Knowledge Institute Podcasts
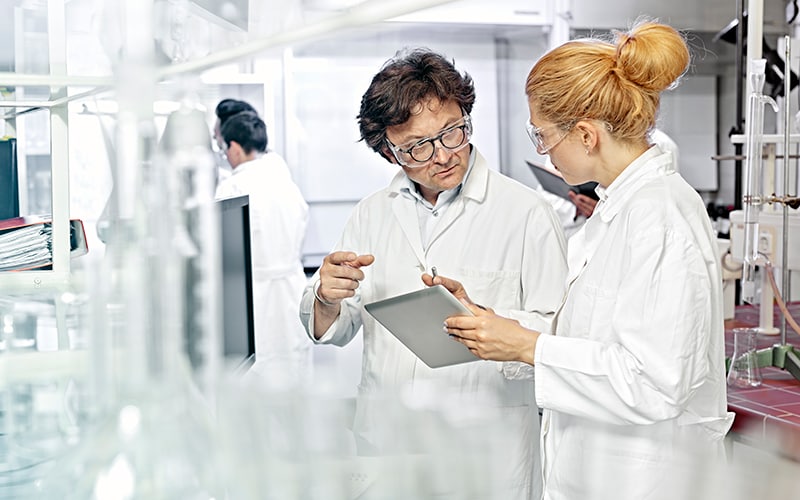
-
How Cloud-powered AI Revolutionizes Polyethylene Production with NOVA Chemicals' Kris Narayan
November 25, 2024
Insights
- Data normalization and contextualization are crucial for successfully applying generative AI in manufacturing operations.
- Automation and AI integration enable end-to-end business process optimization, improving efficiency and decision-making across the organization.
"By listening to this podcast, you hereby acknowledge and understand that your personal data may be processed in the manner described in our Privacy Statement"

Chad Watt: Welcome to Ahead in the Cloud, where business leaders share what they've learned on their cloud journey. I'm Chad Watt, Infosys Knowledge Institute researcher and writer. Today I'm speaking with Kris Narayan, leader of digital enablement and data platforms at NOVA Chemicals. NOVA makes polyethylene, a fundamental flexible form of plastic, and related products at facilities in Alberta, Ontario, Indiana, and Louisiana. NOVA makes plastics and makes decisions driven by data. We'll explore how an established chemical manufacturing business developed that habit and a cloud-based infrastructure to deliver it.

Chad Watt: Welcome, Kris.

Kris Narayan: Thank you for the invite, Chad. Happy to be here.

Chad Watt: Tell us about what led NOVA to develop a data-driven culture.

Kris Narayan: We've got an ambition of really focusing on being a sustainable polyethylene producer, and being one in the top Canadian value companies. A key enabler for us as a part of our strategy is to use digitalization as a foundational element to drive that particular outcome within our organization's strategy.

Chad Watt: Describe a little bit more about that data management approach, and how you get to a data-driven decision.

Kris Narayan: What we established is the key to creating those efficiencies is to really create a force multiplier using the vast amount of data that we have within our ecosystem. One of the elements over there was to take all of the data that we have got within the organization, and really make an enterprise data lake, or lake house as we call it, that can really foster the culture of operating our business through data and enabling decisions through the insights that we create using the data that we've got.

Chad Watt: From a cloud infrastructure perspective, what does it take to make use of that vast amount of data, and get it to the people who make the decisions?

Kris Narayan: We quickly realized that, with the scale of data that we've got, we need to have an underlying infrastructure which supports the growth, the existing volume, and simplifies the ability to interact with the data and do what the functional teams need to do with the data in a platform that can really drive that outcome for us. We were able to establish that doing that in a cloud-native way really helps us create that overarching technology platform that we need to be able to do our business analytics, our enterprise intelligence capabilities. And more importantly, get towards that advanced analytics, and apply the latest and the greatest gen AI capabilities against our data to derive the intended outcomes for our functional stakeholders that we have.

Kris Narayan: That's became the foundational base for us to get to that state. In that journey, when we were looking at the architecture of our data and the data platform, we actually doubled-down on going cloud-native. Creating that underlying foundational infrastructure that needed, which is scalable, which is resilient, and of course, which is secure as well.

Chad Watt: Let's go inside the plant, and talk about maintenance and turnaround. How is NOVA Chemicals using data and machine learning in that process?

Kris Narayan: What we did was we started with the predictive maintenance from a forecasting perspective. We had one use case where we wanted to look at optimization. That's where we thought, "Let's actually look at what does the overall value opportunity look like," and the possibility of really taking machine learning and advanced analytics to really improve the ability to look at forecasting the need for maintenance. At least, with the [inaudible 00:03:55] of 10 to 14 days.

Kris Narayan: What we realized quickly when we actually gathered the data from our manufacturing environment, and working with partners like Infosys, we quickly realized that there's a huge potential for us to quickly identify the need for maintenance and the window for maintenance as well. That was one of our first initial pilot use cases that we kicked off with. That's really shown a great outcome for us. What it has really enforced is applying a data-centric approach within manufacturing can result in really good yields for the organization. Value-wise, we've already realized the value that we had set out for with those initiatives. We are now expanding what we had built for a scoped set of sites to all of our other sites within the organization.

Chad Watt: What is your outlook on automation in the digital and data realm? You're dealing with vast quantities of data. How do you keep your lake and your lake house in order?

Kris Narayan: We almost look at it as a pyramid. Application really becomes the foundation of the pyramid which is generating the data, which really then gets utilized through automation to create the efficiency. Meaning what we established in our journey was as the applications create data, the need to connect to the data is very important, our integrate the applications is very important. That's where automation plays very critical role. We have seen that, in many of our use cases and pilots that we have done, in needing to actually have a robust strategy to automate our integration of platforms, as well as the data flow itself between the platforms and to the lake house stack we have built from an enterprise data platform perspective.

Kris Narayan: We looked at it and took an approach of saying, "Let's look at applications which provide us the data." Let's then overlay that with automation opportunities to connect the ecosystem, from a system perspective as well as data flow standpoint. Then make that available for analytics. And really apply AI, holistic sense, because we look at AI as something that can be applied at an analytics level. We can apply at an automation level. We can actually use applications that have AI capabilities built into it, which is where the industry today is really evolving. It's almost AI dots that analytics. More and more, as we see the evolution of the industry, more and more applications are natively applying AI, whether it's copilots or otherwise.

Kris Narayan: That was our strategy to get going with. That's the journey that we are in today. We see the counterpart of it, which is how the data really evolves. The data gets generated through applications, and there's a transformation and artifacts being built when we automate something and when we start applying analytics. That becomes the feedback loop within the overall ecosystem of generating data, using data, and regenerating new artifacts or new versions of the data. But bringing all of that together in an enterprise, centralized, normalized format is what is helping us to really make data a force multiplier for the organization.

Chad Watt: How do you decide what is worth automating, what you maybe should have a human touch on?

Kris Narayan: When we started looking at how do we really take automation and create value through automation, obviously the initial set of opportunities that we saw, that we heard, and that we received where frankly focused on task-level automation. It was a pain point that a user had, and they wanted to automate so that they could operate more efficiently within that particular business process.

Kris Narayan: But as we worked through some of these initial use cases, what we realized, what we came to realize was automation is about end-to-end business process efficiency creation. Now we're in a journey and in a state where we are working with our stakeholders to take the initial opportunity that we get, work with them to really help understand what does the end-to-end business process map look like, and what does the transformation landscape become by using automation, or intelligent automation as the industry calls it, to really drive a value proposition for the entire process map itself. That's where we are finding the core value proposition of automation is within the organization.

Kris Narayan: We are trying to do more. It's a journey that we are going through, because most of the times, we get requirements based on the pain point that the users have got. That's where we start, but we try to work with them to help them understand how we can really drive an overarching business process optimization by looking at it end-to-end.

Chad Watt: Manufacturing cloud. Tell us a little bit more about your digital operations. How much of it is in cloud, and how much of it is on-prem?

Kris Narayan: Today, our entire analytics work that we do, that's all cloud-driven. They are cloud-native. Obviously, we do have a footprint of applications within our own data centers. That's primarily because we have got plants, and the plants are in remote locations. So connectivity wise, as well as just the efficiency and latency, so we need to have some of these applications.
But core of our corporate assets that we have got, or services that we have got, has been migrated into cloud. That was something that we underwent a year or a year-and-a-half ago, where we actually made a judicious decision to look at our corporate footprint from a data center perspective, and do an analysis of what corporate or enterprise services we could migrate to cloud. Then, what are the key services that we actually need to have within our data centers which are near our physical assets? For obvious reasons.

Kris Narayan: That's where we are today. Everything that we've spoken so far, in terms of having our business intelligence capability, having advanced analytics, the AI opportunities that we are seeking, all of that is today executed using the cloud environment. Or using what we call our NOVA Cloud, effectively. That's where we are in our cloud journey.

Kris Narayan: You spoke about manufacturing cloud, and that's something that we are looking at what does that become? For us, manufacturing cloud is effectively a version of a cloud ecosystem which is closed to NOVA, secure and safe, and provides the flexibility for all our stakeholders to operate, to explore, to expand in a rapid manner without foregoing the aspect of security. As we all know today in the era of cloud, cybersecurity is a key issue. Within manufacturing, with the evolution of industry 4.0, with the connectedness, as well as really augmenting manufacturing processes with automation and AI, it's a key element.

Kris Narayan: In that journey, what we have realized is for us to really ensure that there is a trust in the cloud and the cloud services, you need to create a persona, in a sense. That's what we call manufacturing cloud, which is really an environment that is providing services in an absolute safe and secure manner to our organizational stakeholders. That's our evolution of what we call manufacturing cloud. It is how you build your cloud ecosystem relevant to the organization with the required controls and guardrails.

Chad Watt: Kris, at the risk of a chemical pun, what are the additional catalysts and inhibitors for manufacturing cloud?

Kris Narayan: Today the vendors themselves look at it as a key competitive advantage to be in cloud. From both scalability and efficiency, and ability to just innovate at a rapid pace. Because today, everything that we use has a sense of hybrid-ness. Meaning you could take an optimization problem, there's an approach through first principles, but there's also an approach through data-driven learning, which is applying ML. And inherently becomes a hybrid way of doing things. The only way you could do that effectively is if you have that particular cloud-native infrastructure set up to scale on demand, and to scale in an infinite manner, in a sense. That just becomes a natural catalyst for us in saying the destination is here, and we see that through the strategy of the key partners that we work with.

Kris Narayan: The inhibitors are quite common, and I think it's the same that we would find in any other industry. Security is the primary one, because they're all looking at cloud being an environment which is out there in the internet, accessible from anywhere. There is risks associated with that. Naturally, there are risks. But I think the aspect that one needs to put in front of the stakeholders is, "Yes, there are risks, but there are also controls that we can put in place to really manage those risks."

Kris Narayan: That's what we are trying to do work with our stakeholder groups, to help them understand and appreciate that we agree there are risks. But there are controls that we can place so that we are able to adjust those risks in hand, and actually manage them in a tolerant manner. That's the journey. Manufacturing cloud, one of the key aspect of developing something called manufacturing cloud is showcasing the cyber-proof readiness of that cloud environment.

Kris Narayan: That's the key for us, in ensuring that we are setting ourself up for success. Because as we evolve through the industrialization era, we are going to see more and more connected devices. When we see more and more connected devices within the plants and across the plants, the data has to flow in realtime, in an inventive manner. You got to process the data in realtime as well. How do you do that? The only way you can do that is actually have an infrastructure that can actually support that particular ability to consume, transform, and then compute on that particular data.

Kris Narayan: For us, that's where cloud becomes a key trend for us to do. But then, obviously, we can't do it if we don't have the necessary guardrails to secure that particular environment and secure our data. Because in manufacturing, security from a data perspective, as much as there's the confidentiality aspect of it, the integrity is also very critical because everything that we do on the data is on the foundational principle of the data that we have used has the required data integrity. Because if the integrity of the data changes, then the outcomes are changing as well. That will impact safe and reliable way of operating our plants.

Chad Watt: Now we've talked a little bit about machine learning and your use of that with the predictive maintenance use case. How else is NOVA Chemicals using artificial intelligence?

Kris Narayan: We are looking at how we can use gen AI capabilities for driving knowledge management. We are also investigating and looking at how do we use gen AI for our productivity day-to-day. Something that used to take, say 10 minutes, how can we do it in five minutes? How do we really augment our people with these AI capabilities that makes them do their job more effectively and with easy? Both aspects.

Kris Narayan: I always think that experience is always at the forefront. Any technology that you introduce has to create a better experience. With better experiences, when you get adoption. This axis of digitalization is adoption. If you can't get adoption, then you may have the technology, but it never gets utilized. That means you're not able to really realize the value of it. For us, that's how we look at it. Any opportunity that we look at with AI, with gen AI, really the first thing is what is the experience that it is creating? And how it is going to drive the adoption in an organic way possible. That's where we are.

Kris Narayan: Now we are really looking at exploring the gen AI aspect of AI, and what that could mean for us in terms of the copilots, or other applications of large language models. And non-language models. Today, LLM, where it started with respect to gen from an industry perspective, today LLMs have grown. It's not just about language, it's about image, it's about voice. We are looking at all of that to see what does that become. What does that AI strategy, from a longterm perspective, become? Including the likes of AI agents. If you go back 10 to 12 months ago, chatbots were a thing. Then we had virtual agents. Today the natural progression of that is AI agents, which means they are more smarter, so cognitive by default. How we are able to leverage that. Use AI for process mining. How we can actually mine our existing processes and apply AI on it? What does that culminate into?

Kris Narayan: Those are some of the exploratory areas for us to work through. But that's where we are in the journey.

Chad Watt: Are there some things that that data-driven culture is pushing you guys to pursue with generative AI that, perhaps if you weren't so data-driven, would not be on the table?

Kris Narayan: What we focused on was not on the data science or advanced analytics to begin with. We actually focused on really looking at engineering the data well. So ensure that the data ingested, ensure the data is transformed, and create first level of normalization of the data. Creating a normalized data is really critical. Then we focused on how do we actually embrace data science. How do we embrace advanced analytics? How do we apply generative AI? That's what our take of it has been.

Kris Narayan: The thing that has helped us to connect the dots specifically has been when you get to a phase of applying generative AI with the data that we have, and the models that we have created, the normalization of the data itself, really simplifies the ability to use generative AI technologies on the data. The total runtime to take a particular use case and apply generative AI concepts becomes much faster. That's what we have realized. I'll maybe go into details for that.

Kris Narayan: One of the things that we have realized is contextualization of data and the importance of contextualizing data to be able to apply generative AI. Whether it's generative AI from Copilot, in being able to go in and query about work orders, query about past maintenance activities, or as such. What is needed is you need to contextualize the organizational data. Which is effectively, in industry terms, creating a bit of a knowledge draft of your organizational data, which results in building a data twin. You've got a data twin that is reflecting your business context, and has relationship within the data itself that you've ingested. That really amplifies our ability to go and overlay a generative AI capability, to go and ask questions about your data. Because naturally now, you've got the dataset that has inherently strong relations created between individual data domains that is able to answer queries more effectively, as well as accurately.

Chad Watt: Can you put your finger on a particular capability that this enables for you?

Kris Narayan: We've just started our journey, Chad, so we have not gotten to a state of creating an enterprise knowledge draft, but that's our end state objective of creating an enterprise knowledge draft.

Kris Narayan: With our initial proof of concepts and proof of value that we have looked at, what that's helped us do is two things. One, I alluded to, was creating copilots for our manufacturing stakeholders, which really simplifies their ability ... I'll take an example. If you think of it from a maintenance perspective, and you've got planner personas in your maintenance business processes. It helps a planner to actually go and be able to query about a work order history. To query about a certain maintenance activity that might have been performed on an asset within the plant. The overall time to now go and get a summary of what was done is going to go from, I don't know, maybe 10 or 15 minutes if they are in a previously going into an application, extracting that, and then analyzing it. Versus now, prompting it to your copilot and asking for it. That's one thing that we have seen can really drive efficiency.

Kris Narayan: The other thing that we noted was creating, think of it as an enterprise search platform for manufacturing operations data. If you did not have a contextualized data, what you would otherwise do is you would take your data from your historians, you would take your data from your PNIDs, and smart sheets, and so on and so forth, and then you would try and put them together, and manually create a bit of a join of that dataset, for a lack of better term. But having contextualized layer, what it helps is it helps us create an enterprise search capability. Where you could go in and click on an asset, and then with that asset, you can now look at all the techs. You can look at even some alarms. Everything is now in a single page, contextualized in and around the asset that you searched for, and gives you an entire history of it. What might have taken an hour, two hours, or a day, depending on the organization, now you can do it in minutes.

Chad Watt: Thanks, Kris, for your time today.

Kris Narayan: Thank you very much, Chad. Again, it was a pleasure being part of the podcast here. Appreciate the invite. Have a great day.

Chad Watt: You're very welcome.

Chad Watt: This podcast is part of our collaboration with MIT Tech Review, in partnership with Infosys Cobalt. Visit our content hub at technologyreview.com to learn more about how businesses across the globe are moving from cloud chaos to cloud clarity. Be sure to follow us and follow Ahead in the Cloud wherever you get your podcasts. You can find more details, and our show notes and transcripts at infosys.com/iki in our podcast section.

Chad Watt: Thanks to our producers, Christine Calhoun and Yulia De Barre. Dode Bigley is our audio technician. And I'm Chad Watt, with the Infosys Knowledge Institute. Until next time, keep learning and keep sharing.
About Kris Narayan
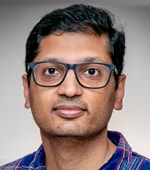
Kris Narayan is an avid technologist with over 15 years of experience in IT having led and managed various functions/areas such as information security, technology operations, enterprise integrations, architecture, secdevops, and digital enablement. Kris has a background in computer science and software engineering having completed BE and PhD in the area, he has worked in various industry verticals such as Fintech, SaaS, Energy, Transportation and Manufacturing, and Academia.
Kris currently works at NOVA Chemicals where he leads their digital technology practice that involves data management, analytics, automation, and cloud enablement.
On LinkedIn
About Chad Watt
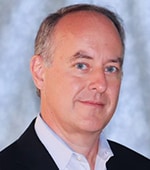
Chad Watt is a researcher and writer for Infosys Limited and its thought leadership unit, the Infosys Knowledge Institute. His work covers topics ranging from cloud computing and artificial intelligence to healthcare, life sciences, insurance, financial services, and oil &gas. He joined Infosys in 2019 after a 20-plus years as a journalist, mostly covering business and finance. He most recently served as Southwest Editor for a global mergers and acquisitions newswire. He has reported from Dallas for the past 18 years, covering big mergers, scooping bank failures and profiling business tycoons. Chad previously reported in Florida (ask him about “hanging chads”) North Carolina and Texas. He earned a bachelor’s degree at Southern Methodist University and a master’s degree from Columbia University.
On LinkedIn
- “About the Infosys Knowledge Institute”
- MIT Technology Review
- NOVA Chemicals
Mentioned in the podcast