Insights
- Investment in artificial intelligence continues to experience significant growth worldwide.
- Organizations are determined to accomplish AI-first business transformation, not wanting to be left behind.
- Certain practices can help them succeed.
- Companies need to think about what problem they are trying to solve with AI, pick a use case with quantifiable benefits, invest time and effort to create a well-constructed roadmap for execution, and make investments that help them drive business impact.
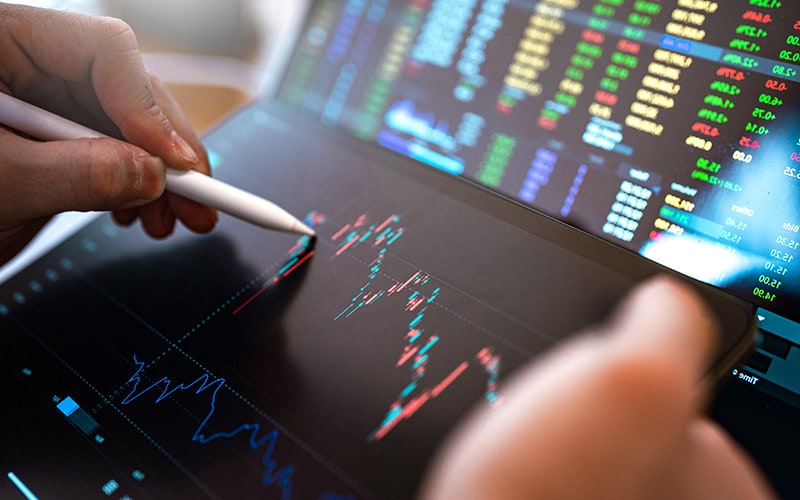
Global investment in artificial intelligence (AI) centric systems is expected to exceed $300 billion by 2026. Successfully adopting AI is predicted to increase the profitability of organizations by 38% on an average by 2035. Unsurprisingly, there is a race among organizations to achieve AI-first business transformation to develop the ability to adapt quickly to economic challenges, cut costs, maximize revenue, improve operational efficiency and productivity, and meet client needs optimally. But what businesses need to do for it?
As part of the Being AI-first Conversations: Richardson Chapter event organized by Infosys Topaz, a panel discussion titled AI-first Business Transformation was moderated by Sunil Senan, senior vice-president, and head of data Analytics and AI at Infosys. The panelists were Maurice Tayeh, global CIO, Hatch; John George, group vice-president, digital & technology, global workplace solutions, CBRE; and Gopal Alagiamanavalan, SVF, apps development group manager, Citi. The experts discussed their experiences in leading business transformation initiatives for their organizations, and the best practices for other companies aiming for similar outcomes.
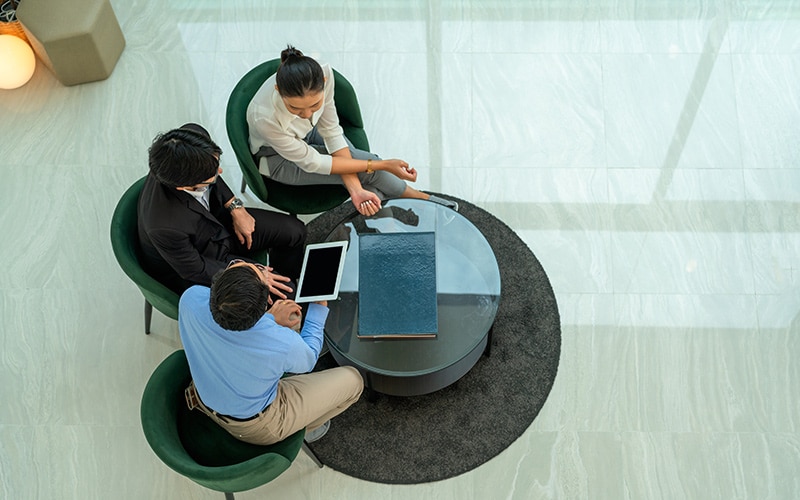
Building a case
Infosys’s Data+AI Radar 2022 emphasizes the need for companies to think about what problem they are trying to solve with AI – in other words, the use case – before they deploy it, to get satisfaction out of their AI deployments. An effective use case is one which is has quantifiable benefits, is highly repetitive, volumetric-centric, consistent, relevant, and contextual in terms of customer service. “In my experience, the product team comes with a requirement, and it is delivered using either AI or generative AI. But when the business team is asked what the quantifiable benefits or return on investment are, they usually say it is too early to tell. Achieving an AI-first business transformation requires companies to be proactive about finding out what pain points they need to resolve, and what results they want to achieve – with the AI technology – be it reduction in manual effort or cost savings,” says Gopal Alagiamanavalan.
An effective use case is one which has quantifiable benefits, is highly repetitive, volumetric-centric, consistent, relevant, and contextual in terms of customer service.
Creating a roadmap
Business transformation can mean different things to different organizations. When it comes to using advanced AI for business transformation, most companies are in the experimentation phase. While that is a reasonable starting point, it is crucial for them to find ways to harness their experimentation and innovate to arrive at concrete business capabilities which can help them achieve measurable business outcomes.
Maurice Tayeh explains the importance of having a well-constructed framework in place. “Hatch is an engineering company. So when you talk about innovation and give 10 engineers the same problem to solve, you get 10 solutions. We had no dearth of solutions and we examined all of them to help us create a project execution framework with which the business transformation journey can begin. The framework covers the game plan from the time you get a proposal to the time you execute the project and hand it over to your client. It should also guide you on how to adopt some of the strategies in your organization’s practices. We started a major digital transformation initiative a few years ago, and we spent a lot of effort and money building the framework. But that is what has been the bedrock of where we are today and what will propel us into the next phase of innovation.”
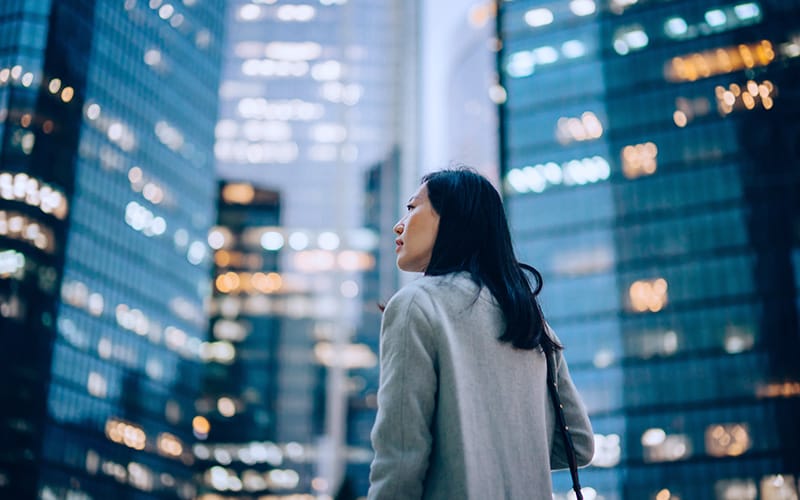
A well-constructed framework lays the foundation for success in AI-first business transformation and helps organizations leap into the innovation phase when it’s time.
After the systems have been put in place, good data is indispensable to continue the momentum of the transformation. A comprehensive analysis of the data helps determine the actions ahead and the right decisions required for it, backed by AI and generative AI in combination with advanced technologies. “While focusing on a new technology, it is essential to also prioritize the people who will use the technology. Companies should consider what impact the technology will have on them, how their work processes will change, and how to help them adopt the technology effortlessly,” Tayeh points out.
Delivering business value
Businesses need to carefully plan their AI-first business transformation. Having a clear vision of the outcome, ensuring it is aligned to business goals, and has stakeholder buy-in, is key to project success and delivering projects that have business value. Organizations need to work out the business value, whether it is through metrics such as revenue increase, profitability growth, brand perception, or market share. After delivering the results, communicating their business value to the client effectively makes all the difference in creating customer satisfaction.
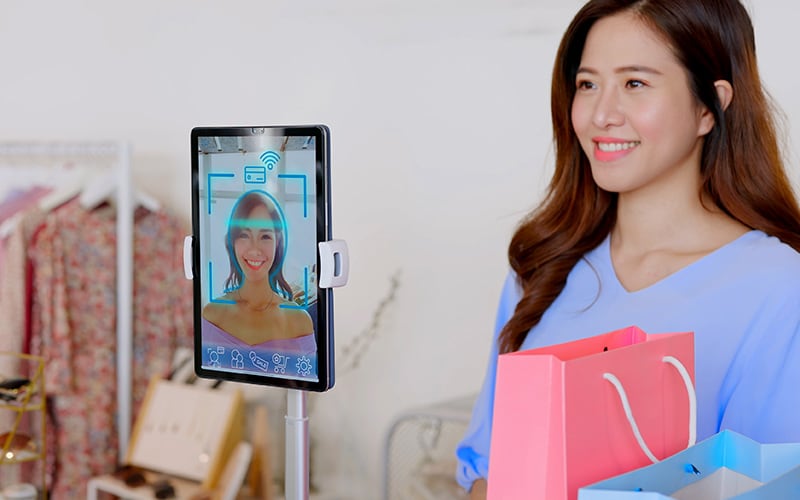
Having a clear vision of the outcome, ensuring it is aligned to business goals, and has stakeholder buy-in, is key to project success and delivering projects that have business value.
It also helps organizations make decisions regarding their future investments. To make the right investments, businesses should evaluate them through the lens of how it will help them drive business impact, if what they are setting out to achieve will be a market differentiator, how will it drive value for their clients, or how it will drive internal efficiency. “An example of where CBRE believes we have used AI to create market differentiation is in the case of smart buildings, where typically around 70,000 alarms are generated in a month. We have been able to use AI to bring down the number from 70,000 to about 1,500, and determine which alarms to act on and which ones to ignore. This has helped save technicians effort, and reduce carbon emissions, not to mention noise levels,” says John George. He adds: “What I have noticed with implementation is, we will be able to get to 80% of the answer very quickly. And the remaining 20% will probably take millions of dollars in terms of investments. The key lies in being able to recognize when it is worth going ahead and when to stop. There’s tremendous value in being transparent about it with your stakeholders, to build better customer relationships.”