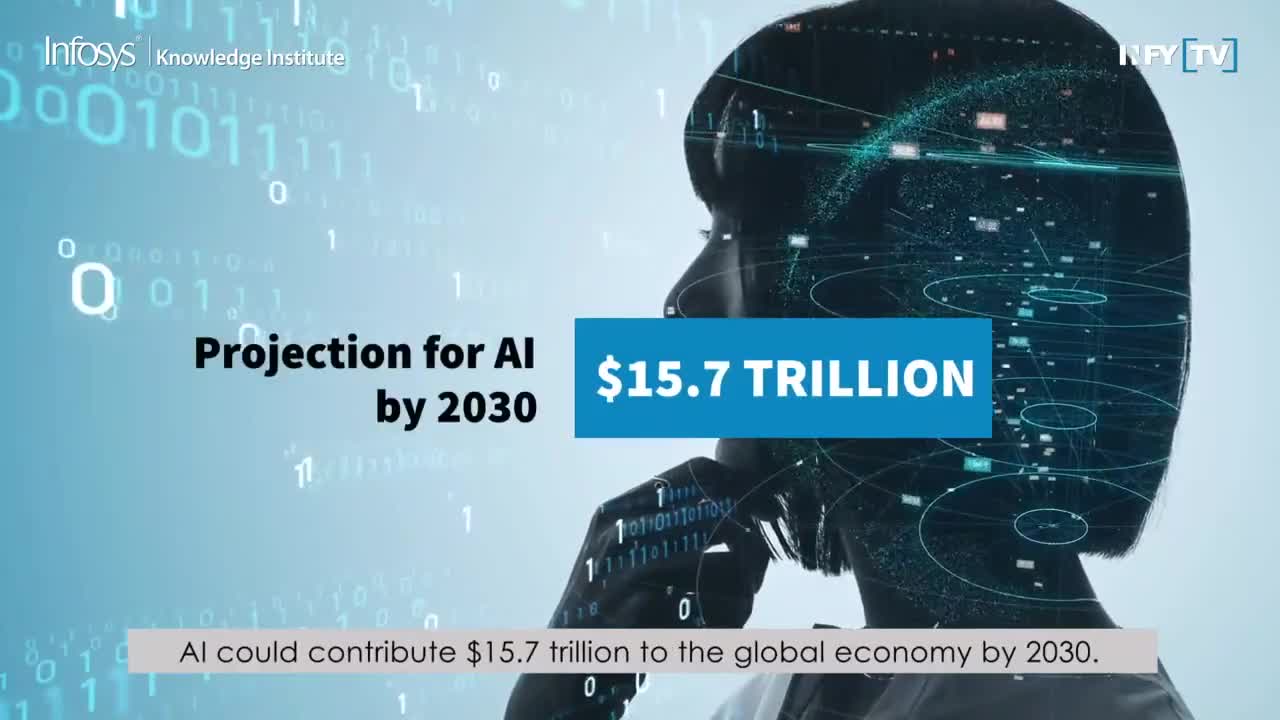
Bias in AI has a real impact on business growth. Here’s why it needs to be tackled.
As organizations across the globe realize the value of artificial intelligence, there is also a growing need to acknowledge the roadblocks and make efforts to remedy them, to maximize the impact of the technology. AI experts share their thoughts.
Artificial Intelligence (AI) can change how companies operate, and over the past few years, the technology has gained significant traction across industries.
Organizations are finding ways to incorporate AI to automate processes, make them more efficient, focus on innovation, and drive significant benefits for business.
According to a PwC report, Artificial Intelligence could contribute close to $15.7 trillion to the global economy and could be a massive opportunity.
In essence, there is a huge opportunity.
“AI has massive potential and is projected to be the biggest economic opportunity of our lifetime,” says Genevieve Smith, Associate Director - Center for Equity, Gender & Leadership - University of California, Berkeley, Haas School of Business.
Source: PwC/ Mitigating Bias in AI Playbook
She notes, however, that as business leaders will be dealing with AI they need to equip themselves to be able to deal with the challenges it could pose.
Bias in AI – Where It Originates
One might wonder what the challenge could be in using an advanced form of technology such as artificial intelligence.
There are quite a few, it appears.
A loan or mortgage being refused because someone belongs to a certain community. Someone might be rejected from a job because they belong to a particular gender.
These, and many such real-life instances point towards the biases in AI, which can have an impact on how the society operates.
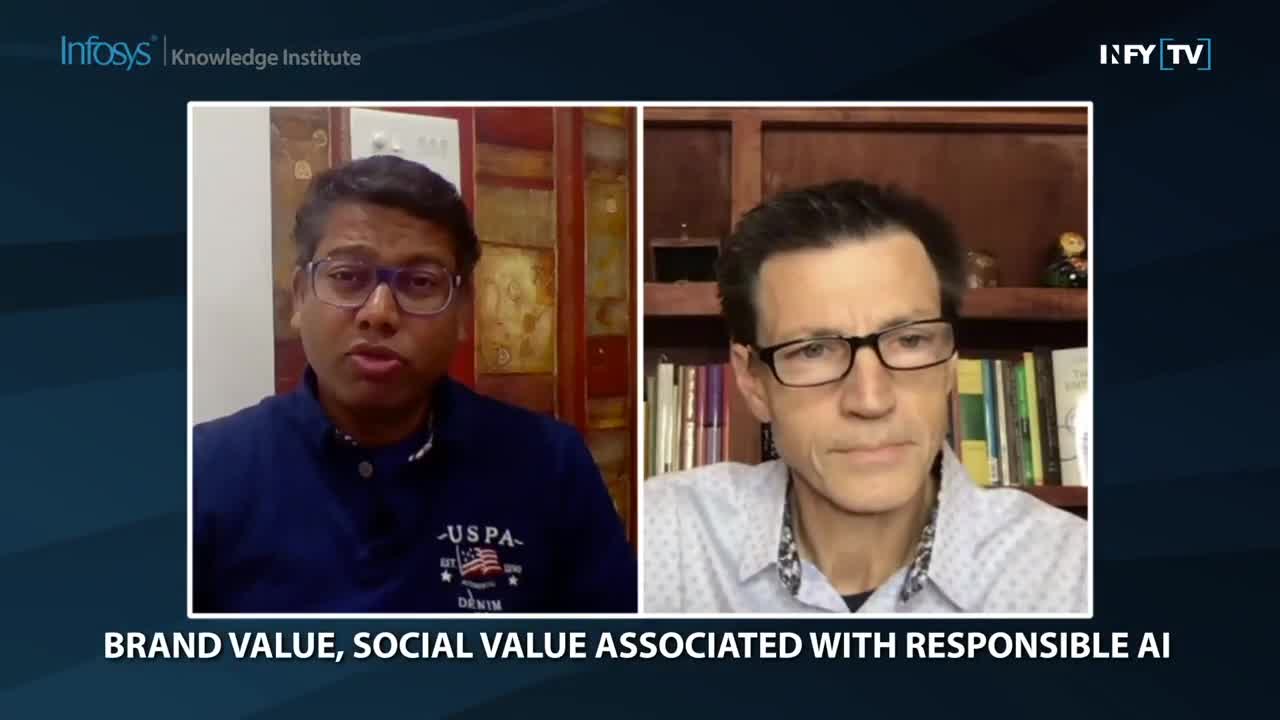
These biases, say experts, credit their origins back to humans.
“We, as humans, experience biases all the time. In fact, our brains are wired to be biased,” says Genevieve, defining biases as cognitive shortcuts that result in judgments that can lead to discriminatory practices.
“If you look at bias, the definition is something around… a tendency, or inclination, or maybe a prejudice, towards, or against, something or someone,” she says.
“We as humans experience biases all the time. In fact, our brains are wired to be biased,” says Genevieve Smith.
“We as humans experience biases all the time. In fact, our brains are wired to be biased,” says Genevieve Smith. Tweet
These human biases have a direct correlation with the ones that crop up in the implementation of AI systems.
To find answers, Genevieve and her colleagues at the Berkeley Haas Center for Equity, Gender & Leadership, delved deep and came up with their research – Mitigating Bias in Artificial Intelligence: An Equity Fluent Leadership Playbook. Read more
The playbook notes how bias creeps in into AI systems, and how organizations should be mindful of the impacts, and possible solutions to derive maximum benefits from the technology.
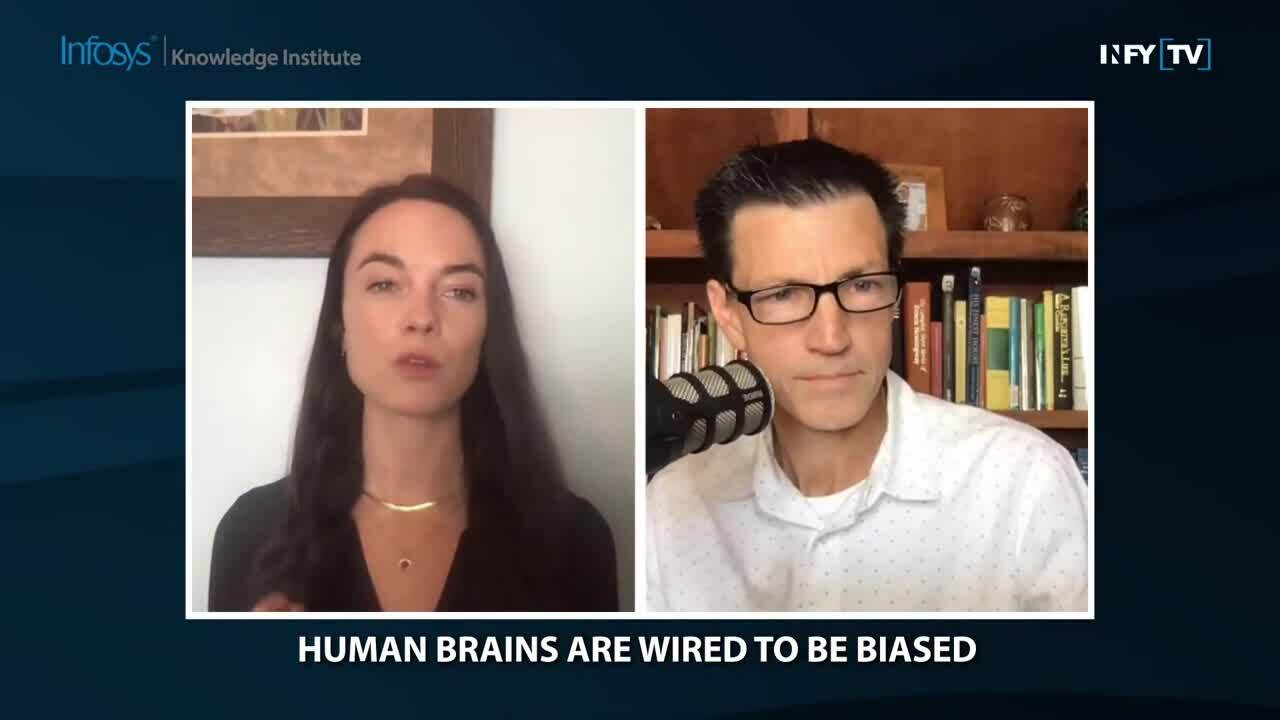
Focus on Responsible, or Ethical AI plays an important role here.
“The goal of AI ethics is to explain how and why technology makes certain often difficult decisions,” says Jeff Kavanaugh, Global Head of Infosys Knowledge Institute.
“Responsible AI means being fair, being responsible, building systems that are explainable, interpretable, and systems that can produce results in a repeatable, consistent manner,” says Sudhanshu Hate, Senior Principal Technology Architect, Infosys.
Keeping Data at the Center
It is critical to understand and appreciate that AI systems are automating judgments, and they learn from data to make decisions and predictions.
Not only does the AI system learn from human choices, and the perspectives and knowledge of those who develop AI systems, but it also learns from how it is operated and used.
“We live in a world with discrimination, inequity, and bias. And this can be reflected and baked into AI systems. So, it really matters who's developing these AI systems and where they're being developed,” says Genevieve.
“We as humans experience biases all the time. In fact, our brains are wired to be biased,” says Genevieve Smith. Tweet
The use of AI in predictions and decision-making can reduce human subjectivity, she says, but it can also embed biases resulting in inaccurate, or discriminatory predictions and outputs for certain subsets of the population.
The solution lies in taking care of data at every step, starting from the selection of the problem, says Sudhanshu.
“The problem that we select should be bias free. And then when you get into data set creation, for training your artificial intelligence system, the data for the different classes should be well balanced,” he says adding that the data should not be in favour of any one class.
“And the third is selection of the right algorithm.”
IKI’s Jeff advises organizations to invest as much as possible in data and in more logic.
“The closer you get to logic and facts, the more that bias tends to recede,” notes Jeff.
“Human involvement is very important, because bias can get introduced at any stage,” notes Sudhanshu Hate. Tweet
Keeping the Human in the Loop
Experts suggest that at having a human in the loop is critical to ensure that bias do not creep into AI systems.
“Human involvement is very important, because bias can get introduced at any stage,” notes Sudhanshu, adding that a strong human involvement is required at the stages across - selection of a problem, creation of data set, collection of the data, and audit of that data.
Jeff notes that even if the process is under control, there always needs to be a human governance or oversight.
The sources of the data, the condition in which the data would be used, and in what condition the system might not work is also important, according to Sudhanshu. And finally, the results generated by the AI system should also be verified by humans.
“So that the system is behaving as per the design principles that have been laid out,” he says adding that the learnings should then be fed back to the system.
A 2019 DataRobot report found that 42% of organizations currently using / producing AI systems are
“very” to “extremely” concerned about the reputational damage that media coverage of biased AI can
cause.
Source: PwC/Bias in AI Playbook
Can Technology Help Reduce Bias?
“Technology plays an important role,” says Sudhanshu, adding that it should be ensured that the data to be used to train the model should be well represented, in which technology can play a role.
“There are techniques to look at the representation of data in a data set,” says Sudhanshu. He suggests that data quality, and whether all classes are represented are also important parameters, while ensuring the explainability of the AI model in the AI life cycle.
“There are frameworks that help you explain the outputs of a model. And that is very, very useful in justifying the results that are produced by a system,” he says.
XAI must be used throughout the AI life cycle
Source: ICETS
Bias and its Impact on Organizations
While organizations across the world are recognizing the power of AI, it is important for them to first acknowledge the presence of bias in the systems simply because the resulting costs are enormous.
Sudhanshu suggests that there is a lot of brand value and social value associated with the technology, and there are a few instances when AI implementations have missed the mark, resulting in a negative impact for brands.
Genevieve agrees.
“Biased AI systems can result in very large reputational costs for companies that produce and or use them,” says Genevieve, while adding that there could also be implications for undermining the AI systems resulting in having to do damage control, losing consumers, or even future market opportunities.
“Companies are acknowledging there's huge reputational harm and risks that can come from these systems,” says Genevieve Smith. Tweet
Genevieve mentions that Microsoft had flagged reputational harm or liability, due to biased AI systems, in a report to the US Securities and Exchange Commission.
“So, there's something that's showing up. Companies are acknowledging there's huge reputational harm and risks that can come from these systems,” she says.
And, it’s not all external pressure, notes Genevieve, speaking of an increasing employee demand for ethical practices.
“AI has immense implications for internal conflicts or unwanted media attention, that can damage corporate reputation and brand value,” says Genevieve, adding, “On the other hand, by having more ethical approaches, algorithms, etc., (organizations) can attract and retain better talent.”
“(Organizations) have to understand the landscape and the complexity of it. So, the awareness of ethical AI should be there with all stakeholders,” says Sudhanshu Hate. Tweet
Tackling Bias at Every Step
Organizations need to make a conscious effort to address the biases ranging from the point of origin to cases where bias creeps in at a later stage.
“(Organizations) have to understand the landscape and the complexity of it. So, the awareness of ethical AI should be there with all stakeholders,” says Sudhanshu.
Genevieve suggests that business leaders ought to cultivate a sense of shared responsibility and think about what the incentive structures and power dynamics are in their organizations.
While ethical considerations (which can require slowing down) and being first to market may be in tension, it is important for business leaders to prioritize and tackle issues such as bias in AI, which have important business implications.
By tackling bias in AI systems throughout the development and management of these systems, businesses can...
- Mitigate risk
- Maintain strong brand reputation
- Have a superior value proposition
- Stay ahead of forthcoming legislation
- Be a competitive leader in the fast-paced industry
Source: Bias in AI Playbook
“[Ethical considerations and being first to market] can really be at odds. Business leaders have an important role to play in updating incentive structures so that it's more encouraged to take that pause, to consider those ethical implications,” says Genevieve, adding:
“Business leaders also have the opportunity to set responsible and ethical AI principles and approaches that can decide what it means to responsibly deploy these technologies and think about what “ethical” means.”
Genevieve also mentions the importance of being in alignment with new tech governance to be able to better navigate an increasingly challenging regulatory landscape, while maintaining alignment with their own corporate values.
“Because this is very evolving field, it's very important that businesses have a very good perspective of what is happening in the regulatory world,” adds Sudhanshu.
“It really matters who's developing these AI systems and where they're being developed,” says Genevieve. Tweet
Questions such as – What are various regulation in in their industry? And, what are the things that they should be avoiding? – should be tackled so that they don't get onto the wrong side of the of the regulations, says Sudhanshu.
“These are all really critical for corporate governance to consider, and the Board of Directors has a role to play,” says Genevieve mentioning that establishing AI ethics boards and ethics, and ensuring that responsible AI principles and codes are all a part of the corporate agenda is essential.
Beyond these actions to mitigate bias in AI systems at the corporate governance level, there are also important actions for businesses take to support diverse, multi-disciplinary teams and enable responsible AI models (see Graphic 4).
Teams
Enable diverse and multi-disciplinary teams working on algorithms and AI systems.
Promote a culture of ethics and responsibility related to AI.
AI Model
Practice responsible dataset development.
Establish policies and practices that enable responsible algorithm development.
Corporate governance & leadership
Establish corporate governance for responsible AI and end-to-end internal policies to mitigate bias.
Engage corporate social responsibility (CSR) to advance responsible / ethical AI and larger systems change.
Use your voice and influence to advance industry change and regulations for responsible AI.
Source: Bias in AI Playbook
Sudhanshu also emphasizes that there should be consistent, coherent principles that everybody should be able to abide by.
“There are other things like usage of right technology, usage of right frameworks, so that there is no scope of bias creeping in at any stage of the AI lifecycle,” he notes, adding that with appropriate steps, even if bias does creep in, it should get caught and be eliminated.
“In the knowledge Institute research, firms that concentrate on AI governance outperform the rest of the firm's by considerable margin,” adds Jeff.
Genevieve, however, suggests that businesses can also build in the right processes and steps from the get-go.
“Instead of retro actively employing them… building in these processes from the beginning can really be helpful and transformative for business,” she says.
Organizations have the opportunity to tackle biases that can come up.
“Businesses that get this right will get ahead,” she says.