Insights
- Each industry employs use cases and nuances for autonomous systems specific to their distinct requirements.
- Standardized automation protocols foster seamless autonomy, communication, benchmarking, and economies of scale for talent and infrastructure.
- The journey to autonomy is a staged process, not a single big-bang event.
- Organizations reach their autonomy level based on business needs and evolve with developments in the industry.
- At the most mature highest level, autonomous systems are capable of independent decision-making, response, and self-training.
From textile mills in the 18th century to diverse industries today, automation has continued to evolve, sometimes through periods of disruption. Each industry employs use cases and nuances for autonomous systems specific to their distinct requirements. However, standardized automation protocols foster integration, seamless autonomy, and communication between systems. These protocols help organizations develop technology roadmaps, optimize digital infrastructure for economies of scale, and nurture necessary skills.
Standardized automation protocols foster seamless autonomy, communication, and economies of scale for talent and infrastructure.
The International Society of Automation’s ISA-95 standard developed an automated interface between enterprises and control systems, but it is specific to manufacturing. As another example, SAE's G-31 is a forum for expert information to apply digital technologies for electronic transactions in the aerospace industry. As a third case, Infosys and the other members of the G-21 committee serve an important role to create technical reports and secure technology for critical components across the product lifecycle and supply chain. These autonomy standards are evolving and need to transcend departmental boundaries and cover multiple functions across industries.
The journey to autonomy is a staged process, not a single big-bang event. At the most mature level, autonomous systems are capable of independent decision-making, response, and self-training. The Infosys Data+AI Radar 2022 research report introduced the SURE taxonomy — Sense, Understand, Respond, and Evolve — to represent the four levels of AI systems. According to the study, 63% enterprises operate at the basic Sense and Understand levels today (see Figure 1), with only 15% at the highest Evolve level. This gap highlights opportunity to generate significant benefits through autonomous and interconnected systems.
This article explores autonomy levels and capabilities, a common standard, and required resources for each stage. Organizations reach their autonomy level based on business needs and evolve with developments in the industry.
Figure 1. Infosys’ SURE taxonomy: only 15% firms possess advanced AI capabilities
Source: Infosys Tech Navigator 2023
Each industry has unique autonomy requirements. Before introducing a common standard, let's first examine existing autonomy standards for selected industries.
Smart factories for flexibility
Factories utilize a range of autonomy models. Researchers Fan Mo et al. defined the following autonomy levels for manufacturing flexibility to address evolving market dynamics:
- AL1 – The lowest autonomy level. It relies on operator actions and decisions, with disconnected systems and no centralized control.
- AL2 – Basic automation with centralized control in connected systems. It offers some context-aware features with human intervention for many tasks.
- AL3 – Features self-adaptable behaviors and predictive capabilities to meet unpredictable events. Human operators receive suggestions for optimized activities, but primary system tasks remain under human control.
- AL4 – A semi-autonomous factory with high context awareness, where human operators collaborate with the system to define boundaries and action.
- AL5 – A fully autonomous factory, empowered by advanced self-learning, skillful at choosing optimal paths to achieve common goals and adapting to unforeseen inputs.
The Singapore Economic Development Board’s Smart Industry Readiness Index establishes a standard for factory autonomy levels. Six maturity bands, from 0 (lowest) to 6 (highest), evaluate factories across 16 dimensions. Further, Infosys codeveloped a maturity index with the Acatech consortium and Aachen University, to provide a factory autonomy framework.
Honda Car India embraced smart manufacturing in its second Indian factory through digital technologies and real-time visibility. In collaboration with Infosys, the team implemented an IoT-enabled quality control system for real-time defect identification and high-quality vehicle delivery. Honda achieved a key milestone in manufacturing through end-to-end part traceability.
Robots for material handling and precision surgery
Robots are a common manifestation of autonomous systems to handle materials. Field robots broadly fall into four categories: collaboration, inventory transportation, scalable storage picking, and automatically guided vehicles. Borrowing from the SAE International’s taxonomy for car autonomy, there are six defined autonomy levels for field robots:
- Level 0 – Full manual tele-operation
- Level 1 – Robot within line of sight (hands off)
- Level 2 – Operator on site or nearby (eyes off)
- Level 3 – One operator oversees many robots (mind off)
- Level 4 – Supervisor not on site (monitoring off)
- Level 5 – Robots adapt and improve execution (development off)
A large logistics company sought to integrate autonomous robots into its warehouse floor to improve efficiency, accuracy, and speed. However, robotics and automation in traditional warehouse environments require careful consideration to maintain trust between workers, management, and the new autonomous systems. Prior to a robot launch, Infosys subsidiary Kaleidoscope partnered with the company to develop a safe testing and evaluation method for the autonomous robotic design. They created a portable VR-based digital twin of the warehouse and the robot, allowing associates to transition from their actual workstations to a digital twin within the same building. The logistics company safely tested designs, gathered user insights, and reduced effort by 70% compared to traditional validation methods. This VR platform became a hardware and software development roadmap and a powerful demonstration tool.
In healthcare robotics, Eduard Fosch-Villaronga, Hadassah Drukarch et al. from Leiden University, Netherlands, define six autonomy levels:
- AL0 – No autonomy
- AL1 – Robot assistance during a surgery
- AL2 – Partial autonomy for specific surgical tasks under human supervision
- AL3 – Conditional autonomy to devise and execute surgical strategies under human supervision
- AL4 – High autonomy with human surgeon oversight and intervention when required
- AL5 – Full autonomy with surgeon oversight
A recent robotic surgery application involved a remote procedure, with 5G communication for real-time monitoring and feedback. Doctors remotely control a laser and robotic gripper while monitoring the procedure through a video feed.
Robots require millions of data points to synthesize, learn, and make informed decisions. Medical device companies face a significant challenge due to the absence of real-life data. Relevant data ranges from measuring actual tissue thickness during surgery, identifying critical structures through visualization, and understanding surgeons’ natural movements and reactions in various surgical scenarios. Traditional laparoscopic tools lack appropriate sensors and mechanisms to collect such data.
A medical device company sought to transform the surgical suite. Kaleidoscope assisted its design and engineering teams to develop a first-generation surgical robot and advanced laparoscopic tool, as the foundation for a fully autonomous surgical robot. Robotic-assisted surgery requires careful consideration of haptics and surgeon console feedback. Kaleidoscope teams conducted multiple rounds of user testing on the digital user interface, surgeon console haptics, and surgeon response to inform future design decisions.
Driverless cars for safety
SAE International defines autonomy levels in the automotive industry in SAE J3016 as follows:
- Level 0 – No autonomy
- Level 1 – Driver assistance features
- Level 2 – Partially automated features for acceleration and speed, but driver always engaged
- Level 3 – Conditional automation without constant driver monitoring, but the driver must be available when needed
- Level 4 – High automation for all features under certain conditions, with driver intervention when required
- Level 5 – Full automation for all features under all conditions
Google’s Waymo and Amazon’s Zoox operate Level 4 autonomous taxis in Phoenix, Arizona, and California, US (GM’s Cruise was also a significant player, but at the time of writing halted operations due to safety concerns). These taxis have no driver at the steering wheel, but a human monitor the situation and take control when required.
Infosys, in collaboration with Maini Group, built an autonomous buggy by retrofitting an existing golf cart at their Bangalore campus. The buggy utilized a patented drive-by-wire technology comprised of auto-braking and navigation features equipped with advanced LiDAR and vision technologies. The cart has an on-board computer with AI and a deep learning engine to detect objects, lanes, and curbs for navigation. The buggy will operate in controlled campus settings like industrial, educational, airport, and amusement park environments. This technology is also used to build autonomous tow trucks and mobile robots to move material autonomously in a shopfloor. Currently, all these three vehicles − autonomous golf cart, tow truck and mobile robot are commercialized.
Automation in mobility is not limited to cars. Rio Tinto Group harnesses giant autonomous trains, the world's largest and longest robots, to transport iron ore during trials of battery-powered locomotives in Australia. Electrifying these 2.5-kilometer trains will cut diesel use and are expected to support 50% reduction in greenhouse gas emissions by 2030.
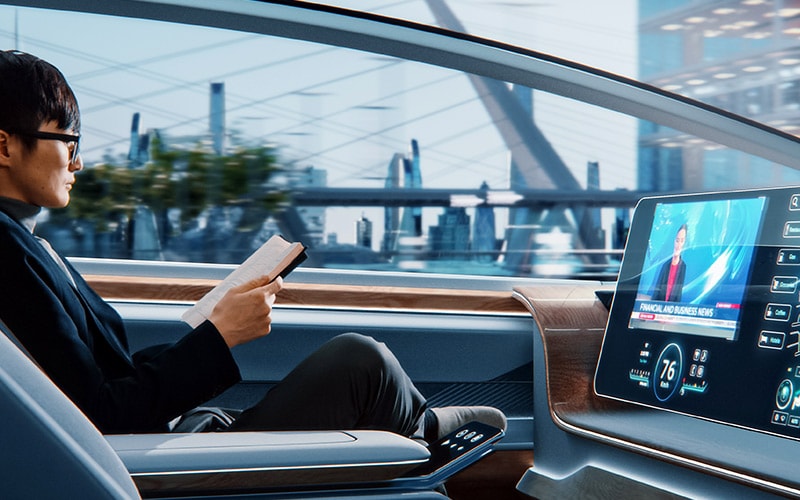
Pilotless flying for safety
The following are the autonomy levels in a pilotless aerial vehicle:
- 1 – Low autonomy; pilot in control for object detection and warnings
- 2 – Partial autonomy; pilot in control
- 3 – Conditional autonomy; pilot acts as a backup to detect objects and avoid situations
- 4 – High autonomy; pilot remotely monitors to detect objects and navigate
- 5 – Full autonomy; pilot sets objectives without the need for continuous monitoring
DragonFly, Airbus’ autonomous technology initiative, seeks safe and efficient flights. Its three-fold initiative during emergencies includes pilot assistance, flight path capability, and automatic landing. The plane autonomously communicates with ground air traffic controllers, descends, and lands with considering other aircraft, terrain, and weather conditions. Like a dragonfly, the technology uses onboard sensors to identify ground landmarks to draw its boundaries and simultaneously utilizes other flight information at the same time. DragonFly embodies biomimicry (biologically inspired engineering), similar to Infosys’ Live Enterprise framework.
Normalized automation levels
Industry-specific autonomy characteristics matter. However, standard autonomy levels facilitate interoperability and benchmarking, leverage lessons from past implementations, support skill development and infrastructure to achieve economies of scale, and enable governance mechanisms.
Figure 2 depicts normalized levels across two dimensions: scale of operation (from components and subsystem level to the overall ecosystem), and technology complexity (from simple data acquisition sensors to advanced control systems).
Figure 2. Autonomous system evolution model
Source: Infosys
Autonomous technology enablers for each autonomy level:
- AL5 – Full automation, where the system performs all functions under all conditions
- AL4 – High automation, where the system performs all functions under certain conditions
- AL3 – Controlled automation with human ready to take control
- AL2 – Partial automation with partial human attention
- AL1 – Fixed automation with full human attention
- AL0 – Manually intensive
Generic levels of automation normalizing the above industry-specific standards:
Table 1. Automation levels, their definition, and characteristics
Source: Infosys
Automation timelines
Advanced autonomy isn't a single step, but a journey of gradual maturity ascent. We recommend a three-horizon roadmap (Figure 3):
- Horizon 1 (H1 or the past one year): Partial automation for individual tasks and processes. The focus is on productivity, efficiency, quality, and cost reduction, to free humans from repetitive tasks.
- H2 (past one year to the next year): Controlled automation with technology innovation, speed, and scale. This phase integrates sensors for motion and gesture detection and control, spatial sensing, obstacle detection, and path planning. Examples include autonomous guided vehicles along specific paths, tow trucks, and robotic systems.
- H3 (three to five years): Fully autonomous systems driven by business needs, featuring connected, intelligent systems with AI-led decision-making, both at the edge and centralized in the cloud. Examples include SAE Level 4 or 5 autonomous vehicles, swarm robots, and truck platooning.
Figure 3. Autonomy time horizons (H1 to H3) and their characteristics
Source: Infosys
Recommendations
As common standards for autonomous systems evolve, four guiding principles for enterprise autonomy implementation have emerged:
- Cross-domain system interoperability: Industry-specific autonomy standards are essential, but system interoperability across domains (mobility, healthcare, energy, facilities) from the outset prevents costly rework later.
- Maturity index for the road map: Select a recognized maturity index framework to mitigate associated risks. These indices are distilled from proven approaches and incorporate valuable lessons. They mitigate risks and facilitate benchmarking with peer organizations, within and beyond industries.
- Balanced central and edge computing for real-time decisions: Central systems like cloud computing may introduce delays, while edge computing on devices has storage and processing limitations. Build intensive models centrally on the cloud, train them, and deploy tested models on the edge with ongoing updates.
- Participation in standards bodies: Enterprises should participate in standards committees of industry bodies such as SAE International to ensure that their voice is heard and for active contribution and advancement of autonomy.
Industry-specific autonomy standards are essential, but system interoperability across domains prevents costly rework later.
As business leaders adopt an AI-first approach, they should view enterprises as a system-of-systems. Each business unit, department, or function is a system with distinct objectives and metrics. When automation and artificial intelligence are implemented, common standards are needed to achieve a global optimum beyond short-term, local benefits at the unit or function level.