Knowledge Institute Podcasts
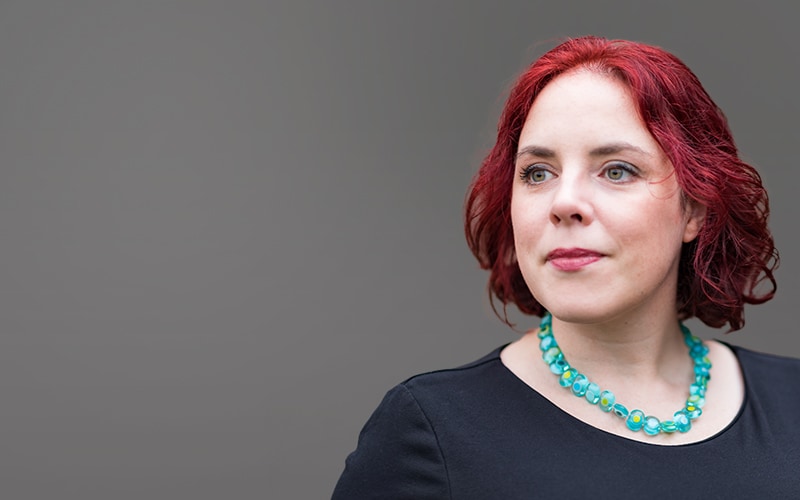
-
AI-powered Biology and Drug Discovery with Dr. Kat Arney
September 23, 2024
Insights
- AI is revolutionizing drug discovery by analyzing vast datasets of chemical compounds and biological interactions, drastically reducing the time it takes to identify potential new treatments. This approach allows pharmaceutical companies to pinpoint promising drug candidates faster, lowering costs and increasing the chances of success in clinical trials.
- By leveraging AI algorithms, researchers can analyze patient data, such as genetic profiles and medical histories, to tailor treatments to individual needs. This personalized approach is reshaping healthcare, enabling more effective and targeted therapies for conditions like cancer, diabetes, and rare genetic disorders.

Kate Bevan: Hello, and welcome to this episode of Infosys' Podcast on Being AI-First: The AI Interrogator. I'm Kate Bevan of the Infosys Knowledge Institute, and my guest today is Dr. Kat Arney. Kat is a polymath. She's a scientist in her own right, she's a public speaker, she's the author of a number of erudite but really accessible books on the science. Those cover everything from the workings of our genes to the working of cancer and how science is tackling it. Also, she's the founder and creative director of First Create the Media, which is a life sciences focused content agency. So yeah, pretty full-on there. Kat, thank you very much for joining us.

Kat Arney: Thank you for having me.

Kate Bevan: Oh, it's a pleasure. It's a pleasure. I'm going to dive straight in because you are across so many AI companies and interesting life sciences companies doing cool things with AI. What's the most exciting or interesting thing you're seen in life sciences with AI at the moment?

Kat Arney: For me, one of the things that excites me the most is the potential for generative AI in biology. I think everyone's got really excited about generative AI technologies like ChatGPT, but I think we forget that these things are large language models. They take large sets of language as a training data set, and then they can predict what you think should come next in a sentence.
Biology is also a language. DNA is a language made of four letters, effectively they're four chemicals, but we refer to them as letters. You can think of them as say the letters of a recipe in a cookery book. They spell out the equivalent instructions. Then also proteins, which the things that the recipes make are made up of 20 letters, effectively 20 different amino acids. From that, we construct all the things in biology effectively.
Using the large language model approach that you can use to say give a prompt to ChatGPT and say, "Write me a poem in the style of Shakespeare about a dog," you can say to a large language model based on biology, "Okay, write me the sequence of a protein that should attack something on a cancer cell." For example, one of the companies we're working with called Etcembly, they're doing this for a type of molecule called a T cell receptor. These are found normally in your body. They recognize things on aberrant cells, abnormal cells like cancer cells, and then tell the immune system to destroy them. We make these all the time in our body, but what if we could use those therapeutically to target the immune system against cancer? So what they're doing is using effectively the same thing like ChatGPT to speak only like ChatTCR to spool out loads and loads of ideas for what these things should look like.
What makes a good one, thinking about the structure of it, the sequence of it, what does it bind to, what's a good target? Then, and this is the important bit, they take them, they make them in the lab. None of this stuff is real until you actually make it and test it. Then they can put that information back into the model and iterate on that to develop new ones. They've managed to develop a TCR-based therapeutic, their first therapeutic, that's really high affinity. So it's high affinity as you'd need to make a medicine, and they've done it in 11 months when normally it would take two years to do this through kind of old conventional methods of screening and testing. That's just their first one.
I think that these kinds of technologies for writing new ideas in biology, new proteins that have never been seen in biology, for using generative AI to come up with creative ideas for DNA sequences, for protein sequences, that for me I think is just super, super, super exciting. But like I said, they've all got to be tested. They've got to be shown in the lab that you can make them, that they work, and then in humans that they are effective and safe.

Kate Bevan: Where are we at with that at the moment? I saw the news story about the new therapy that was just basically making melanoma go away. Is that part of this technology?

Kat Arney: There's lots of applications for AI and machine learning, the boring, but actually more useful and widely-used cousin of AI in biology. We are starting to see the first generation of AI machine learning design, drugs starting to come into clinical trials. That's a big question about whether these things are actually any better than doing it the hard way, doing it through sensible design and testing, all these kinds of things.
I think there's a lot of excitement around some of the novel, we call them modalities, like types of treatments. So there's been a lot about mRNA vaccines. They hit the headlines in the COVID pandemic and then how can we use this kind of technology, which basically it's a training module for your body. It tells your body what it should be looking for and guarding against or attacking. So using AI, using machine learning to kind of think about how to design these sorts of things, I think is potentially really exciting as well.
Then you couple that with the advances that we've seen in manufacturing and just basic protein engineering, all this kind of stuff, this fusion of better techniques, better technology. Adding generative AI into the mix I think is just, it's a really, really, really exciting time, but it will have to result in better therapies because that's the output that we need. But huge potential because there are so many diseases where we don't have even good treatments, let alone cures. Cancer's the big one because it's incredibly common, there's big money there. But many, many, many diseases where we really need some, desperately need some innovation.

Kate Bevan: Where else could this be useful? I'm thinking of motor neuron disease, which of course has been in the news in the UK recently with the death of Rob Burrow, who's the rugby player? Could it be useful for that kind of disease?

Kat Arney: Yeah, and there's many applications. The generative AI stuff I talked about, that's exciting for trying to write new ideas in biology. Some of the more conventional applications are just using AI to crunch through the data that we have. Pharmaceutical companies have huge data sets that are really barely scratched the surface of them. The same with massive public data sets, the data sets that come out of research, big studies, small studies. We worked for a while with Health Data Research UK that are doing a lot of work to try and curate these data sets, clean them up, make sure they're good quality, make sure we know what's in them so that people can access them, and then run machine learning models on them. Lots of issues about how you do that safely if you're doing it with data. Lots of stuff about, I don't know if you've talked on the podcast about things like trusted research environments where you keep all the data in one place and then you let people come in and play with it and then they have to go away.
It's making all this incredible data that does hold the keys to so many treatments, making that open and explorable, but while also keeping it safe and secure and enabling people to do things with data in a very, very safe and secure way. I think now we've actually got the technology to do that. I mean, God, people used to just send round spreadsheets of patient data. This is bad.
Then you have exciting things where you get into the world of knowledge graphs, and this is an area that my partner's company, Ontoforce, they work at this how do you get all these data sets and kind of smush them together and get insights out of that about what targets should we be going for in disease. This speaks to things like motor neuron and things like multiple sclerosis where we don't even really have good drug targets. We don't know what's going on in these diseases. Are there actually different cohorts of patients that we would need to be treating in different ways? I think the answers to all of that are in data sets that we have, maybe in some data sets we don't have yet. But then using these kinds of AI machine learning techniques to smush through all the data, crunch through all the data and get these insights.

Kate Bevan: Given the potential we've got a lot of this data anyway, what is the next step? Is it to bring it together? Is it to communicate the need to do this? Is it to get it into labs? Is it to get it out to research? What's the next step in turning this into treatments?

Kat Arney: Well, I mean there's so much going on already. I go to an annual conference that's organized by the Bio Industry Association called TechBio, and there there's just so many companies that are using public data sets, private proprietary data sets to try and find novel insights and tackling really exciting questions in biology and then designing therapeutics for them. So I think that there's really like this flywheel is starting to turn, and the point about machine learning and AI is that it's iterative. You do something, you find out, you put the data back in and then your model learns and optimizes. So I think we're starting to see the turning of that flywheel.
I think it's somewhere where the UK really, really excels, and I would really like to see whoever's in government next taking this very seriously as an incredible engine of the UK economy. Our NHS is a phenomenal source of health data. We have a National Health Service, we have national health data. We have an incredible asset in the NHS and trying to sort out the current suboptimal way that we organize clinical data and health data and the stories that the public are told about ooh, evil pharma's rummaging through your data, what this data actually can do and can tell us and how we can use it responsibly. I think that's a very, very important communications challenge, and it's an important scientific and technical challenge.

Kate Bevan: Yeah, I'm going to come to you now with your hat as a science communicator on how do we tell those stories? How do we get away from the narrative of bad big pharma selling off your data, privacy issues? How do we communicate that so that people understand A, what an enormous opportunity this is, and B, why we should be up for sharing our data in this way?

Kat Arney: Yeah, and I think it ultimately is important to remember that it is every individual's own choice what happens to their data, what happens to their body. It's everyone's choice. I think it's important that we do get these narratives right. The work that we did with Health Data Research UK, it was about how do we tell the stories about the positive impacts of using health data, how it is done safely, who does get access to it, what does that mean. A lot of people do understand that if you want to get medicines, the way that we get medicines in a capitalist economy is that there has to be a huge amount of R&D done. We have to get the data from somewhere and that pharma companies have the assets and the leverage and the investment to do that.
I think what's important is that we really talk about the outputs of it and also that we need to demonstrably see that there are improvements. I think we forget the incredible improvements that have come in things like cancer treatment and treatments for so many diseases are telling the story of the positive stories that are coming from the use of health data. So I think that's going to be really important. We are still at the early stages, so finding really good success stories is quite hard, but I think it is important to be really alert for those stories and also accept that there are other narratives, and we do need to recognize what people are worried about. People have legitimate worries.

Kate Bevan: Your other hat is that you're a content professional. So thinking like that, how do you think AI fits into content and science communication?

Kat Arney: This is a really interesting one. About a year, 18 months ago when things like ChatGPT really came on the scene, the agency world ... I run a science communication agency, I'm part of agency world, everyone just panicked because they were like, "Oh my god. AI is the thing that's going to take all our jobs. Oh no, anyone who's a writer, you are going to be made redundant in five years. Oh, no." Panic, panic, panic. I sort of sat and I thought ... Luckily I live with an AI professional, so we could talk about this. I have lots of friends who work in these fields, and I think that it is important to see AI content generation as a tool, but to be honest, it needs to get an awful lot better.
I'm not sure that it will achieve what we actually need to achieve from content. Because the more I think about it, things like ChatGPT and stuff like that, they're very good at listing what. They can list facts. They're not always accurate, they do hallucinate. But what they can't really provide is context, is why, is insights and insights that aren't in the public domain that companies may want to put out. So we're working with all these companies trying to talk about their ideas, their proprietary technology, the advantages of that. None of this stuff is in the public domain yet because we're announcing it. We're talking about it.
I did see an AI science content marketing company, and I looked at their website, and they had some blog posts about this is how you should do content marketing. Every single one of them, clearly written by AI, just banal. Nothing that I didn't know already because all this stuff is generated from things that everyone knows already because they're all out in the public domain already. Nothing specific, nothing insightful, nothing relevant to them, nothing really of any value. So I think that certainly from my perspective as someone who really prides themselves on finding the story, finding the hook, finding the things that's interesting and true and human and insightful and different about the work that our clients do and then communicating that in a compelling way, I think my job's safe for a bit.

Kate Bevan: I keep thinking about more and more as I see how generative AI is being used in content and in business, actually because I talk to a lot of people who use AI, AI tools in business is actually what's going to be really useful is the small models, the in-house models, the on-prem models, which are drawing on an in-house body of knowledge or proprietary knowledge rather than just the enormous public tools like ChatGPT, like Bard, like all of those that just pull in any old thing from anywhere. Are you seeing that as well?

Kat Arney: Yeah, exactly. Because you build, if you work with a client or if you work in your own organization, you build an internal knowledge base. I used to work at Cancer Research UK in science comms for many years, and I was like, "I wish that we could have got those tools trained on all the things we know," because I'm going back and reading annual reports from 1902. Can we digitize those? They were digitized at one point, but how can we make sense out of them and insights. Certainly we use AI tools. We use a tool called Descript. We make recordings of our workshops and things like that. You pop it in Descript and say, "Give us a summary of this." Amazing. Absolutely incredible. The transcription's really accurate. You can make things like little audiograms and videograms really easily. We use some AI tools for just generating puns and ideas and, "Okay, we're writing an article about this. Have we missed anything important?" Just that sort of sense check. But we would never just rely on an external AI to write content for us because it's just pablum basically.

Kate Bevan: It is. And also the problem with uploading your own stuff into something like ChatGPT is you're putting it out into the public domain, and you can't do that with proprietary information.

Kat Arney: Exactly. Every AI based software we use, we're like checking the box going, "Nope, nope. You do not take this. You do not do anything with this." This is private client information. I think the companies that get that and where the value is in enabling companies or agencies like ours to really generate insights from the proprietary stuff we have, I think is going to be very useful.

Kate Bevan: Yeah, that's where I see it too. I'm going to come to my final question now. It's one I ask everybody, and I know you've got some interesting thoughts on that. Do you think AI is going to kill us?

Kat Arney: Ooh. Well, I don't think AI per se is going to kill us, but the thing that I really, really worry about is the risk of the degradation of our knowledge base. Particularly that concerns me is the degradation of our knowledge base with regards to science and particularly health science. Now, what we're seeing is that a lot of these AIs are trained on the corpus of scientific knowledge, the scientific literature. I can tell you that a lot of the scientific literature is not up to much. There are things in there that are irreproducible, there are things in there that are flat out fraudulent, there are things in there that are plagiarized, that are just wrong. There's all sorts of stuff in the scientific literature, and it has got much worse recently. So the corpus of scientific literature already is contaminated, and now AIs are being trained on this corpus as a training set.
We're already starting to see AI generated papers. There was a fantastic example that did the rounds on Twitter, and it had a picture of a mouse with kind of enormous genitalia, enormous gonads, and it was talking about stem cells and things, and it was laughable. But you're like, someone has put together this paper, this is now going back into the scientific knowledge base because the checks and balances for a lot of this are just broken. People are publishing all sorts of nonsense, and then the AI is being trained on that again. I'm concerned as well when you feed that back into things like education. So what are students learning? Are people using ChatGPT basically as a search engine when it can still hallucinate? Google's now using these things as a search engine where it's hallucinating, it's not accurate.
So the thing that I really worry about that could do real harm is just unmooring to empirical reality from what is conceived to be truth and knowledge on the internet. Where are we gate keeping what is actually correct? And there's some like the big stuff like the second law of thermodynamics, and then just really small stuff like, okay, this niche signaling pathway in a cell. But if you're a pharma company that thinks that that niche signaling pathway is important in a disease and you're developing a drug against it, if that's not actually true, you are in big trouble because either you'll spend millions and millions of pounds developing a drug that then turns out not to work, or you'll get through and into trials and it will not be safe, it will not be effective. These are serious risks, and we miss out on treatments that could be useful. We lose sight of what is actually empirically real in science. So for me, that's the fancy way of saying I am genuinely concerned about that.

Kate Bevan: I think we'll have to leave it there. Dr. Kat Arney, thank you very much for joining me.

Kat Arney: Kate, it's been a pleasure.

Kate Bevan: The AI interrogator is an Infosys Knowledge Institute production in collaboration with Infosys Topaz. Be sure to follow us wherever you get your podcasts and visit us on infosys.com/iki. The podcast was produced by Yulia De Bari and Christine Calhoun. Dode Bigley is our audio engineer. I'm Kate Bevan of the Infosys Knowledge Institute. Keep learning, keep sharing.
About Kat Arney
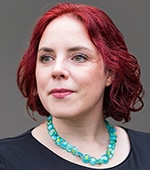
Kat Arney is a multi-award-winning science writer, public speaker and broadcaster, and the founder and Chief Creative Officer of First Create the Media - a communications strategy and content agency specializing in the life sciences. Following a degree and PhD at Cambridge University, Kat spent 12 years in the science communications team at Cancer Research UK, co-founding the charity’s ground-breaking Science Blog and acting as a principal media spokesperson. Kat is the author of three books, Herding Hemingway’s Cats: Understanding how our genes work, How to Code a Human and Rebel Cell: cancer, evolution and the science of life. She has fronted numerous radio shows and podcasts, including Genetics Unzipped and The Suffrage Science podcast: How women are changing science, the series Ingenious on BBC Radio 4, telling stories about our genes, and Bug in the System, a three-part flagship documentary series about the past, present and future of cancer.
- On LinkedIn
About Kate Bevan
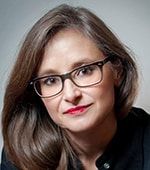
Kate is a senior editor with the Infosys Knowledge Institute and the host of the AI Interrogator podcast. This is a series of interviews with AI practitioners across industry, academia and journalism that seeks to have enlightening, engaging and provocative conversations about how we use AI in enterprise and across our lives. Kate also works with her IKI colleagues to elevate our storytelling and understand and communicate the big themes of business technology.
Kate is an experienced and respected senior technology journalist based in London. Over the course of her career, she has worked for leading UK publications including the Financial Times, the Guardian, the Daily Telegraph, and the Sunday Telegraph, among others. She is also a well-known commentator who appears regularly on UK and international radio and TV programmes to discuss and explain technology news and trends.
- On LinkedIn
- “About the Infosys Knowledge Institute”
- “Generative AI Radar” Infosys Knowledge Institute
- First Create the Media
- TechBio UK 2024
- Etcembly
- Ontoforce
Mentioned in the podcast