Insights
- With inflation on the rise, consumers have become picky about what they spend on.
- This makes it critical for CPG companies to set the right prices for their products.
- Traditional RGM models are not evolved enough to help them make the right decisions related to pricing or the selection of products to retail.
- Data-driven RGM models can help them with the insights that they need to adjust their prices in this situation, and largely predict the outcomes of their actions.
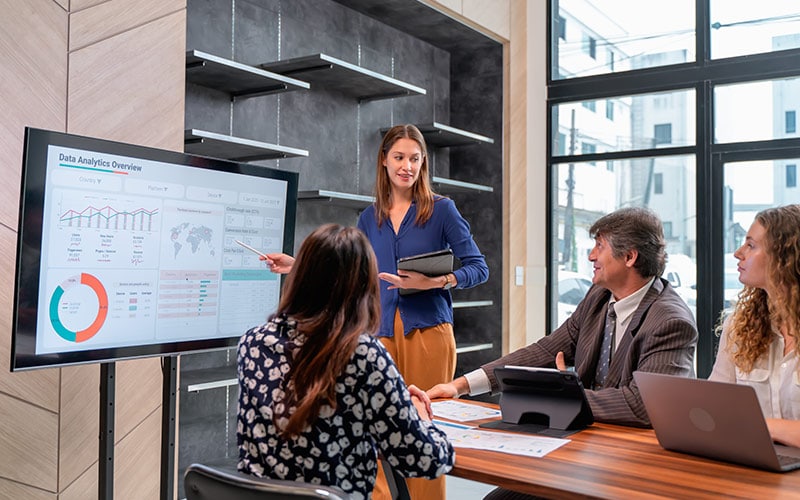
Economists are predicting recessionary conditions for 2023 amid rising inflation, declining consumer confidence, and low average household savings levels. Consumers are already shifting their spending habits and trading down from premium brands and ‘nice to have’ products to value brands and staples. It is more important than ever for consumer packaged goods (CPG) companies to embrace a data-driven revenue growth management (RGM) model. It could be the key to nailing their mix of product selection at the best price using price pack architecture, and trade promotions to grow market share and profits, and maintain strong channel partnerships.
“As the cost of living continues to rise globally, it is increasingly critical for companies to adopt a data-based approach towards pricing and price pack architecture. It might be their best shot at maximizing revenue and profits simultaneously while keeping their brand value intact in a chaotic economic landscape,” says Maksym Roshchyn, Global RGM Lead, Kraft Heinz, in ‘Grow Revenue, Not Costs: RGM Lessons from Kraft Heinz’, a virtual panel discussion by Infosys and Consumer Goods Technology (CGT).
Nestlé has had its strongest sales growth in 14 years, resulting from a 7.5% price increase. Its third-quarter organic revenue growth in 2022 was 9.3%. CEO Mark Schneider has attributed the significant organic growth to adjusting prices thoughtfully to reflect inflation.
As the cost of living continues to rise globally, it is increasingly critical for companies to adopt a data-based approach towards pricing and price pack architecture.
Another example is Unilever which hit a historic price increase of 12.5% in the third quarter of 2022, a carefully considered move, according to their spokesperson. Its net profit for the full year was up 24.9% on 2021. Clorox, which competes with Unilever in home-cleaning products, on the other hand, increased prices by 12% over 2022 and saw a volume decline of 21%, as consumers shifted to cheaper alternatives. This drives home the significance of striking the right balance in price, volume, and brand value in achieving growth successfully by leveraging brand equity.
However, hitting the sweet spot is easier said than done and takes effort, not to mention time, capital, and resources. Often, it is a toss-up between time, speed, cost, and quality.
RGM models: Traditional vs next-gen
Many companies are still arriving at their pricing and price pack architecture using traditional RGM models. These models are not equipped to consider the nuances of pricing vis-à-vis specific economic climates, and are accompanied by a host of issues:
- Incomplete data
Many organizations are limited to using historical data from internal sources, resulting in reactive strategies with short-term impact. Lack of insights into competitor pricing, sales velocity, consumer data, and retailer partner data means that decision-makers must fall back on guesswork when it comes to the big picture of market position and opportunity. - Lack of precision data
RGM leaders are often limited to using aggregate-level data, and are unable to capture granular insights for the brands, product lines, and stock keeping units (SKUs) at the customer level (that includes segments, geographies, and individual consumer segments), channel level (digital, retail, mass, club, and marketplace), and competitor level. - Operational silo
A lack of centralized governance poses an additional challenge. When individual business units and line managers take their own sources of data and use their own tools and processes, it becomes a challenge to standardize and unify data for a single source of truth. Decisions are made without knowledge transfer to groups that could benefit from it. - Manual processes
Manual data analysis, reconciliation, and reporting is time-consuming, and prone to human error.
Traditional RGM models are not equipped to consider the nuances of pricing vis-à-vis specific economic climates, and come with a host of issues.
However, revenue growth management strategies are advancing. “RGM has evolved in two directions. One is strategic RGM which connects pieces of the organization and understands the consumer and shoppers, to develop the long-term strategy. The other is precision RGM which involves driving decisions at a very granular level using digital tools. It has taken analytics to the next level, enabled by advances in AI. There are several pricing tools we are working on right now,” says Maksym Roshchyn of Kraft Heinz.
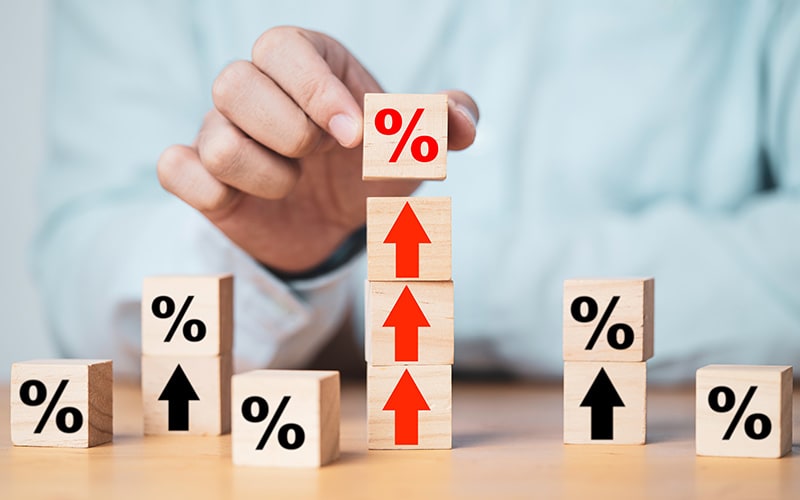
Companies are realizing the need for an analytics and data-driven revenue growth management framework and decisions. Chad Matthews, CCO, PepsiCo Beverages - North America, has expressed how data and analytics play a significant role at PepsiCo by helping it give its retail partners access to actionable insights that help with inflationary scenarios.
An AI-assisted system can help companies find the best means to hit upon data-driven insights to maximize revenue potential with predictability.
How can companies find the best means to hit upon data-driven insights to maximize revenue potential with predictability, while maintaining a good profit based on favorable cost of sales and volumes, and growing market share? The answer lies in being supported by a robust automated system.
How a data-driven model works
Companies are struggling to find ways to reduce their input costs and keep margins favorable. Increasing prices is the most common option. However, companies will have to find a way to do it while considering the effect it will have on consumers who are already reeling from inflation stresses. They must also factor in the impact on their volumes or market share.
A well-built automated system generates insights to help companies change the prices in an informed manner, while also considering those other pieces. It works by gathering data across sources, be it simple sales data from syndicated sources or other data sets such as brand equity surveys and social media data. It then applies an econometric and AI model to accurately predict consumers’ willingness to pay and their stickiness towards the brand. Based on this, it makes strategic pricing recommendations at the brand, pack, and channel level to achieve competitive-yet-sustainable growth of market share and profitability.
The benefits of a data-driven model are many:
- It prevents human error and eliminates the element of guesswork, enabling businesses to standardize their granular pricing, and forecasting processes across the organization.
- Self-learning AI – that can train itself and learn – combined with a statistical model used for predictive analysis helps simulate pricing, price pack architecture, and trade promotion scenarios, and accurately forecast the consequent increase or decrease in revenue and profit. It also predicts consumers’ willingness to pay. This empowers companies to draw up the product selection, and customize pricing for various channels.
- It provides information on tactical measures related to trade promotion. Companies can get a sense of how to organically grow in the place that they operate in by creatively pricing their product and having promotions that match or beat the competition to get a better return on investment.
Companies are beginning to recognize the importance of data analytics and technology in helping them make responsible price-related decisions. Anheuser-Busch InBev is one such case. It has stressed on the importance of not banking on just an analytics team to provide insights to a business team, but building a system that encourages collaboration between humans and machine which the business team can derive insights from before arriving at solutions to maximize revenue growth.
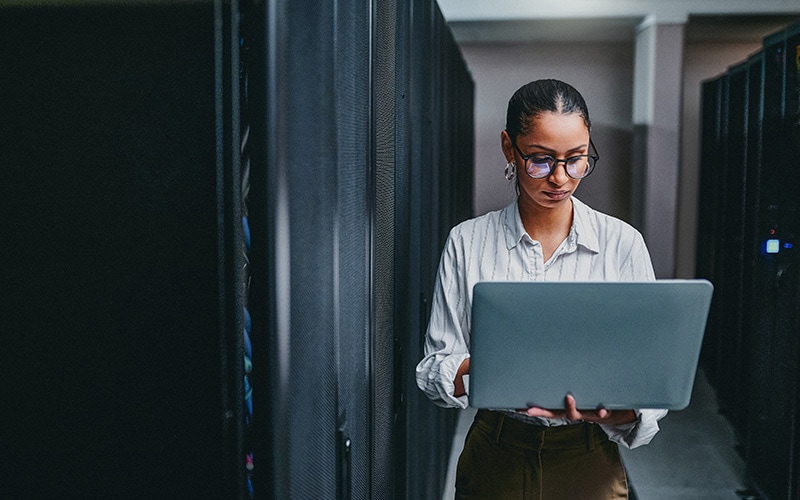
Taking the first steps
To build an econometric model that leverages data analytics to produce desired insights, the first step for companies is to identify the right sources for the data, and decide which ones are most relevant for them to solve their challenges. New and untapped data sources also make for good options.
They must assemble a robust IT and data science team to build and maintain data models. This can be outsourced if necessary. Data, including both internal metrics and external inputs, can then be used to benchmark against competitors and join the pieces of the jigsaw to create an econometric model that measures the impact of the factors in play, and create a pricing strategy.
“The first foundation for any digital capability in revenue growth management is the ability to harness the right data to drive meaningful insights,” says Rahul Ubgade, AVP, Consumer, Retail and Logistics, at Infosys. “The second layer comprises advanced algorithms, including econometric models, which are getting smarter by the day. The third layer is user experience and working out how to hide the data science from the user.”
He adds: “The actual decisions are going to be taken by the sales and marketing teams in the operating company. The RGM team must provide the tools that fit into the users’ journeys. These capabilities should be API-enabled so that the data can be communicated between different software, and the recommendations can be consumed by downstream and upstream systems.”
To build an econometric model that leverages data analytics to produce desired insights, the first step for companies is to identify the right sources for the data, and decide which ones are most relevant for them to solve their challenges.
While pricing and price pack architecture might largely remain a mystery and prone to being influenced by external factors beyond the control of companies – such as war or a climate crisis – leveraging a data and AI-driven model that weaves in various factors that affect pricing and recommends pricing and a price pack architecture based on it might be the most reliable weapon in their arsenal.