Insights
- Generative AI drives business value, but compromised data can hamper progress.
- Artists, programmers, and writers can deliberately spike data, rendering foundation models useless.
- This can happen on a large scale through image poisoning, nonfunctional scripting, and, in the case of writers, generative AI training on nonsensical articles littered across the web.
- Businesses can roll back to datasets free of poisoning; train on smaller but higher quality datasets; use rigorous adversarial testing techniques; and most importantly, curate and verify data before adding to the training dataset.
- Firms can take definitive actions such as putting money behind the creator industry, buoy up the data science profession, and promote the need for human-centric copy creatives.
With thousands of foundation models and big money flowing in generative AI, the top seven tech giants are riding high. Apple, Microsoft, NVIDIA, Alphabet, Meta, Tesla, and Amazon collectively fueled 50% of the S&P gains in 2023.
How long will this heyday last?
Well, opinions vary.
Some say it will end this year itself, while others think it will continue for many years to come. Infosys Generative AI Radar reports found that many firms have already realized significant value capture from this technology and expect more with advancements ahead.
Yet, a case of compromised data can hamper progress. Content creators have already objected to their work being scraped to train underlying foundation models and may retaliate by deliberately destroying datasets that feed those models. This can happen even if consumers know the provenance of data used to create new works through an AI watermarking feature that could soon become mandatory after President Biden’s executive order on artificial intelligence late last year.
Generative AIs – from ChatGPT to Bard to Stable Diffusion’s latest models – require vast quantities of data to determine input weights effectively.
If this data is poisoned, or tricked into seeing things in the data that isn’t there, then the artwork, or code, or business editorial that foundation models produce will be full of hallucinations, rendering them essentially useless.
Artists, programmers, and business writers, among others, whose roles may be affected by generative AI, have strong incentives to see this occur. Such a scenario can produce an arms race between big enterprises using generative AI and creators who might spike the data.
Firms will then need robust responses to counteract this trend, including buying and curating pure datasets, along with better model security measures.
A wave of AI lawsuits
Programmers, writers, and artists lay the groundwork for training generative AI. Creators are already turning to boycotts and court appeals to protect their work from unauthorized commercial use and improper licensing. Novelist John Grisham and other prominent authors have taken OpenAI to court, and programmers allege Codex crawls their work but don’t “treat attribution, copyright notices, and license terms as legally essential.” Visual artists are taking legal actions against Stability AI, Midjourney, and DeviantArt for copyright infringement, alleging unauthorized use of their work. In response, some big firms ensure commercial customers that they will cover legal costs if sued over generative AI output. Microsoft is a case in point. Discussions revolve around paying publishers so chatbots can surface links to individual news stories in their responses.
Following the US executive order, companies like Adobe have floated the idea of marking data as ‘not for training’ through an anti-impersonation law. And to get ahead of what’s coming, AI companies, including OpenAI, signed an agreement with the White House to develop a watermarking system to let people know if something was generated by AI but made no promises to stop using internet data for training.
Some new tools block web crawlers from OpenAI and other foundation models. OpenAI said website operators can disallow its GPTBot crawler on their site’s Robots.txt file or block its IP address. However, they cannot retroactively remove scraped content from training data used by models like ChatGPT.
Attack of the bereaved
Regardless, content creators will experience some income loss, potentially leading to further action from them. Game theory shows how this might play out: these same groups that are under threat are likely to look for ways to stop generative AIs scraping their work and being used to replace them. One way to do this is through data poisoning. Researchers at the University of Chicago, led by Professor Ben Zhao, have already developed two tools, Nightshade, and Glaze, to help artists poison their artwork by subtly changing pixels to trick generative AIs into misinterpreting images.
On a small scale, this protects an individual artists’ work. But on a large scale, this may render generative AI useless. Producing this effect on a large scale is possible using generative AI. Tools like Glaze and Nightshade process the images, infecting the data, and once uploaded to image sites across the internet, they will be scraped and collected for ingestion by other generative AI technologies. In the quest for data to finetune models such as Bard and ChatGPT, these legitimate technologies will ingest poisoned data that will make them worse, and quickly.
Business writers have a similar path to self-destroying generative AIs, requiring no special tools. They could use generative AI to write articles with nonsensical jargons or an argument converse to true business writing (cloud computing is ineffective and problematic, for example), and hallucinations repeating the nonsense will increasingly appear in the foundation model’s output. They will then create thousands of new business news websites and seed these articles widely. They will also ensure that these sites are easy to scrape and ingest, and are search engine-optimized to appear more useful. As an added layer, they can use an outside tool to constantly search for terms on these fake news pages and click the links, increasing their importance to the search engine. The result will be the same as it will be for artists, with generative AIs being far less effective than they are currently.
The third group, the programmers, won’t even need generative AI: scripting will suffice. The point for them will be to fill the internet with nonfunctional code. In the same vein as the business writers, their goal will be to create thousands of websites that appear to be focused on helping programmers code but with actual code snippets remaining nonfunctional. Because generative AI is looking to predict the next sequence of characters in a string of code, something simple like placing a semicolon at the end of every line in Python, programmers can befuddle generative AI.
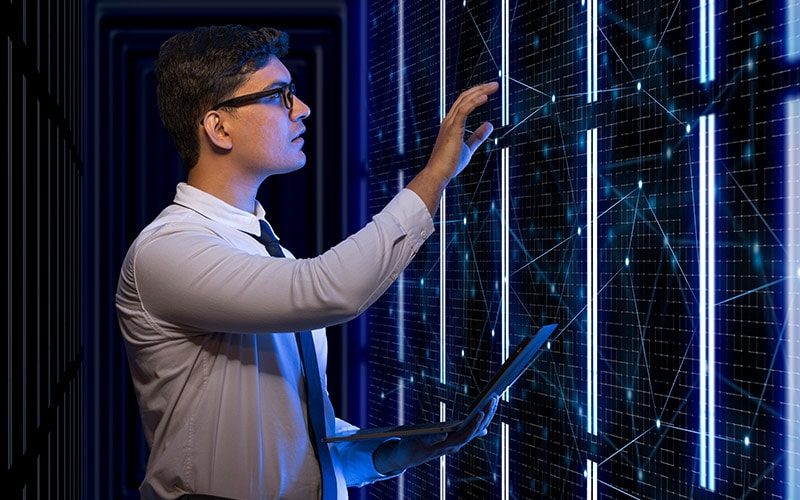
The arms race: What this means for large corporations
If content creators do seek to poison the AI well, an arms race could start, with businesses fighting back to stop this. However, before they find a way to ensure the data on which they train their generative AI models is pure, much damage will have been done, with poisoning making newer versions of ChatGPT, worse than older versions.
Creators of foundation models have a few tools in their arsenal, though. Here are a few:
- First, firms such as Anthropic and Google can roll back to training on datasets free of poisoning.
- Second, new research can uncover novel solutions, including training on smaller but higher-quality datasets.
- Firms will want to build robust defenses against these attacks, beyond data curation. “We don’t yet know of robust defenses against these attacks. We haven’t yet seen poisoning attacks on modern [machine learning] models in the wild, but it could be just a matter of time,” said Vitaly Shmatikov, a professor at Cornell University who studies AI model security. “The time to work on defenses is now.” Some examples of stopping poisoning in its tracks include rigorous adversarial testing and homomorphic encryption, which we detail in this Infosys Knowledge Institute paper.
- Finally, data science teams can curate and verify all data before adding them to the training dataset. For example, in healthcare, third parties such as Defined.AI can collect prompt and response data. These datasets comprise numerous real-world physician prompts and their corresponding machine-generated responses. They include both clinical and nonclinical conversations. The firm also provides pure datasets across life sciences and engineering. However, this will increase costs of adding new data to models, potentially impacting profits of firms selling generative AI solutions.
Let’s not overreact
Artists and creators have protested, are protesting, and will continue to protest unless large organizations show that generative AI not only benefits business but also empowers artists, programmers, and writers.
Important here is to not overreact on regulation.
Omar Al Aloma, the United Arab Emirates AI minister, says that premature technology regulation, motivated by fear, led to the decline of the Ottoman Empire. The printing press had just been invented, and in 1515, the calligraphers came to Sultan Selim and said: “We’re going to lose our jobs, do something to protect us.” He overreacted, banning one of the most important technologies the world has ever seen.
The same thing can happen with AI. Instead, upskilling and reskilling ventures must be top of the enterprise to-do list. We found that programmers are much more productive when using AI, especially junior programmers.
Reduce fear of this new technology and show that firms care about people who can potentially be displaced.
Instead of marketing initiatives that act as window dressing and hand waving, firms should put money behind the creator industry, buoy up the data science profession, and promote the need for human-centric copy creatives, including the sort of thought leadership we write here at the Infosys Knowledge Institute. This will do a lot to ensure the scenarios suggested here don’t become a thorn in the flesh to innovation, just when we need it the most.