“Financial stability cannot depend on omniscient supervisors identifying and preemptively defusing any potential source of crisis; it requires safeguards that can help the system withstand the force of a severe storm, and tools the government can use to limit the damage.”
The runup to CECL
In early 2000s regulators were lenient toward banks and regulations allowed banks to lend more. Unchecked lending and willful disregard for a borrower’s ability to pay led to the 2009 financial crisis. It also revealed that the growth of credit-loss reserves did not keep pace with the rise in loan growth1 (Figure 1). The five-year loan growth CAGR (2002-2007) was 9%, against 4% for loss reserves.
The crisis turned the tables on lending, and regulators placed strict checks. Interbank lending froze with minimal or almost no consumer lending activity. At the same time, the growth in loss reserves surged immediately post the crisis.
Figure 1. Loan growth outpaced loss reserve growth prior to the crisis (U.S., 1993-2006)
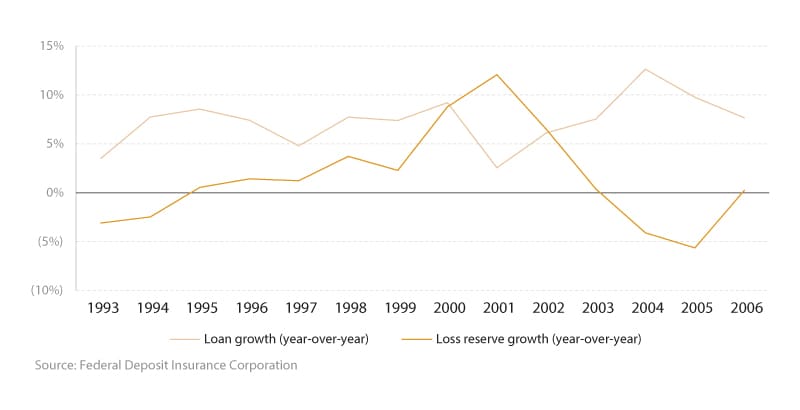
Investors increasingly became aware of the inherent risks of the incurred loss method to delay the recognition of credit losses until they meet the probable threshold at which a loss is incurred. Investors criticized it for being backward looking and restricting an institution’s ability to record expected credit losses.2 In fact, the loss allowance coverage was the lowest in 20 years at 1.15% (Figure 2). This prompted a need for a better, more accurate accounting standard to clearly reflect the state of loans.
Figure 2. Loss allowance coverage was the lowest in 20 years prior to the 2008 financial crisis (U.S.,1986-2018)
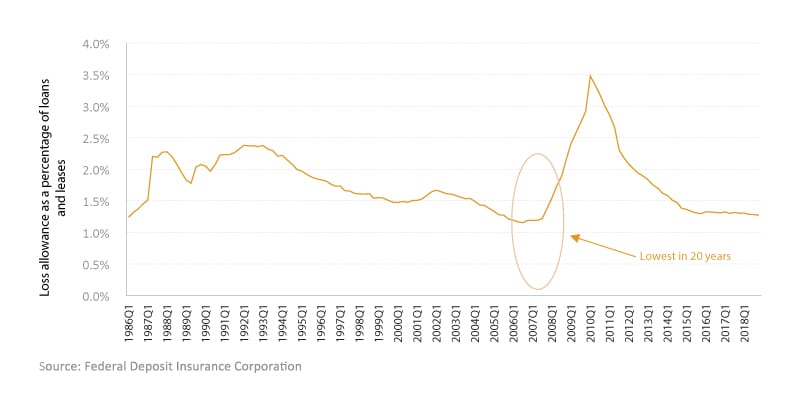
Financial institutions are unique, with varied portfolios and different risk appetites. To overcome these dissimilarities, and to strengthen the financial system, the regulator has to ensure all institutions recognize and report an accurate projection of credit losses.
The Financial Accounting Standards Board (FASB) proposed the Accounting Standards Update Financial Instruments — Credit Losses, and its impact on allowance for loan and lease losses, that introduced the CECL methodology for estimating allowances for credit losses in order to address the weakness of the current incurred-loss approach. CECL expects banks to use historically data-driven credit-loss computation methodologies and to apply statistical modeling techniques where appropriate. The model looks to establish a clear, causal relationship between accounting and economics, and further strengthened with macroeconomic variables, risk factors, and credit losses.
Closing loopholes in the old standard
CECL will replace the present incurred loss standards — FAS-5 and FAS-114 — and will enable institutions to calculate losses using an expected loss method. The standard provides guidance on how entities should measure credit losses on financial assets held at amortized cost. The CECL measurement of expected credit losses encompasses relevant information on past events and experiences, current conditions, and reasonable and supportable forecasts that affect the collectability of the reported amount.
The credit-loss estimation method is a major change from the current impairment model. CECL is proactive compared to the current reactive, incurred-loss computation.
The FASB states the new standard will improve financial reporting as it requires a timelier recording of credit losses.
Credit loss estimation under CECL
The standard expects banks to account for financial instrument losses from inception until the estimated life of the instrument or “life of loan” — the difference between the loan at amortized cost and the expected recoverable amount. However, the FASB only provides guidelines and gives institutions the flexibility to use any of the below methods to estimate credit losses, based on the size and complexity of their portfolio. The methods include:
- Roll rate: Credit losses are computed based on historic roll rates (migration from one delinquency bucket to another) against a portfolio.
- Vintage analysis: The portfolio is grouped based on the age of origination. Future losses are estimated on the back of average historical losses, with the macroeconomic outlook adjusted based on qualitative (Q) factors.
- Loss rate: Different pools are created within the portfolio segment. Future losses are estimated based on average historical losses against the pool.
- Probability of default: The default rate is computed using the lifetime probability of default (PD) and loss given default (LGD), for expected credit loss (ECL), against a static pool or for each account.
- Discounted cash flow: The present value of expected future net cash flows is used to compute losses. This method is applied at an account level.
Figure 3. Evaluation of CECL methodologies, in order of increasing complexity
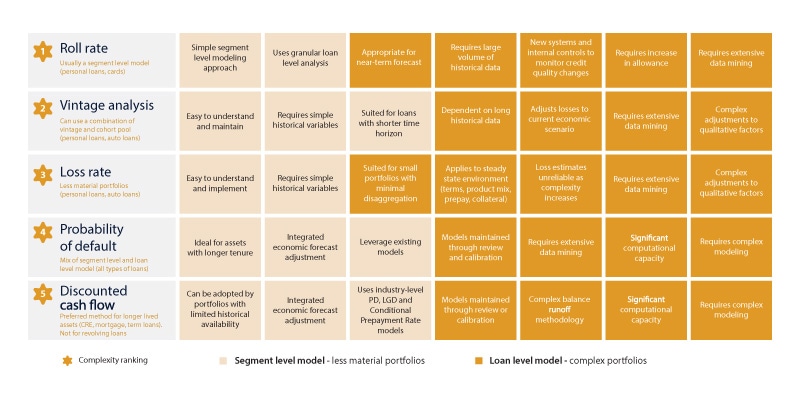
Steps to implement CECL
- CECL Standard Interpretation
Forecast losses at the primitive level — broken down into subsections of business activities. Drill down to the product level if necessary or due to methodology requirements. - Data Management
The most difficult part is to identify or source historical data on a portfolio or product level — the raw material needed at the starting point. Data analysts spend hours seeking to understand the historical behavior of products and performing comparative analysis, to arrive at a suitable method for each portfolio. - Segmentation and Methodology Selection
Build the model based on prescribed methodologies in conjunction with macroeconomic data. Challenges include setting up the macroeconomic data repository and usability as per the guidance of each institution’s economic department. - Model Build
Historical data, in the horizon of three to 10 years, plays a pivotal role in setting the tone of validation for the model. Tracing the validity of the sourced data is vital as it decides the relevance of loss-forecasted data and partitioning, depending upon the policy or trend change. - Data, Methodology, and Model Validation
Checks include data, assumptions, methodology, and performance testing to ensure models are fit. - Disclosures and Reporting
Test the model for at least a quarter for computations, application integrations and reporting templates. This will help provide a clear view to management.Improvements in validation can be found using artificial intelligence (AI) and machine learning (ML) as an ongoing process using automated model recalibration techniques. - Integration Governance and Monitoring
After going live, banks can look at strategically integrating CECL processes with similar regulatory assessments including comprehensive capital analysis and review (CCAR), Dodd-Frank annual stress testing (DFAST) and Basel risk-weighted assets (RWA). This will create synergies in data procurement, model review, and reconciliation.
Figure 4. Seven step CECL execution plan
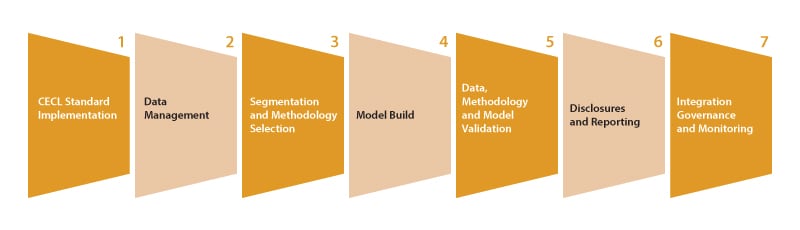
Upcoming CECL focus areas
With less than a year to go (public business entities that are SEC filers are required to adopt CECL effective Dec. 15, 2019), banks are well into their CECL implementation journey.3 Most surveys indicate that a majority are in the final stages of standard interpretation and data provisioning. Over the next few quarters, banks will focus on the following areas:
Figure 5. CECL implementation focus areas
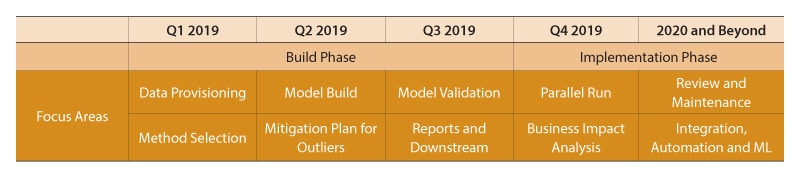
CECL implementation challenges
Large banks can mitigate challenges in implementing CECL, which forecasts loan losses similar to IFRS 9, but they still face the following concerns:
Data limitations
Concerns related to historical data still exist, as banks did not fully comply with BCBS239 (Basel Committee on Banking Supervision), with appropriate data controls and governance in place. Major issues across the banking industry include:
- Multiple servicing systems with nonstandardized data models.
- Mergers and portfolio acquisitions (examples include the Capital One acquisition of ING Direct, USA, and the PNC Financial Services acquisition of RBC Bank, USA) and their impact on data.
- Lack of granular behavioral data (for instance, prepayment history).
- Limited historical data availability due to system upgrades.
- Lack of historical data for new products.
Based on the complexity and materiality of balance-sheet portfolios with data limitations, banks are expected to either use external models or reuse models created for portfolios with similar risk behavior.
Methodology choices
Important considerations to select a CECL method for a portfolio include:
Figure 6. Characteristics of an effective CECL methodology
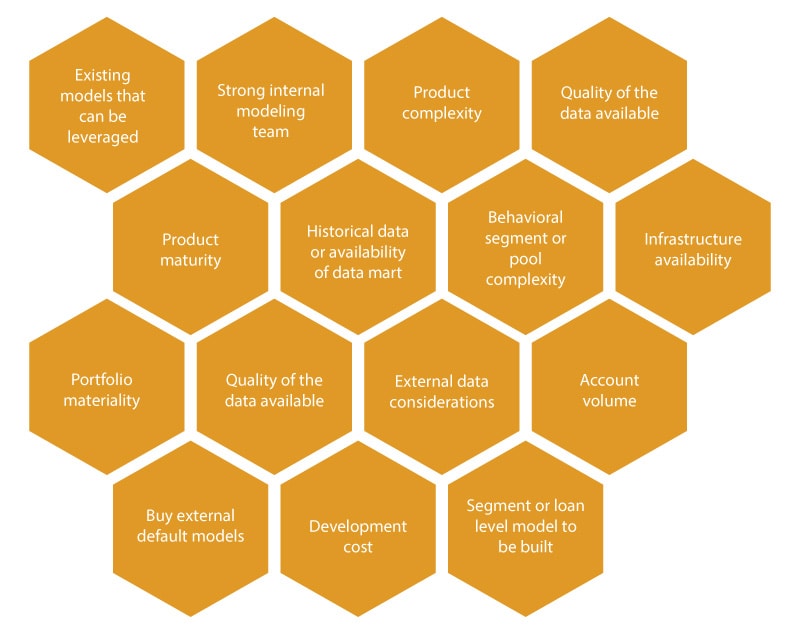
Large banks are expected to test all five methodologies (Figure 3) for some of their materially important portfolios and implement the most appropriate method. For less significant portfolios, they could use less complex methods. Midsize banks are expected to choose less complex models initially (to maintain a low risk of error) and move to complex models over time.
Skilled resources availability
CECL model development requires resources with data analytics and model-building experience. Banks generally have a small model development team and face a shortage in skill sets. They are expected to ramp up their teams with risk domain and analytics expertise over the next few months until CECL goes live and “run-the-bank” takes over.
Technology and infrastructure
Many banks have made investments in technology, with thought given to the computational nature of much of the regulatory reporting — Basel, CCAR, DFAST and internal stress tests. The execution of the CECL model on a quarterly basis is expected to incur additional load due to the volume of historical data and the computing power it demands. As a result, over the next few months, banks are expected to invest in infrastructure.
Methodology validation
Many banks undertake the model and methodology validation at the end of the process; however, validation must run parallel with the model build. This restricts data and assumption limitations and identifies approaches early. Since CECL’s concepts (including behavioral life of loan, most likely scenario, reasonable supportable forecast) are subject to interpretation, early validation helps identify and justify the right methodology. This standardizes the data gathering and model-documentation process and lays the groundwork for integration.
Achieving success beyond implementation
CECL implementation will likely result in the following challenges after the compliance date:
Impact on capital and profitability
CECL is expected to increase the banking industry’s credit-loss reserves by $50 billion initially,4 while as per the Office of the Comptroller of the Currency, credit-loss reserves could rise by 30% to 50% for banks.5
Banks must allow for additional loss reserves early to reduce the probable impact on profitability and capital. Alternatively, lending may be reduced, resulting in a liquidity crisis and credit crunch.
Rating agency Fitch said that in the short term, individual banks’ creditworthiness and their underlying loans will “remain unchanged,” while in the longer term, the ratings will depend on how each bank responds to the accounting requirements.6
While banks face significant issues in implementing CECL, they have the option to phase out the adverse effects of the proposed change over a period of three years.7
Business impact
As CECL could result in a spike in credit losses in certain accounts or portfolios, business units must relook at their lending strategy for assets where the impact is highest. For example, portfolios with longer tenor can be impacted with higher credit losses due to higher uncertainty. This will likely result in changes to the origination mix, pricing, tenor, collateral or mitigants and have an impact on banks’ overall risk appetite leading to a revision in the credit risk policy.
Access to robust, user-friendly analytical tools (including Hyperion OLAP cube, Tableau, SAS Visual Analytics) must be available to analyze and identify specific assets or pools of assets that contribute to higher credit losses. As credit losses under CECL are likely to fluctuate every quarter, business units need to explain the reason(s) for the fluctuation.
Business as usual (BAU) and automation
It is important to define a BAU process for regulatory submission under CECL, as credit-loss forecasts go through multiple levels of approvals before regulatory submission. This requires both the finance (treasury, asset/liability management) and risk teams to work together.
Robotic process automation can automate manual processes and workflows such as the following:
- Approval workflow for CECL allowance.
- Separate tool or functionality for management overlays and adjustments for better auditability.
- Data transfer between upstream and downstream systems and report generation.
Business self-service
Regular model calibration is required as additional data gets collated with changes to the internal and macroeconomic conditions. Further risk factors will likely be defined as business users access and modify parameters from a front-end and a user interface perspective, instead of depending on the technology team to make these changes.
Machine learning in model review and calibration
Model review has traditionally been a manual process. Banks are now looking to adopt machine-learning algorithms to self-learn and calibrate the models.
As data gets created, machine learning will help identify irrelevant variables or recommend adding variables as part of the model equation.
This could be based on statistical analysis on variables (P values, r squared and correlation) and recommend changes to variables used in the models. This will bring additional accuracy of credit-loss numbers instead of redeveloping the models.
Consistency in models and assumptions
CECL calculates expected loss until the estimated life of the loan by considering the future balance projections after adjusting for prepayments. Many parameters are used by different departments within the bank. It is important to bring in consistency so that the same computation logic and assumptions can be used across departments.
Realizing the promise of CECL
CECL is a fundamental shift from how banks currently calculate credit losses and involves significant efforts to comply.
It shouldn’t be viewed only as a tactical goal but as a continuous strategic journey.
Over time, banks expect to generate synergies within related regulations in sharing infrastructure, data and tools. They will also increasingly adopt (1) RPA for automating workflows and manual processes to reduce turnaround time and (2) machine-learning techniques to continuously improve loss computation methodologies.
It is important for banks to have a clear vision and roadmap for their target end state. This future ready approach combined with operational rigor will help banks mitigate risk and take full advantage of the business opportunity that CECL provides.
References
- Loan Loss Reserves, Accounting Constraints, and Bank Ownership Structure,” Pages 4 and 31, The Federal Reserve Bank of Richmond, November 2011,
- Loan Loss Reserves, Accounting Constraints, and Bank Ownership Structure,” Page 3, The Federal Reserve Bank of Richmond, November 2011,
- Current Expected Credit Losses (CECL): Benchmarking Survey,” Global Credit Data, 2019,
- UPDATE: Fitch provides potential CECL impact for banks, initial ratings outlook,” Fitch Ratings, January 24, 2019,
- Curry, Thomas J. “Remarks by Thomas J. Curry, Comptroller of the Currency, Before the AICPA Banking Conference, Washington, D.C.” September 16, 2013,
- UPDATE: Fitch provides potential CECL impact for banks, initial ratings outlook,” Fitch Ratings, January 24, 2019,
- Implementation and Transition of the Current Expected Credit Losses Methodology for Allowances and Related Adjustments to the Regulatory Capital Rule and Conforming Amendments to Other Regulations,” OCC, December 21, 2018