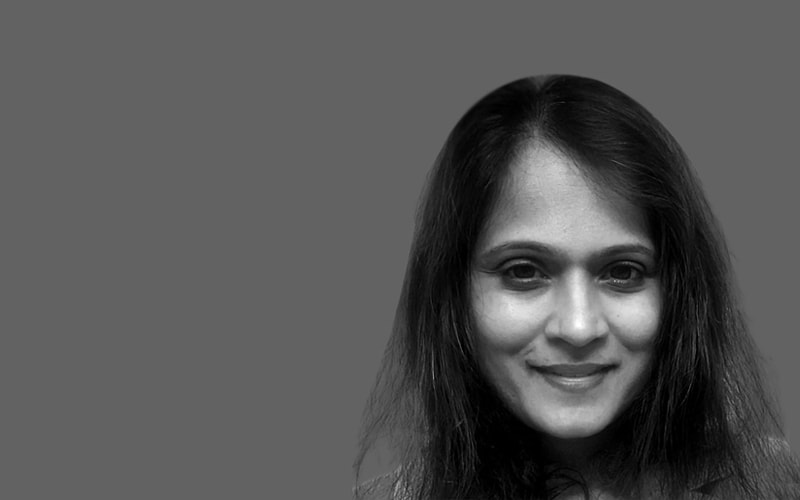
-
AI Interrogator: Evolution and Responsible Governance in AI with Rajeshwari Ganesan
November 6, 2023
-
Ethical AI is an urgent and hot topic as we try to stay ahead of this fast-evolving technology. Rajeshwari Ganesan, one of Infosys’s leading experts on machine learning and AI, joins host Kate Bevan of the Infosys Knowledge Institute to discuss the importance of human oversight and governance in building responsible AI.
Insights
- AI is evolving from transparent and easily interpretable transparent models to black box models that rely on large amounts of data and intricate patterns that are hard for humans to understand. This move has raised concerns about the interpretability and accountability of some AI systems and outputs.
- Human involvement and governance remains critical in validating and interpreting AI outputs and addressing ethical and societal concerns. AI may automate some tasks, but there will always be the need for humans to regulate, interpret, and make important decisions in the context of AI systems.
"By listening to this podcast, you hereby acknowledge and understand that your personal data may be processed in the manner described in our Privacy Statement"

Kate: Hello and welcome to Infosys Knowledge Institute's AI podcast, the AI Interrogator. I'm Kate Bevan, a senior editor with the Infosys Knowledge Institute. And my guest today is Raji Ganesan who is a distinguished tech-nologist from Machine Learning and AI at Infosys. Actually one of the coolest people I've ever spoken to about AI, so Raji, I'm absolutely delighted to have you. Thank you for joining us.

Rajeshwari: Pleasure is mine, Kate.

Kate: Raji, when we spoke about this before, you made a really interesting distinction between us being both builders and consumers of AI. And I wanted to dig into that a bit. And then to come to thinking about the control we have over AI. Do we have enough control and do we have the right kind of control over it?

Rajeshwari: Kate, that's a great question, and I wanted to take a step back and talk about AI, when we look at it, we have the data, we have the algorithms or the models, and then we have the prediction.
We were using a lot of math statistics, we were building systems, for example, which would say when you call a call center, you can ask, "Okay, this is your approximate waiting time." Or the way the seats are allo-cated to you in an aircraft. It could even be the recommendations you get on a retail store, what product you may be interested.
So here the models were very transparent. A lot of data you fed into the system, the model and the output was very, very predictable. But going ahead, this was the onset of neural networks and deep learning.
The interesting fact, Kate, was the data was more important than the models. We had to feed these systems with lots and lots of data, and the algorithms would actually find the innate underlying patterns, which was often difficult for humans to figure out.
For example, the most interesting case, which I've been fascinated with was, when lots of data was fed into the systems, they were able to find the gene expression related to triple-negative breast cancer, right? Which physicians did not know to begin with.

Kate: Oh, really? That's really important and interesting.

Rajeshwari: So while this was an advantage, that the systems could figure out patterns which subject matters expert could not, but it was a double-edged sword. On one hand it was finding patterns, but how do you know these patterns are right or wrong?
So this actually led to the situation wherein these models were black box. We were fearing these models be-cause the data which goes in is the data from the society, and we know that humans by nature innately have these biases.
So whether it came to loan delinquencies or whose resumes were picked up by these systems, these are few things which made a headline. There was always this concern that these black box models... We had to have better explainability.
Now, coming to generative AI, it's become even more complex because the data is not yours. The model is not yours. You're just consumers of AI, a lot of times. People talk about hallucinations. That is one form of not knowing the consequences.
Despite not owning the data, despite not having the models, when we consume these models, how do we still build responsible AI systems? And that is the main thing, Kate. With respect to this, this is my thought process on this.

Kate: Generative AI makes us consumers of AI. So knowing therefore that we've got a sort of two-pronged relation-ship with AI, how then do we build a responsible AI system? We talk a lot about "Responsible by design.” What does that actually mean and what are the outcomes?

Rajeshwari: It's very interesting, I think. "Responsible by design" is something like if you look back when we are building non-functional requirements like security or performance, we always said, "Security by design."

Kate: Anybody who knows about cybersecurity, you'll be nodding along going, "Oh yes, security by design, we know about that."

Rajeshwari: Right. So the whole idea was security or system performance or other aspects of system should not be enough. The thought, "We need to begin early and we need to engineer this in the lifecycle state." So I think responsible AI by design possibly is that kind of thinking process that could make responsible AI which has multiple dimensions. It may be safety, it may be reliability, it may be fairness, ethics, it doesn't matter, right? We can composite in one word called Responsible AI.
Now, when it comes to responsible AI in the design stage, we need to think of methods. For example, retriev-al-augmented methods. Or aspects of being able to fine tune these models for your domain at the design stage. When it comes to programming, it's about having external control of these models.
So if these models are going to hallucinate, it's an example like this, right? Let's say I have a person who has lost his memory. You can't say, "Have more willpower, and make sure you don't get lost." It's not possible. You need to have an external method wherein you can give that kind of support that if the person loses their way, you are able to bring them back.
Q2, I think from a programming perspective, we do need to have these external controls or frameworks, which can flag when there are hallucinations and ensure the systems are back on track.

Kate: What kind of frameworks are we talking about? I mean, I presume we're talking about human input. Are we talking about having a wide range of stakeholders? Or are we talking more about computer science approach and guardrails here?

Rajeshwari: So in the second part, which I'm talking about the programming, it's purely software engineering frame-works, Kate. But I'm not saying that's the only thing. One part of it is to look at, as you said the software en-gineering lifecycle stages.
So we spoke about design, we spoke about the programming, the third is verification and validation stage. This is where Kate, I think we also spoke about the fact that it's impossible to have testing, traditional testing on these models. You can just benchmark and say, "Hey, this is fine." Because it's not so simple.

Kate: Yes. You can't benchmark for what one person thinks is fair and what one person thinks isn't fair. Can you?

Rajeshwari: Right. So this is where we need to have other methods like Socratic AI, which we spoke about, right? Wherein you do have methods wherein multiple of these elements are in conversation to reduce these hallucinations when the system is up and running.

Kate: Do you want to explain the Socratic method for the people who haven't heard of it before? Because you had to explain it to me. Because I hadn't heard of it before.

Rajeshwari: So Socratic AI is a very interesting method which was proposed by Princeton. One of the things the hypothesis says is by question and answering. Let's say the beautiful example which was taken was when Socrates had this little boy who is five years of age who didn't have a formal education in mathematics. And he asked them the simple question that, "If you have a square which is of dimension two by two, can you double it?"
The boy instantly says, "No. Each side I will double by two." And Socrates says, "No. If you do that, you would-n't be doubling, but you would be doing more than that."
So with a series of conversation, Socrates asked this little boy without formal education in math, but the boy is able to come up with the right answer.

Kate: So it's guiding the AI to come up with the right answer? Is that a good way of explaining it?

Rajeshwari: Yes, yes. The other simple example, Kate, is if I ask the question that when I was ten years of age, my sister was half my age, how old is Priya? The answer of the machine says, "Priya is 35 years of age." Where did I ever say Priya is my sister?

Kate: Yes, exactly. It's a bit of a brain bender, isn't it, sometimes?

Rajeshwari: Yeah. So when I asked the question, did I mention, "Priya is my sister?" It goes back and say, "Well, I'm sorry, Priya, I never mentioned Priya is your sister. Your sister is 35 years of age."
So things of this nature could be in the mathematical reasoning perspective. So today, when we look at the spectrum of some of these newer technologies, they have knowledge, they have reasoning. When we say rea-soning, it's mathematical reasoning, Right? It could be spatial reasoning.
So those kinds of questions, the machines are very weak, but they write the answer in such beautiful English one tends to get confused that they're actually producing right out. But so I think that's the second part.
The third part, which is the verification and validation.
And the fourth part is really the deployment and things really, how can it hand it off to humans or human judgment? So these four things, Kate, is more on the software engineering, but I think there is another big dimension which you indicated, which is about governance.

Kate: Governance and human input, and lots of different stakeholders.

Rajeshwari: Having human input. I mean, let's take a very simple example. Think about a supply chain problem and ma-chines taking decisions on who is the supplier they have to be chosen or what routes has to be done. Let's suppose that we decide to have a strategy of just-in-time supply chain, which means last-minute planning can lead to a lot of suboptimal route, and you may have carbon footprint. Or you may not be giving the task or job to suppliers over smaller businesses. So those are the times when what you think is purely technical issues could actually manifest to taking the responsible AI dimensions, right? Of environment and damage. It could be of being fair to all kinds of suppliers. Maybe it's a minority, you want women business, we don't know. That's where the governance, I think also plays a big role, Kate.

Kate: One thing I actually want to dig into a bit with you is also, we use words that impart human understanding. It might anthropomorphize AI when we talk about reasoning and hallucination, those are things that humans do. Do you think that's overstating it or do you think that's a metaphor that helps people understand what it's doing?

Rajeshwari: People look at certain dimensions of AI, especially as consumers of AI. They're very fascinated by the knowledge aspects of AI.
So today, if I say, give me a proposal to submit to my customer in this area, it produces a beautiful narration. But if I ask the spatial questions saying, "I'm moving upwards, north in this direction, coming back south, tell me how far I am." It will prepare some grand theory of pythagorus theorem, which is just not relevant.
So this is where I think users of AI have to be very much aware that it's the beautiful English eloquence, but doesn't mean it's correct. So adding this, hallucinations that way are important, and we shouldn't get con-fused.

Kate: With AI, it looks as though we do need humans involved still. Is that a threat for jobs then? Or are we going to be replacing jobs, creating different jobs or creating worse jobs?

Rajeshwari: That's a very difficult question, but I think the future of workforce is not going to look the same way as it is today. A lot of it is the low-fungible jobs, which is going to be sort of substituted by AI, and they would be jobs, new jobs, which would be created.
But I think humans today would be on top of matters from a regulation, both from a government and societal regulations, Kate.

Kate: I saw recently a piece, it was on Twitter, and it was about London's railway stations. I don't know if you know London, but we have lots of railway stations and it's kind of specialist knowledge where you can get to from which station. And it said in there that the Harry Potter platform was at, I think Victoria Station, which it's not. All of Twitter was going, "Come on, it's King Cross."
Is this something that the AI's written and nobody's checked it? And it's the checking it, isn't it? Which for me actually sounds like a more skillful job. So I'm sort of hoping that there'll be more skillful jobs and interesting jobs rather than necessarily just supervising robots all day.

Rajeshwari: And at the speed at which AI gives a response, it's impossible to have human in the loop at every step. So I think we have to reimagine what we should be doing. How can humans be there? What are the conditions when they can hand off to humans. We need to think through those fundamental design decisions.

Kate: I'm sort of thinking of the promises of the '60s and '70s. When we were thinking about The Jetsons and how we were going to have a new age of leisure with all the robots doing it for us. I'm still waiting for it like 50 years later.
I'm going to finish off with one question, which thinking about humans and Ai, is the AI going to kill us all, do you think?

Rajeshwari: Well, I seriously think we are far away from that situation. Possibly it's the climate change that we need to be worried much more than AI killing us today. Even with the generative AI, we are struggling with a lot of is-sues, the very basics.
So I think it's a long time before, at least in my perspective, that AI is going to kill us. We are going to have other serious issues which will be in the forefront of humanity, which we need to tackle and solve, not AI.

Kate: That's very reassuring. Thank you, Raji. That's absolutely brilliant.

Rajeshwari: Thank you so much, Kate.

Kate: That was part of the Infosys Knowledge Institute's AI podcast series, the AI Interrogator.
Be sure to follow us wherever you get your podcasts and visit us on infosys.com/iki.
The podcast was produced by Catherine Burdette and Christine Calhoun. I'm Kate Bevan of the Infosys Knowledge Institute. Keep learning, keep sharing.
About Rajeshwari Ganesan
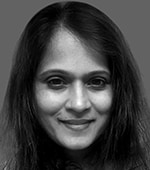
Rajeshwari Ganesan is a Distinguished Technologist in ML & AI and the Field CTO based in Seattle, with 25 years of experience in the IT industry. Highly regarded for her expertise in AI, Software Resiliency, Product Engineering, and Cloud dependability, Rajeshwari works closely with CXO client leaders globally, advising on innovation and transformation. With 14 US patents, 30 international technical publications, and multiple Innovator of the Year awards, she is recognized for exceptional contributions. Rajeshwari is a part of the CTO Office at Infosys, led by Rafee Tarafdar.
Connect with Rajeshwari Ganesan
- On LinkedIn
Mentioned in the podcast
- “About the Infosys Knowledge Institute” Infosys Knowledge Institute
- "The Socratic Method for Self-Discovery in Large Language Models" Princeton NLP, May 5, 2023