Insights
- Generative AI is evolving at a rapid pace.
- Companies are using it to improve their businesses and boost employee productivity.
- However, scaling generative AI will require them to build well-defined strategies.
- That includes planning a robust infrastructure, identifying the right business use case, managing risks, and training employees in new skills.
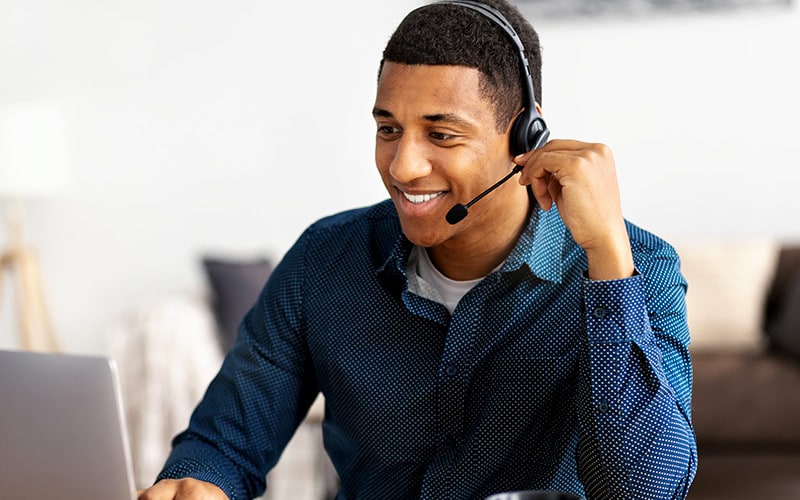
Generative AI has created a stir across industries with its ability to create different forms of content. The technology is advancing rapidly, and organizations have been quick to use it to save time and effort for their workforce. But scaling generative AI and using it successfully to meet the demands of different teams across an organization as well as clients, demands an intensive strategy and effort.
As part of the Being AI-first Conversations: Richardson Chapter event organized by Infosys Topaz, a panel discussion titled Scaling Generative AI was held. Moderated by Prasad Joshi, SVP and head of Infosys Center for Emerging Technology Solutions, it included panelists such as Cameron Gwinn, senior director, KPMG; Hitendra Kashyap, senior vice-president, Bank of America; David Williams, assistant vice-president, automation, AT&T, and Diganta Nayak, executive director, Verizon. The experts discussed the various aspects that companies need to consider when scaling generative AI, and how they execute related strategies.
Scaling generative AI and using it successfully to meet the demands of different teams across an organization as well as clients, demands an intensive strategy.
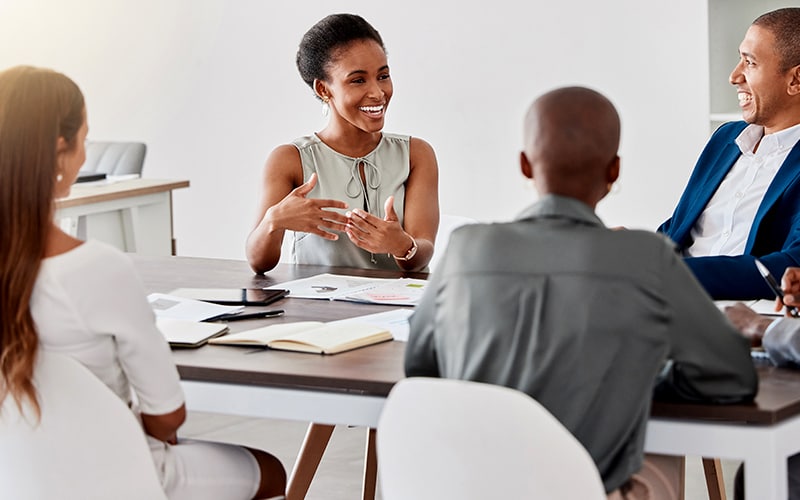
Ingredients for scaling
Before businesses can plan the adoption of generative AI, it is imperative for them to identify the right business use case for it and develop a strategic vision for its execution. An often ignored but critical next step is that of getting people practices right. “Among key strategies required to prepare for scaling generative AI, training your workforce in the new skills that the deployment demands – such as prompt engineering and fine-tuning – is foremost. Then comes the hiring of key resources from the industry who can help your organization navigate its journey in harnessing the power of generative AI,” says Diganta Nayak.
Planning the infrastructure required to deploy AI, particularly in terms of managing data quality, storage, and computation, is of utmost importance. Culture is also paramount. Developing a culture of innovation prepares teams for experimenting actively – with a product-centric value delivery mindset – where potential failures are viewed as opportunities for learning and growth. That said, organizations should be mindful of costs and carefully assess the return in investment before scaling generative AI. “Scaling is a two-pronged approach. The first one is to be able to scale to the demands of the business. You have created a generative AI powered application. But can it sustain the load of 10,000 requests hitting it concurrently? Then you should consider the scaling of the framework. If there is an infobot you have developed, can it scale to 10 other infobot use cases your organization may be needing? These are some aspects to consider,” notes Nayak, emphasizing that Verizon makes sure to keep the customer and employee experience at the core of its generative AI use cases. Collaboration with industry experts, peers, and research institutions is another practice that the organization follows to share knowledge related to this technology and advance collectively.
Before businesses can plan the adoption of generative AI, it is imperative for them to identify the right business use case for it and develop a strategic vision for its execution.
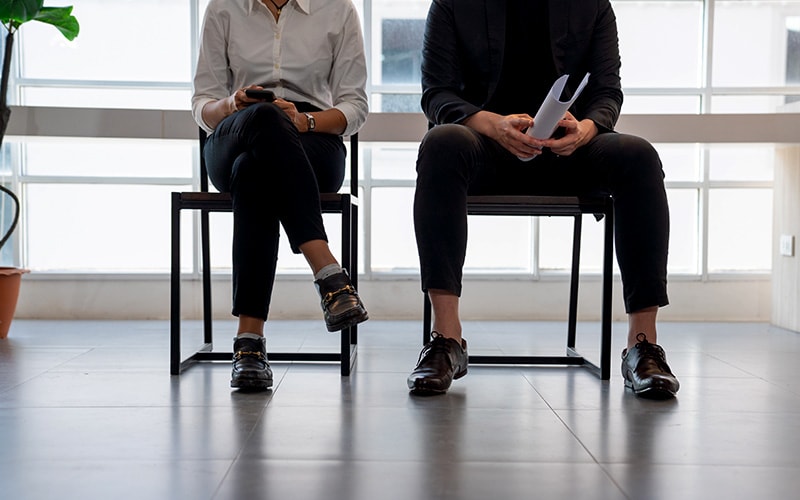
Instances across industries
The use cases for generative AI are many, be it creation of marketing content, design elements, code, or even new food flavours. “Generative AI works wonderfully with other technologies that we already have. Things we have been able to do include customer service, where every interaction between the agent and the customer is being captured. We have been able to use generative AI to produce a call summarization of the two talking, so that the agent does not have to manually write it out. It is comprehensive, saves time, and is a good capture of what happened on that call so that the agent does not forget anything, and the next agent can see where they need to pick up from,” says David Williams.
In the financial services industry, most companies are creating proofs-of-concept and pilots to experiment with generative AI. But the example of customer service is still a major one in terms of how the industry is scaling generative AI. The technology is making a deep impact in driving customer centricity, by means of chatbots that customers can use for self-service. While at present such chatbots provide basic account-related information or FAQs that simplify complex concepts for the customer, the scope for personalization is a game-changer. Hitendra Kashyap points out that generative AI has the potential to provide customers with personalized service on different channels: advise related to wealth management or investments. It could also be useful in generating reports related to organizational audits to be submitted to a regulatory authority, saving employees hours of time and manual effort.
Key considerations
While companies are eager to adopt generative AI, they should be mindful of the risks involved, be it ethics or security-related. They should have a robust framework that equips them to manage aspects such as governance, and responsible and ethical AI. When it comes to approaching generative AI, the first thing to consider is security. “Organizations have to decide if they want to opt for open source or closed source. AT&T decided to start with closed sources. Providing a safe sandbox in which your technical practitioners can start to have some generative AI discussions is key,” Williams emphasizes.
While companies are eager to adopt generative AI, they should be mindful of the risks involved and have a robust framework to manage them.
Having a strong data strategy is necessary, especially for businesses that deal with sensitive data and should pay attention to the permissible use of data. They should also use their discretion before passing on the guidance generated by large language models (LLMs) to clients. It is critical to examine the sources and quality of data that the LLM has tapped into, and ensure that the model is functioning optimally before it is deployed.
Generative AI is a continually evolving technology, which makes it difficult for leaders to take decisions related to it, knowing that new and improved developments could be just weeks or a month away. “It is about getting the technology into people’s hands so that they understand what it is and how to work with it. This way the technology teams can start working on bigger applications that can be much more impactful down the road. In this business, expert-level knowledge is extremely important, and so the ability to build some of the retrieval augmented generation (RAG) based applications becomes significant,” says Cameron Gwinn.
With generative AI being an emerging area, companies trying innovative approaches, and new research continuing to unfold, the future looks exciting for this technology.