is the infrastructure optimization, because what is the system on which you're running this and is that subscribing to the energy compliance? Is that subscribing to high performance, high sustainability? So that's a very important decision. The heart of it. What is the infrastructure story?
Second is the resource optimization, which goes a little bit into the technical aspect, but the idea is that when you take these models, and you really have to make a decision on do you want to go ahead and retrain the entire model all over again? So, the question becomes do you need that? There might be 10, 15% of cases where it might make sense to do that, but as an industry, where we are at is that with contributions from Google, for example, we are bringing such powerful out-of-the-box foundation models.
And the aspiration there, Kate, is that you only have to do a very small amount of delta work to optimize it to your use case. So, I think at least that should be your first ... "What are the choices I have to take this model and make it work for me, so I'm going to go train the whole thing?" Because it's not just your skill sets are needed. It's not just the cost. It's also the impact.
Then third thing, which is very important is again, to bake in the aspects of sustainability in your responsible AI framework. What is the cost of every inference? How do I measure the footprint? How do I measure the energy consumption?
So if you bring infrastructure, then the resource and then the responsible AI together and make it a practice as op-posed to an idea that no matter what feature you release, it has to go through those checks of safety, of impact, of harmful content categories, all of that, then I think you have something to put into execution. It's not just an idea or a concept at that point.

Kate Bevan: So, it's about taking the individual pieces and thinking about the impact and then putting them together.

Miku Jha: Have a framework, because otherwise it'll just be a great idea. You have not even best practices. Like with Google, we don't put a feature in GA till it goes through our responsible AI check. We don't put a feature in GA till it goes through our AI principles in general. So, you have to have that discipline within the organization as opposed to a nice to have, because the moment you put something is nice to have, it's not going to get done. There's so much to do. There is time to market, there's speed of innovation. All the intentions are there, but it'll not get done unless you're intentional also about sustainability.

Kate Bevan: Right. So, it's about focus as much as anything. I'm going to just move on to the final question. I always ask do you think AI is going to kill us all?

Miku Jha: That's a hard question, but I feel it is overwhelmingly unlikely.

Kate Bevan: Good. That's brilliant.

Miku Jha: That's how I would put it. Because see, one thing is as it stands today, if you think about these large foundation models, they all go through different variations of reinforcement learning human feedback, which means that you're already training the model on, hey, this is harmful. So, you're building that intelligence in the systems as is. There's a lot of progress made in the AI safety research domain.
Our systems, they are quite safe today, but it's only going to get better and better in terms of safety with all the guardrails and the work which is going on. That does not mean that there are no use cases which can have a mali-cious impact or harmful impact, but I think we have to be very focused on risk assessment, go to it at a much differ-ent level of depth, and then start thinking of what is the mitigation for that?
In my mind, even if we took the hypothesis that what you said is possible, it's not that an AI system would be capa-ble of doing it alone.

Kate Bevan: True.

Miku Jha: It can be a whole collection of rules and records and processes and all of that, and your Excel spreadsheet also has a role to play in it, right? But you're not going to say that the spreadsheet is going to do this, but we might say an AI is going to do this, but AI is never going to do this in silo. And first of all, I just don't feel it's anywhere there yet. I know till 2020, I was having a hard time differentiating between a cat and a dog in a meaningful way. So, though we have made a lot of progress-

Kate Bevan: You mean they've come a long way since then?

Miku Jha: It's a topic where we can talk for hours, but I really think it is highly unlikely given all the progress that is being made, not just on models, but also on what is responsible AI? What are the safety practices? What are the guardrails? Regulatory aspects. So, it's all going to come together, but I'm not going to say a 100% the other side.
Also, there'll always be a possibility. We have been dealing with threat detection, we have been dealing with mali-cious use cases forever, even in the traditional software development.

Kate Bevan: Miku Jha, that's really reassuring. Thank you very much for your time. It's been brilliant talking to you.

Miku Jha: Thank you, Kate. Thank you so much. I enjoyed our conversation. Thank you.

Kate Bevan: Thank you. It was brilliant.
The AI Interrogator is an Infosys Knowledge Institute production in collaboration with In Infosys Topaz. Be sure to follow us wherever you get your podcasts and visit us on infosys.com/iki. The podcast was produced by Yulia Debari and Christine Calhoun. Dode Bigley is our audio engineer.
I'm Kate Bevan of the Infosys Knowledge Institute. Keep learning, keep sharing.
About Miku Jha
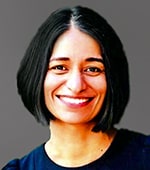
Miku Jha is a director of AI/ML at Google Cloud. She is a visionary senior technology executive with a proven track record of leading large organizations and driving transformative solutions for earth-scale problems. With deep expertise in applied AI, business messaging, ADs infrastructure, IoT, and mobile virtualization, Miku has consistently delivered exceptional results throughout her career.
Currently Miku is leading the AI/ML Center of Excellence at Google, focused on innovating, accelerating and scaling the adoption of Google’s world-class Generative AI solutions for the ecosystem. Prior to joining Google, Miku spearheaded the Applied AI Innovation unit at Meta. Her leadership was instrumental in orchestrating the build-out, launch, and expansion of groundbreaking AI solutions for Meta's products and platforms. She successfully unlocked a new multi-billion-dollar revenue stream by launching and expanding Meta's WhatsApp Cloud API, revolutionizing business messaging.
Miku also contributed to industry-wide advancements through the release of the open-source cross-media ADs measurement framework, "Halo." Miku's entrepreneurial spirit has led to three successful startup exits, highlighting her exceptional talent for turning innovative ideas into commercially successful ventures. As the founder and CEO of AgShift, a globally recognized AI-powered platform for autonomous food quality evaluation, Miku achieved a remarkable 33% reduction in food waste across the supply chain in leading food organizations. She secured a patent for a versatile IoT platform and pioneered the industry's most advanced AI-enabled food quality analyzer, Hydra. This product has significantly improved operational efficiencies and reduced food waste, making a positive impact on the environment.
Throughout her career, Miku has demonstrated her ability to effectively lead large teams and drive innovation. Her leadership roles at IBM and VMware showcased her prowess in managing global business units, leading successful mergers, acquisitions, and integrations. Beyond her professional achievements, Miku is passionate about mentorship, particularly empowering young women in technology. She has served as a technical advisor and advisory board member for multiple startups, guiding and nurturing their growth. Miku's outstanding contributions and leadership have earned her prestigious accolades, including being recognized as a Forbes Next 1000 Honoree in 2021 and as one of the top 10 Women Business Leaders by Mirror Review.
Miku Jha's remarkable journey as a senior technology executive is defined by her ability to tackle earth-scale problems, drive innovation, and deliver exceptional results. Her expertise in applied AI, combined with her entrepreneurial spirit, has enabled her to create groundbreaking solutions and lead organizations to success. Miku's dedication to mentorship, combined with her numerous achievements and accolades, establishes her as a dynamic and influential leader in the technology industry.
- On LinkedIn
Connect with Miku Jha
- “About the Infosys Knowledge Institute”
- “Generative AI Radar” Infosys Knowledge Institute
- AgShift
Mentioned in the podcast