Knowledge Institute Podcasts
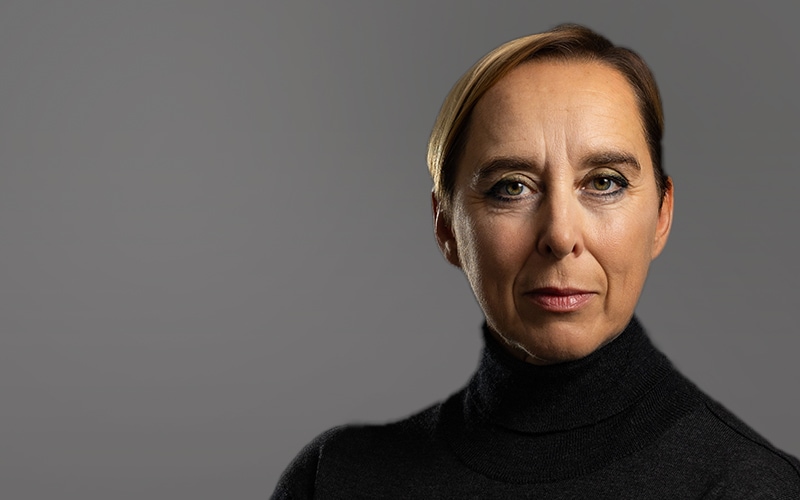
-
AI Interrogator: AI’s Data Dilemma with Timandra Harkness
January 08, 2024
Insights
- Transparency in AI systems is crucial, both in terms of their operations and the biases they may contain. Transparency is seen as a critical factor in building trust and allowing individuals to assess the overall level of trustworthiness in AI applications.
- Privacy concerns and potential misuse of data are highlighted through examples like Strava's cycling data and the New York City Taxi Data Set. There is a need for responsible data usage, considering the ethical implications and potential consequences for individuals if their data is repurposed without consent.

Kate Bevan: Hello and welcome to this episode with the Infosys Knowledge Institute's podcast on all things AI, The AI Interrogator.
I'm Kate Bevan, and my guest today is Timandra Harkness, who's a broadcaster and the author of the book, "Big Data: Does Size Matter?"
I'm really delighted to have you here because it's such an important underlying point for the whole of AI. Is the data that underpins AI good enough? What is that data? Where does it come from?

Timandra Harkness: Okay, that's a very broad question, and if I was going to be really picky, I would say, "Good enough for what?" Because I think that's a key question. The question where it comes from is one of the really great things about what they used to call big data and is now just data is that you can recycle data, if you like. You can use data that was collected for one purpose or even that was just a byproduct of people doing stuff that we do because we do everything digitally now and say, "Okay, well now we can mine this data," as they say, for information about something else. And that of course has opened up so much potential for us-ing it for different purposes, but that data that was collected for one purpose isn't necessarily good enough for another purpose.

Kate Bevan: We don't have the rights to use it. I mean, that's the other question, isn't it?

Timandra Harkness: Yes, there is that, yeah, somebody's given their consent to their data being used for one purpose, they find it reused for something else.
Actually, one of my favorite examples of this is the app called Strava, which is used by a lot of very sporty people who do running and my brother and his partner who run marathons and half-marathons, they use it to time their runs so they can say, "Oh look, this was my maximum speed on this bit," and then they share it with their friends. It's all very competitive and this is great.
So Strava realized at one point that they had lots of data from people cycling in cities so they could build a heat map of where people cycle most, and then the city planners went, "Well, this is wonderful. We want to build more cycle infrastructure. We want to see where people are cycling. We'll put the cycle lanes there."
Then of course, I mean, thankfully the people at Strava were smart enough to say, "Well, yes, but we don't capture every single cycle journey made by everybody. We only capture cycle journeys done by people who use Strava, who by definition tend to be more sporty and more confident. They may not be the people that you're building cycle lanes for. So don't take our heat maps of where confident Strava users cycle now and take that as complete data set about where people might cycle if you built cycle lanes."
So, where we think in terms of good enough, is it good enough for what? And is it a good enough match for your purposes, or was it collected for something so different that actually it would mislead you if you used it to train your machine learning model for a completely different purpose?

Kate Bevan: I'm also thinking actually along those lines of the time Uber released... I can't remember when it was, it's a few years ago, they released all data showing that X number of New Yorkers were doing a walk of shame on Sunday mornings of coming home from nights out. So that makes me think the data sets we are building and relying on are also putting people's privacy at risk, right?

Timandra Harkness: Yes. I think this is a real problem that when you get enough data, even when it's anonymous, it doesn't real-ly take much of it, that you could put it together and identify an individual. And even if you don't identify individuals, you can identify patterns of behavior that might pick out certain types of people. There's a really lovely data set, people like playing the data sets called the New York City Taxi Data Set, which is like the records of all the New York City taxi meters.
So, it shows its location time, but also fares. So, you could go into the data set now and pick out an individ-ual taxi in the past, not live, and see where they picked up and where they went and where they dropped off and what fare they got. Somebody calculated whether the characters from Die Hard 3, I think it was, could have got from the tube station in Manhattan to the Upper East Side in half an hour as happened in the film, whether this was possible, because in the film they didn’t hail a taxi, they did drive it and so they got all the journeys at roughly that time and weekday that actually were done and worked out how many of them happened within half an hour, and I think about half of them did.
So, they said, "Actually, yeah, you have a 50/50 chance," but perhaps more worryingly, if you could follow a taxi driver's regular habits, then you could work out perhaps this taxi driver never works between sunset Friday and sunset Saturday, or perhaps this taxi driver regularly stops five times a day at the Muslim prayer times. So, you could actually deduce very personal information about people from a data set that had been made public because taxi meters are a public information source. So, there is always that tension between using information for a new purpose that might let you do wonderful things, but also, as you said earlier, if somebody hasn't consented for their data to be reused for those purposes, it could have consequences for them that they wouldn't have chosen.

Kate Bevan: So how do we make ordinary members of the public, like you and me, but also the tech people who want to build things who maybe don't think about the implications of using this data, how do we make people more alert to that? And, I suppose, how do we make people more willing to share data because more data should, in theory, build better models, right?

Timandra Harkness: Well, yeah. I mean, I think those two are connected because I'm a great believer in Onora O'Neill the philos-opher. So, when somebody asked us something about trust and how can you build trust in the public of... I don't think it was data particularly, I think it was something else, and she said, "Well, we shouldn't worry about getting people to trust something. We should worry about becoming trustworthy." Because if a sys-tem is trustworthy, then people should trust it, but otherwise perhaps they've got good reason not to trust it. Why should people go, "Yes, use my data for absolutely everything," if they've got no way of knowing whether their interests and the interests of the people using the data are compatible? I think there's a lot in that to try and build systems that are transparent, not just in terms of how they work.
So, think about health data for example. There's no doubt that in the UK, especially health records are a massively valuable resource for research because it's a very complete database of the progress through the years of the entire population, pretty much. So, the potential for research about long-term impacts of things, about efficacy of different treatments is great purely for research and better human health.
That also means, of course, that it's financially valuable, which in the UK, because the system is government funded, that could also be a really good thing because puts more money into a system that's not really got enough resources, and when you tell people, "Well, we'd like to have access to your health data in order to improve research for future people, their health conditions, and also to put more resources into the NHS," people tend to be very willing to do that. People have a sense of altruism. They say, "I understand that there's a risk that my own personal privacy could be intruded upon," because again, it's quite easy to re-verse engineer and find out who somebody is, but most people actually are quite willing to take that risk if they believe in the purposes for which it's going to be used.
But if you said, "Well, we'd like to use your health data so that we can make sure we allocate resources to people who have lived a healthy lifestyle and not to other people," for example, then I think most people would say, "Well, no, I don't think I agree with that. I don't necessarily want you to be turning away smokers and overweight people from health treatments." So, I think transparency about purposes is something that would make a big difference.

Kate Bevan: I think the transparency is a real challenge as well anyway, isn't it? You say that you can reverse engineer people whose data makes up part of that data set, but transparency should also include how these algo-rithms are built, understanding of theirs bias in them. So, there's a real tell-us error around transparency.

Timandra Harkness: Oh, definitely, and that is where I think it's not reasonable to expect every individual to really understand how it all works, but if we are explicit about the assumptions in our models and the purposes of our models and the limitations of the datasets, then people can at least make a judgment about the overall level of trust. I mean, it's like you get an aeroplane, right?
Most of us do not have a detailed knowledge of aircraft engineering, but we have an understanding of things like that The aircraft industry is very safe, that it has an exemplary system for identifying past prob-lems and what caused them and preventing them happening again. And so, we have an overall level of trust that it's going to be as safe as it can possibly be. Now, it would be wonderful, wouldn't it? If we had that in the tech industry, it would be fantastic.

Kate Bevan: When we've got the data, how do you get the best out of it? I mean, we always saying, "Oh, we need data scientists for that." Who are these data scientists? How do they get the best out of that data?

Timandra Harkness: It's a very new job title, and I suspect it covers people with a very wide range of backgrounds. I mean, that would be calling every everybody who works in the aircraft industry, an aircraft engineer, like, "Well, okay, you probably could do that," but it probably doesn't tell you very much. Is this person on the ground or a spanner, or are they looking at a big computer designing asset workflow systems?
I guess handling data you could say is a skill in itself. Now, I'm a little biased. I kind of have slid in really as a statistician sort of, I'm actually talking to you in the Royal Statistical Society as it happens, and I think there could be a lot more cross over there because if you like, statistics is the kind of underlying theory of data and how you use data, many of the rules of which come from before the machines to do it, but most of the principles still apply.
I mean, a lot of the things that we do very usefully now with data that no human could manage in their brain. Things like clustering, things like finding, if you like, natural clusters of data points that have things in common, and then letting the computer tell us what it is they have in common so that we can treat them as a kind of population. That actually comes from a quite basic statistical methods that predate the comput-ers, but we just use it in a more sophisticated way.
And I think that that kind of attitude that it's not magic, you don't just design a really whizzy computer pro-gram and then tip a load of data in as if off a tipper truck, answers magically come out. It's much more like cooking. It's much more like saying, "Okay, right, well, we want a cake, so we need some stuff that's going to bind it together, some stuff that's going to trap air, some stuff that's going to make it taste good. What have we got here? Let's work out what we need, find what we have, and then work out a recipe that's go-ing to get us from A to B," and I think we could do a lot more of that with data.

Kate Bevan: What does that mean exactly for data?

Timandra Harkness: In the sense that if you know what information you want to get out, then you say, "Okay, well what do we need for this?" And then you go looking for the right kind of data and say, "All right, well, what is it we need to do to this data then?" What kind of AI is going to let us get out the answers that we need and how the two good things going to work together? If the data set isn't quite what we'd ideally like, can we tweak the AI program to compensate for that?

Kate Bevan: Or synthetic data.

Timandra Harkness: Or synthetic data, exactly. Can we make something out of the data that we do have that we can then oper-ate on as if it was organic data?

Kate Bevan: I think of synthetic data as a bit like the PCR process for amplifying DNA. You take a little fragment of it and then you run the process and you get much more, and from that you can start inferring things about what strain of Covid you've got or whether this fragment of data can be identified as coming from a victim from a crime, that kind of thing. Is that a fair analogy?

Timandra Harkness: Yeah. I think there are some advantages to synthetic data. Going back to what we were talking about before, which is the danger that you acquire a data set that's quite innocuous from some innocuous purpose like getting a taxi, and then it ends up being attached to some individual or some subpopulation in a way that's problematic. Well, synthetic data of course is a great way to get away from that because you can say, "All right, well, what kind of insight or what kind of results could we get from the original data set?" Now, how can we still get those useful results?
I mean, I remember talking to someone in the civil service who said we have some really useful interesting data about school results broken down by ethnic background, but the problem is, of course, we can't make those public because outside of big cities in many places, there are really only a handful of pupils from par-ticular ethnic backgrounds, and once you've broken it down into a particular school and year group, you can literally tell who those people are.
Two of my secondary schools were like that. There were so few kids from ethnic minorities that if you said to me, "Oh, the two brothers from a Chinese family," I was like, "Oh yes, that's the guy in my math set or the guy the year below." So obviously you don't want to release a data set like that because then everybody in the world can see what results these two guys got because they're the only Chinese kids in the school. But you do want people to be able to look at the data and use it for all sorts of really important purposes to look overall. So, she was very excited actually in adorable way about what you could do with the data so that you could still use it without spoiling the overall results too much, but you could change it so that it didn't impact any individual's privacy.

Kate Bevan: A lot of people are very worried about data and AI and the harms of it. I'm going to sort of finish my last question, which kind of more focus your mind on it. Is this AI going to kill us all?

Timandra Harkness: They won't kill us all, might kill certain of us. I think we're still a very long way from any kind of artificial intelligence that can do anything like what humans can do. It's obviously better than us at certain things, mathematical processing, pattern finding, all that kind of thing much better, but it's not as sentient. It hasn't got any self-awareness. It hasn't got any purposes. It can't set its own purposes.
Also, human beings are making it, so if you're worried about what this machine that doesn't exist yet might do, you need to directly worry at the humans making it. Why would you make something that might be used for this purpose that would clearly be bad for at least me, if not the whole of humanity? I just think it's a distraction. I think things we should be worrying about are things like baking unfairness into the system by not being critical enough of what data we're using and deferring too many human decisions to artificial in-telligence. I think we're at a point in history where people are really reluctant to take responsibility for de-cisions and take the initiative and generally be in the driving seat of our lives, including people who are in authority. "I'm not responsible, then I'll just do what the computer tells me." It's really unsurprising, but deeply, deeply worrying.

Kate Bevan: I think that's a great place to leave it. Timandra Harkness, thank you very much for joining us.

Timandra Harkness: It's been an absolute pleasure. Thank you for having me.

Kate Bevan: The AI Interrogator is an Infosys Knowledge Institute production in collaboration with Infosys Topaz. Be sure to follow us wherever you get your podcasts and visit us on infosys.com/iki. The podcast was produced by Yulia De Bari, Catherine Burdette, and Christine Calhoun. Dode Bigley is our audio engineer. I'm Kate Bevan of the Infosys Knowledge Institute. Keep learning, keep sharing.
About Timandra Harkness
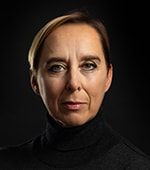
Timandra Harkness is a writer, broadcaster, and presenter.
Timandra is a regular on BBC Radio, writing and presenting BBC Radio 4’s FutureProofing and other series including How To Disagree, Steelmanning and Political School. BBC Radio documentaries include Data, Data Everywhere, Divided Nation, What Has Sat-Nav Done To Our Brains, and Five Knots. She was resident reporter on all 8 seasons of social psychology series The Human Zoo and is now the spare presenter for Radio 4’s Profile.
Since winning the Independent newspaper's column-writing competition, she has written for many publications including the Telegraph, Guardian, Sunday Times, Unherd, BBC Focus magazine, WIRED, Unherd, the New Statesman, Men's Health, and Significance (the journal of the Royal Statistical Society).
Timandra chairs and speaks at public events for clients including Cheltenham Science Festival, the Royal Society, the British Council, the Royal Geographical Society, the RSA, the Institute of Ideas, the British Library, Wellcome Collection, the Alan Turing Institute, the Royal Academy of Engineering, and many others.
Her book Big Data: Does Size Matter? published by Bloomsbury Sigma in 2016, came out in an updated paperback edition in June 2017. Her second non-fiction book will be published by Harper Collins in May 2024.
After performing improvised & standup comedy, & touring with a tented circus, she formed the first comedy science double-act in the UK with neuroscientist Dr. Helen Pilcher. Since then, she has written and performed scientific and mathematical comedy from Adelaide (Australia) to Pittsburgh PA.
In 2010 she co-wrote & performed Your Days Are Numbered: The Maths of Death, with stand-up mathematician Matt Parker. They performed the show to average audiences of 100.3 and 4 star reviews at the Edinburgh Fringe, then toured it in the UK and Australia. Timandra's science comedy since then includes cabaret, gameshows, and solo live show Brainsex (with Socrates the rat).
Timandra has a BA in Film and Drama with Art & Art History, a BSc in Mathematics & Statistics, and an MA in Philosophy from Birkbeck College. She is a Graduate Fellow of the Royal Statistical Society, and, from January 2024, an elected member of RSS Council.
Connect with Timandra Harkness
Mentioned in the podcast
- Infosys Knowledge Institute
- “Big Data: Does Size Matter” by Timandra Harkness
- Strava
- New York City Taxi Data Set
- “Generative AI Radar” Infosys Knowledge Institute